Dependently Coupled Principal Component Analysis for Bivariate Inversion Problems
2020 25TH INTERNATIONAL CONFERENCE ON PATTERN RECOGNITION (ICPR)(2021)
摘要
Principal Component Analysis (PCA) is a widely used technique for dimensionality reduction in various problem domains, including data compression, image processing, visualization, exploratory data analysis, pattern recognition, time-series prediction, and machine learning. Often, data is presented in a correlated paired manner such that there exist observable and correlated unobservable measurements. Unfortunately, traditional PCA techniques generally fail to optimally capture the leverageable correlations between such paired data as it does not yield a maximally correlated basis between the observable and unobservable counterparts. This instead is the objective of Canonical Correlation Analysis (and the more general Partial Least Squares methods); however, such techniques are still symmetric in maximizing correlation (covariance for PLSR) over all choices of the basis for both datasets without differentiating between observable and unobservable variables (except for the regression phase of PLSR). Further, these methods deviate from PCA's formulation objective to minimize approximation error, seeking instead to maximize correlation or covariance. While these are sensible optimization objectives, they are not equivalent to error minimization. We therefore introduce a new method of leveraging PCA between paired datasets in a dependently coupled manner, which is optimal with respect to approximation error during training. We generate a dependently coupled paired basis for which we relax orthogonality constraints in decomposing unreliable unobservable measurements. In doing so, this allows us to optimally capture the variations of the observable data while conditionally minimizing the expected prediction error for the unobservable component. We show preliminary results that demonstrate improved learning of our proposed method compared to that of traditional techniques.
更多查看译文
关键词
Principal Component Analysis,Canonical Correlation Analysis,Partial Least Squares,Segmentation,Shape Analysis
AI 理解论文
溯源树
样例
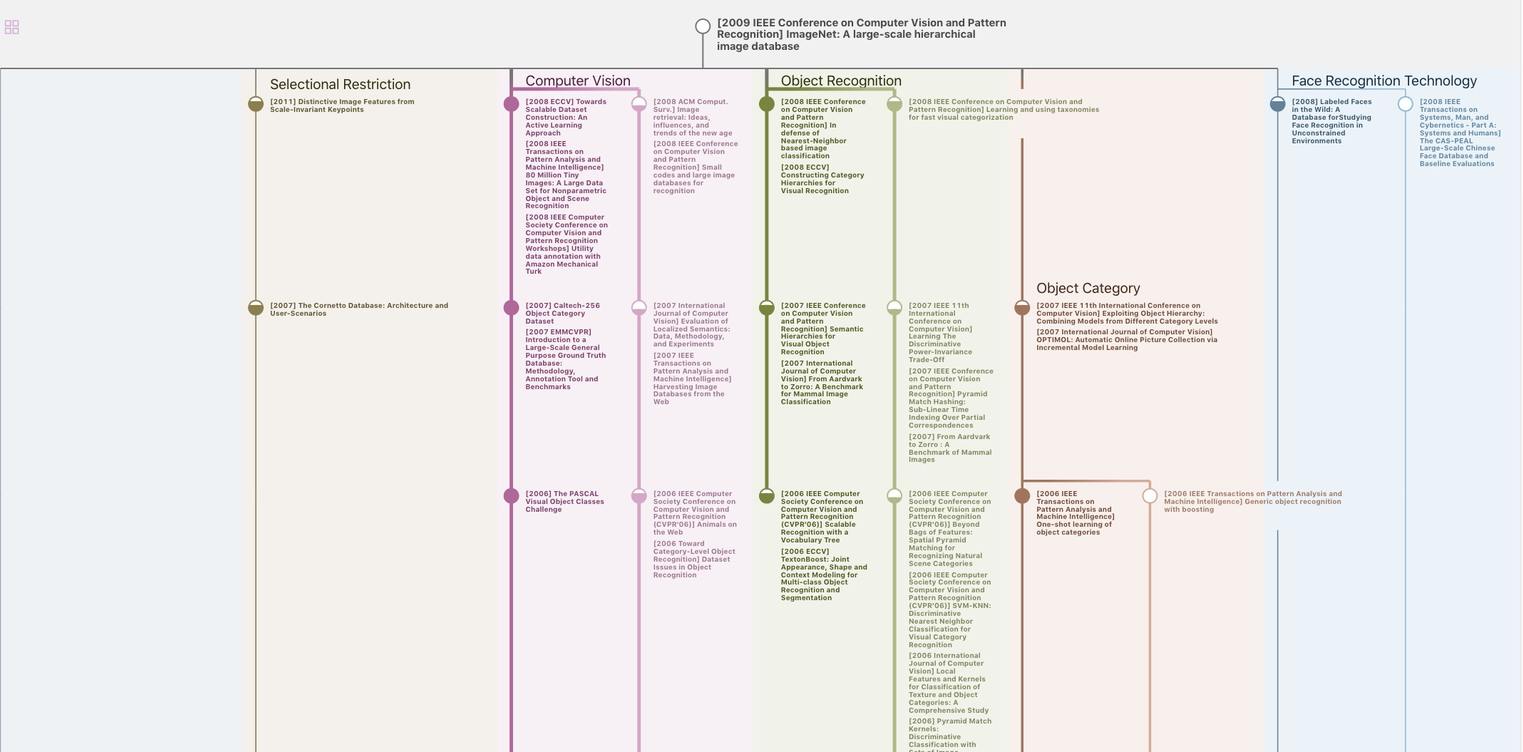
生成溯源树,研究论文发展脉络
Chat Paper
正在生成论文摘要