Enhancing Depth Quality Of Stereo Vision Using Deep Learning-Based Prior Information Of The Driving Environment
2020 25TH INTERNATIONAL CONFERENCE ON PATTERN RECOGNITION (ICPR)(2020)
摘要
Generation of high density depth values of the driving environment is indispensable for autonomous driving. Stereo vision is one of the practical and effective methods to generate these depth values. However, the accuracy of the stereo vision is limited by texture-less regions, such as sky and road areas, and repeated patterns in the image. To overcome these problems, we propose to enhance the stereo generated depth by incorporating prior information of the driving environment. Prior information, generated by deep learning-based U-Net model, is utilized in a novel post-processing mathematical framework to refine the stereo generated depth. The proposed mathematical framework is formulated as an optimization problem, which refines the errors due to texture-less regions and repeated patterns. Owing to its mathematical formulation, the post-processing framework is not a black-box and is explainable, and can be readily utilized for depth maps generated by any stereo vision algorithm. The proposed framework is qualitatively validated on the acquired dataset and KITTI dataset. The results obtained show that the proposed framework improves the stereo depth generation accuracy.
更多查看译文
关键词
texture-less regions,road areas,driving environment,deep learning,depth maps,stereo depth generation accuracy,depth quality,high density depth values,autonomous driving,stereo vision,post-processing mathematical framework,U-Net model,optimization,mathematical formulation,KITTI dataset
AI 理解论文
溯源树
样例
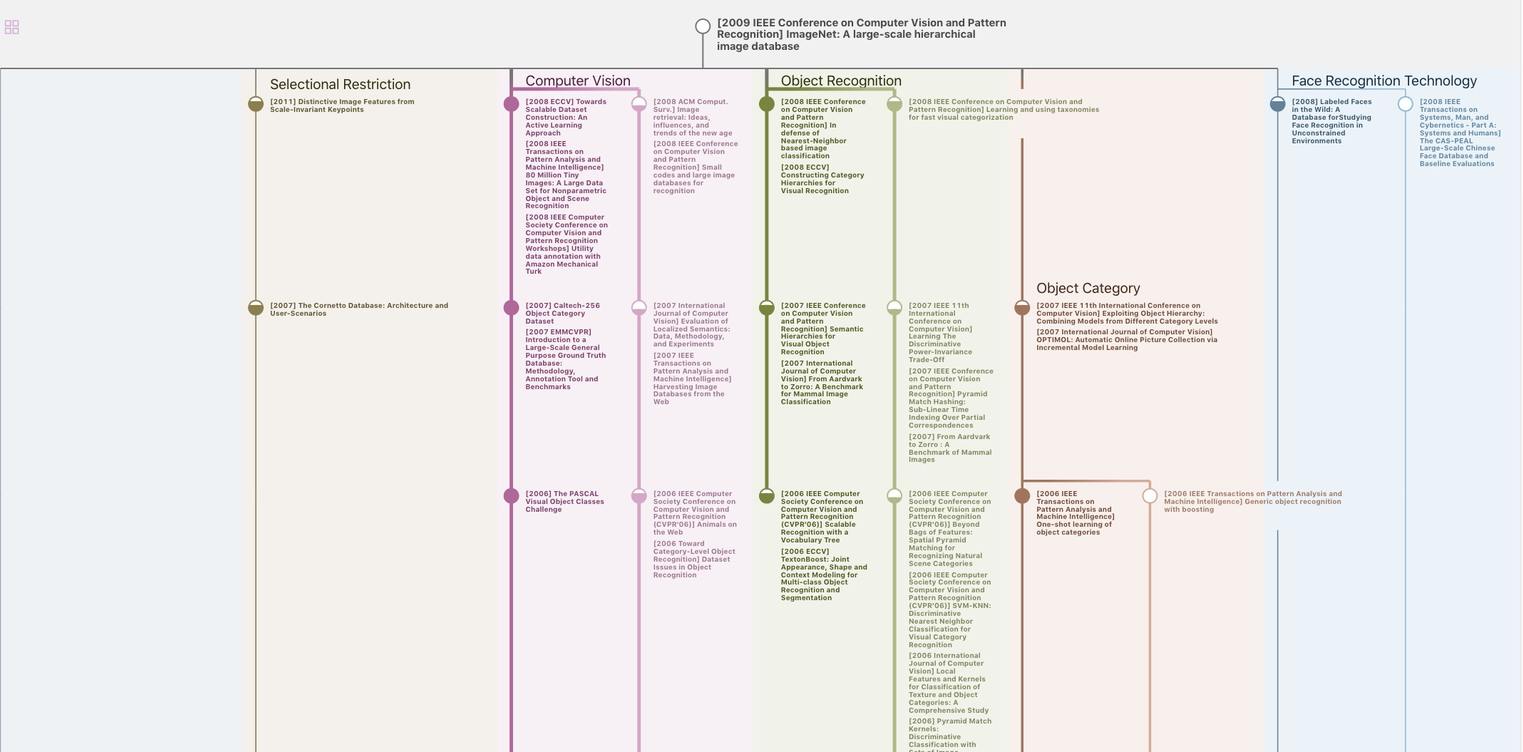
生成溯源树,研究论文发展脉络
Chat Paper
正在生成论文摘要