Optimal Strategies For Comparing Covariates To Solve Matching Problems
2020 25TH INTERNATIONAL CONFERENCE ON PATTERN RECOGNITION (ICPR)(2021)
摘要
Many machine learning tasks can be posed as matching problems in which we are given a "probe" entry that we expect matches some of the entries in our "gallery". The general solution to these problems is to retrieve matching entries based on statistical dependencies between the probe and the gallery data that are learned using complex models. Often, however, there are other common covariates to the probe and gallery data which might be easily inferred and may explain some of the statistical dependencies between the two. In this paper we present a probabilistic framework to derive optimal matching strategies based only on covariate features for three broad tasks, namely N-way classification, pairwise verification and ranking. We use canonical metrics to determine the maximum performance that can be expected if only covariate features are used and determine the marginal gain of using complex models. We find that covariate matching achieves an EER within 10% of a CNN in the verification task, and an MAP within 22% of the a DNN based model in the ranking task.
更多查看译文
AI 理解论文
溯源树
样例
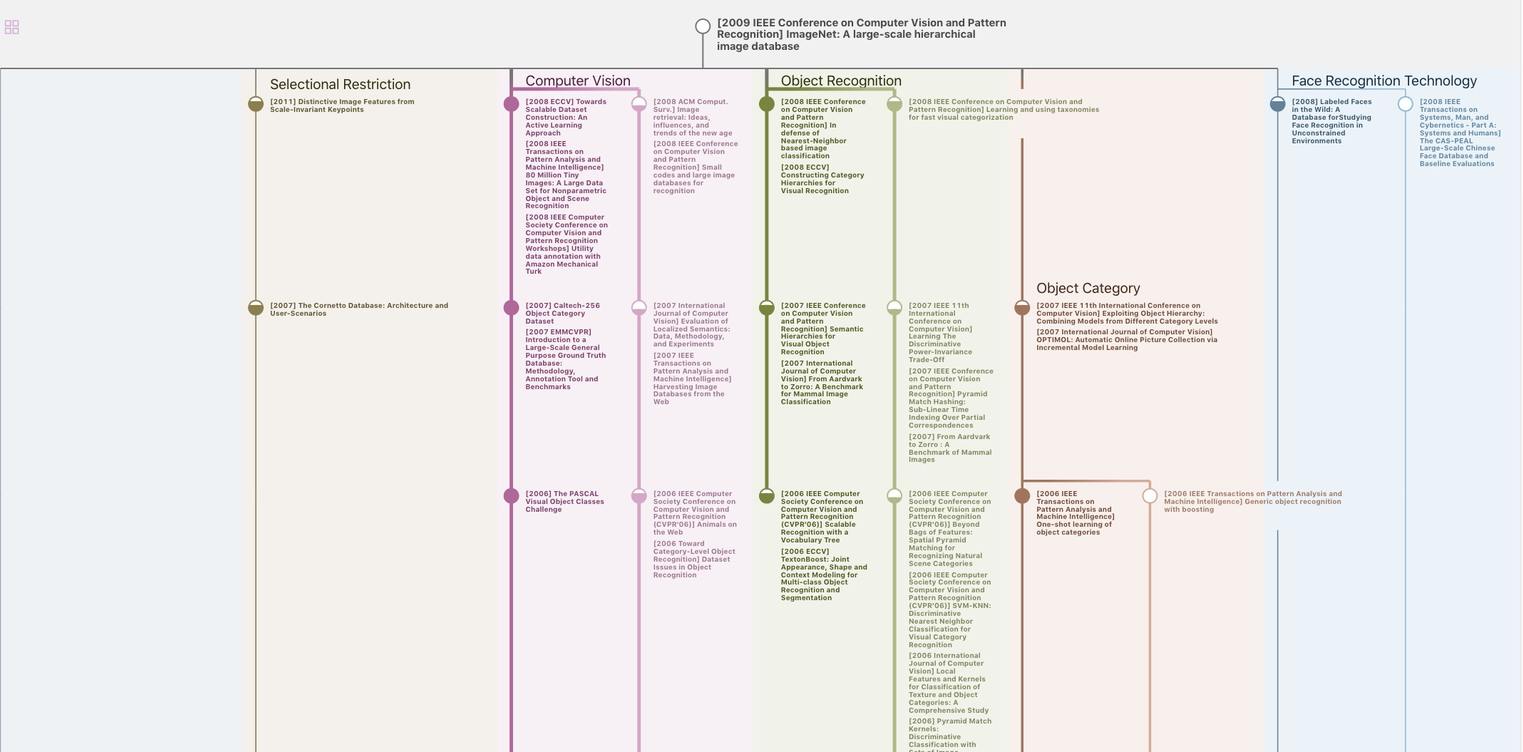
生成溯源树,研究论文发展脉络
Chat Paper
正在生成论文摘要