Tensorized Feature Spaces For Feature Explosion
2020 25TH INTERNATIONAL CONFERENCE ON PATTERN RECOGNITION (ICPR)(2020)
摘要
In this paper(1), we present a novel framework that uses tensor factorization to generate richer feature spaces for pixel classification in hyperspectral images. In particular, we assess the performance of different tensor rank decomposition methods as compared to the traditional kernel-based approaches for the hyperspectral image classification problem. We propose ORION , which takes as input a hyperspectral image tensor and a rank and outputs an enhanced feature space from the factor matrices of the decomposed tensor. Our method is a feature explosion technique that inherently maps low dimensional input space in R-K to high dimensional space in R-R , where R >> K, say in the order of 1000x, like a kernel. We show how the proposed method exploits the multi-linear structure of hyperspectral three dimensional tensor. We demonstrate the effectiveness of our method with experiments on three publicly available hyperspectral datasets with labeled pixels and compare their classification performance against traditional linear and non-linear supervised learning methods such as SVM with Linear, Polynomial, RBF kernels, and the Multi-Layer Perceptron model. Finally, we explore the relationship between the rank of the tensor decomposition and the classification accuracy using several hyperspectral datasets with ground truth.
更多查看译文
关键词
Tensor, Tensor Decomposition, Hyperspectral Imaging
AI 理解论文
溯源树
样例
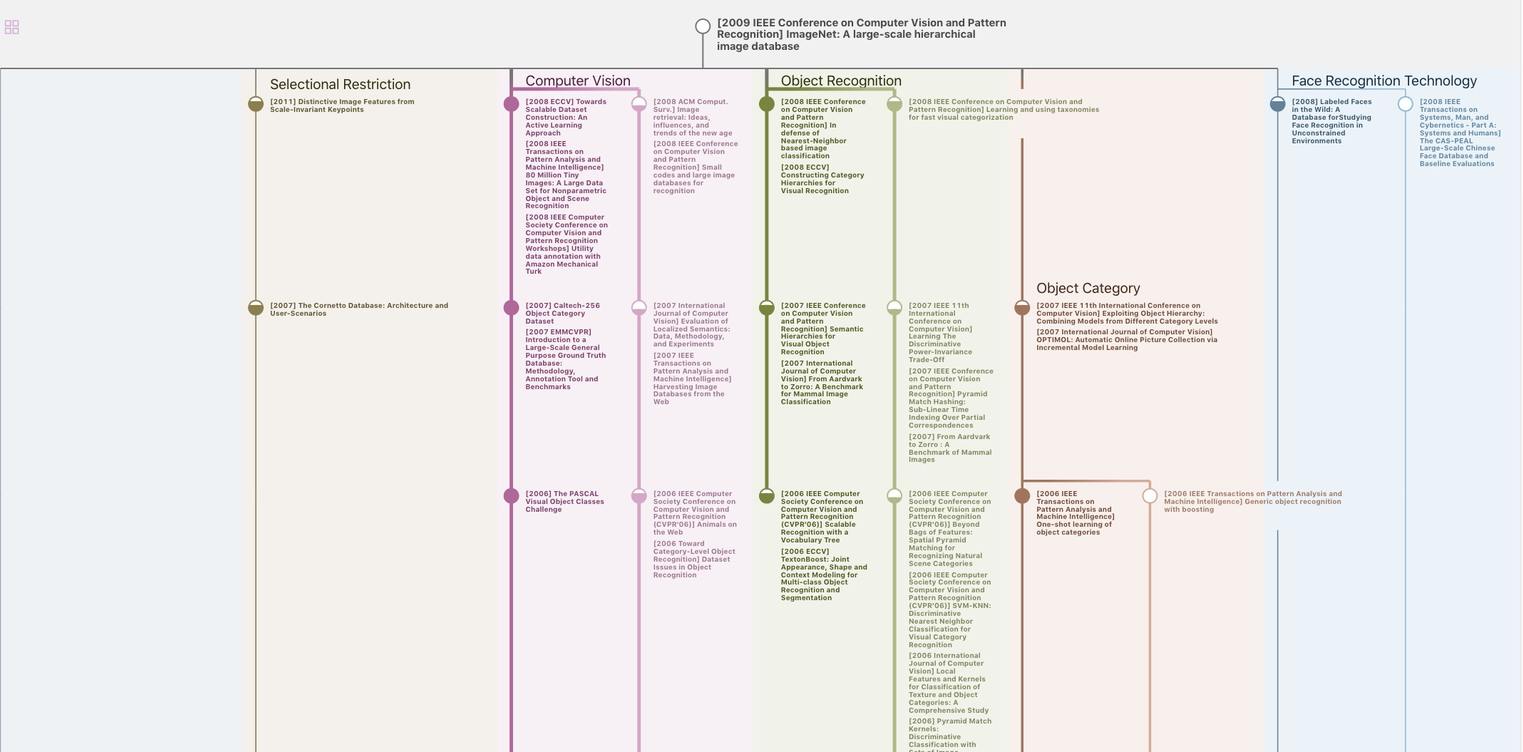
生成溯源树,研究论文发展脉络
Chat Paper
正在生成论文摘要