Explainable Feature Embedding Using Convolutional Neural Networks For Pathological Image Analysis
2020 25TH INTERNATIONAL CONFERENCE ON PATTERN RECOGNITION (ICPR)(2020)
摘要
The development of computer-assisted diagnosis (CAD) algorithms for pathological image analysis is an important research topic. Recently, convolutional neural networks (CNNs) have been used in several studies for the development of CAD algorithms. Such systems are required to be not only accurate but also explainable for their decisions, to ensure reliability. However, a limitation of using CNNs is that the basis of the decisions made by them are hardly interpretable by humans. Thus, in this paper, we present an explainable diagnosis method comprising two CNNs playing different roles. This method allows us to interpret the basis of the decisions made by the CNN from two perspectives: statistics and visualization. For the statistical explanation, the method constructs a dictionary of representative pathological features. It performs diagnoses based on the occurrence and importance of learned features referred from its dictionary. To construct the dictionary, we introduce a vector quantization scheme for CNN. For the visual interpretation, the method provides images of learned features embedded in a feature space as an index of the dictionary by generating them using a conditional autoregressive model. The experimental results showed that the proposed network learned pathological features that contributed to the diagnosis and yielded an area under the receiver operating curve (AUC) of approximately 0.93 for detecting atypical tissues in pathological images of the uterine cervix. Moreover, the proposed method demonstrated that it could provide visually interpretable images to show the rationales behind its decisions. Thus, the proposed method can serve as a valuable tool for pathological image analysis in terms of both its accuracy and explainability.
更多查看译文
关键词
Explainable AI, Convolutional neural networks, Pathological images
AI 理解论文
溯源树
样例
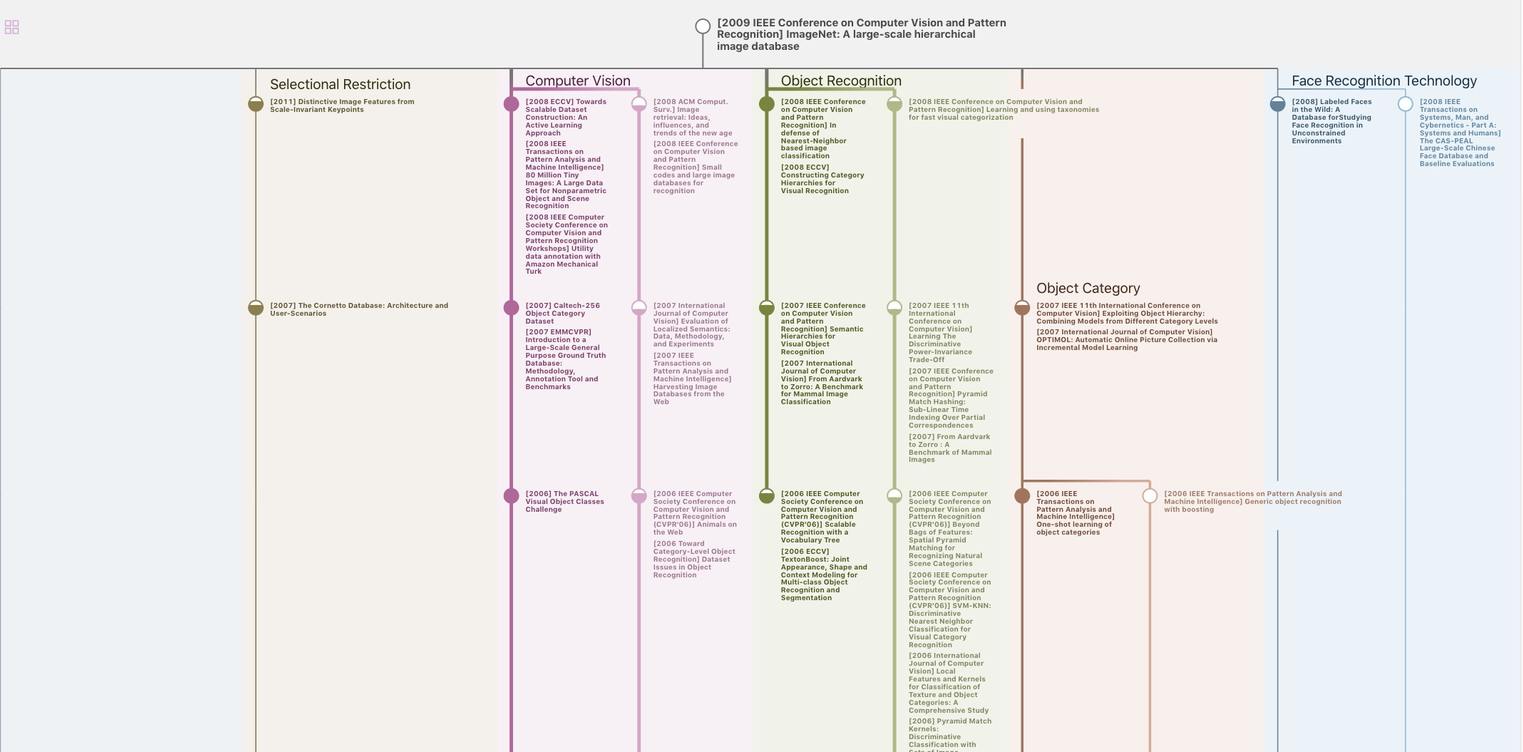
生成溯源树,研究论文发展脉络
Chat Paper
正在生成论文摘要