Gap: Quantifying The Generative Adversarial Set And Class Feature Applicability Of Deep Neural Networks
2020 25TH INTERNATIONAL CONFERENCE ON PATTERN RECOGNITION (ICPR)(2020)
摘要
Recent work in deep neural networks has sought to characterize the nature in which a network learns features and how applicable learnt features are to various problem sets. Deep neural network applicability can be split into three sub-problems; set applicability, class applicability, and instance applicability. In this work we seek to quantify the applicability of features learned during adversarial training, focusing specifically on set and class applicability. We apply techniques for measuring applicability to both generators and discriminators trained on various data sets to quantify applicability.
更多查看译文
关键词
generative adversarial set,class feature applicability,deep neural networks,applicable learnt features,problem sets,neural network applicability,set applicability,class applicability,instance applicability,adversarial training,data sets
AI 理解论文
溯源树
样例
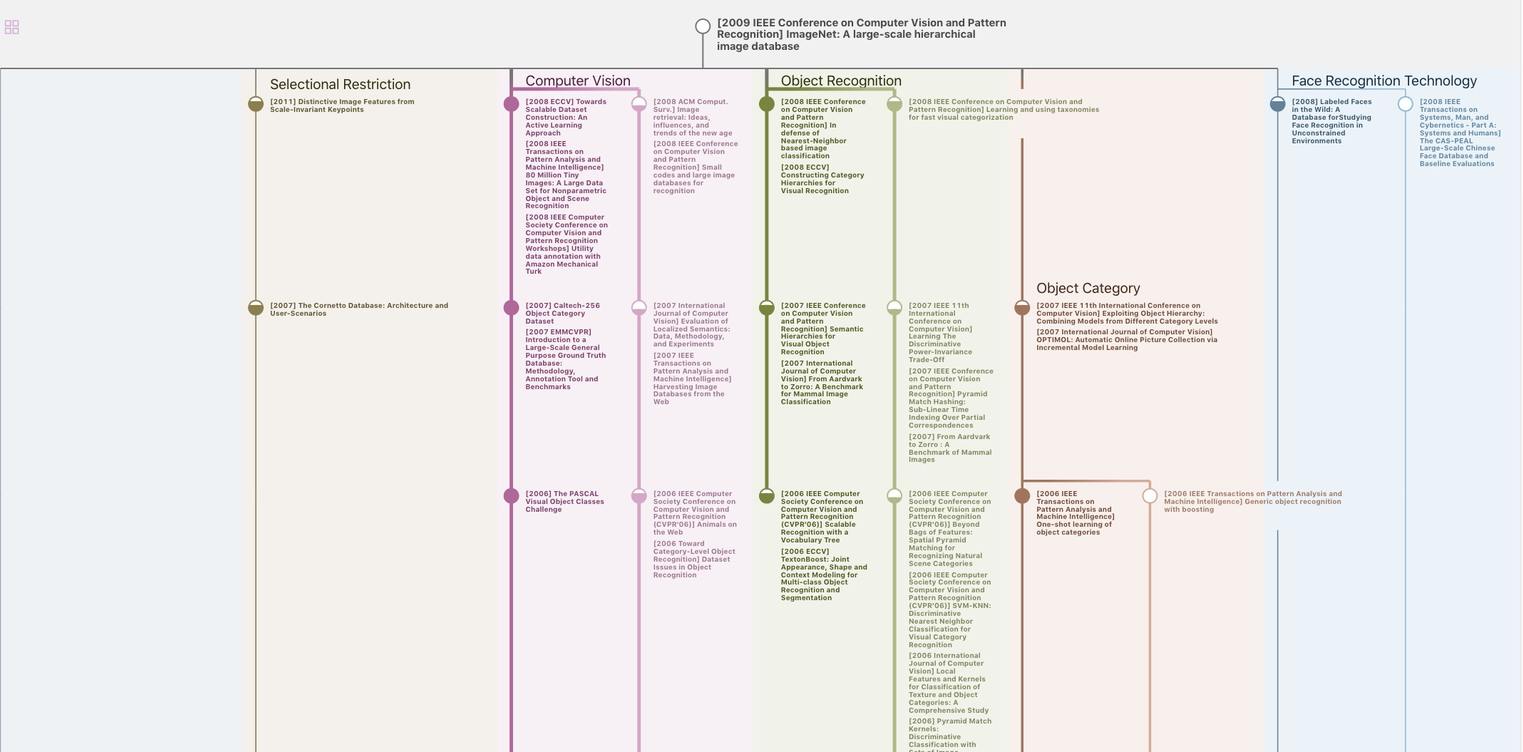
生成溯源树,研究论文发展脉络
Chat Paper
正在生成论文摘要