Semi-Supervised GANs with Complementary Generator Pair for Retinopathy Screening
2020 25TH INTERNATIONAL CONFERENCE ON PATTERN RECOGNITION (ICPR)(2021)
摘要
Several typical types of retinopathy are major causes of blindness. However, early detection of retinopathy is quite not easy since few symptoms are observable in the early stage, attributing to the development of non-mydriatic retinal cameras, these cameras produce high-resolution retinal fundus images that provide the possibility of Computer-Aided-Diagnosis (CAD) via deep learning to assist diagnosing retinopathy. Deep learning algorithms usually rely on a large number of labeled images that are expensive and time-consuming to obtain in the medical imaging area. Moreover, the random distribution of various lesions that often vary greatly in size also brings significant challenges to learn discriminative information from high-resolution fundus images. In this paper, we present generative adversarial networks simultaneously equipped with a “good” generator and a “bad” generator (GBGANs) to make up for the incomplete data distribution given limited fundus images. To improve the generative feasibility of the generator, we introduce a pre-trained feature extractor to acquire condensed features for each fundus image in advance. Experimental results on integrated three public iChallenge datasets show that the proposed GBGANs could fully utilize the available fundus images to identify retinopathy with little label cost.
更多查看译文
关键词
complementary generator pair,retinopathy screening,typical types,nonmydriatic retinal cameras,high-resolution retinal fundus images,Computer-Aided-Diagnosis,deep learning,diagnosing retinopathy,labeled images,medical imaging area,random distribution,high-resolution fundus images,generative adversarial networks,good generator,bad generator,incomplete data distribution,fundus image,generative feasibility,available fundus images
AI 理解论文
溯源树
样例
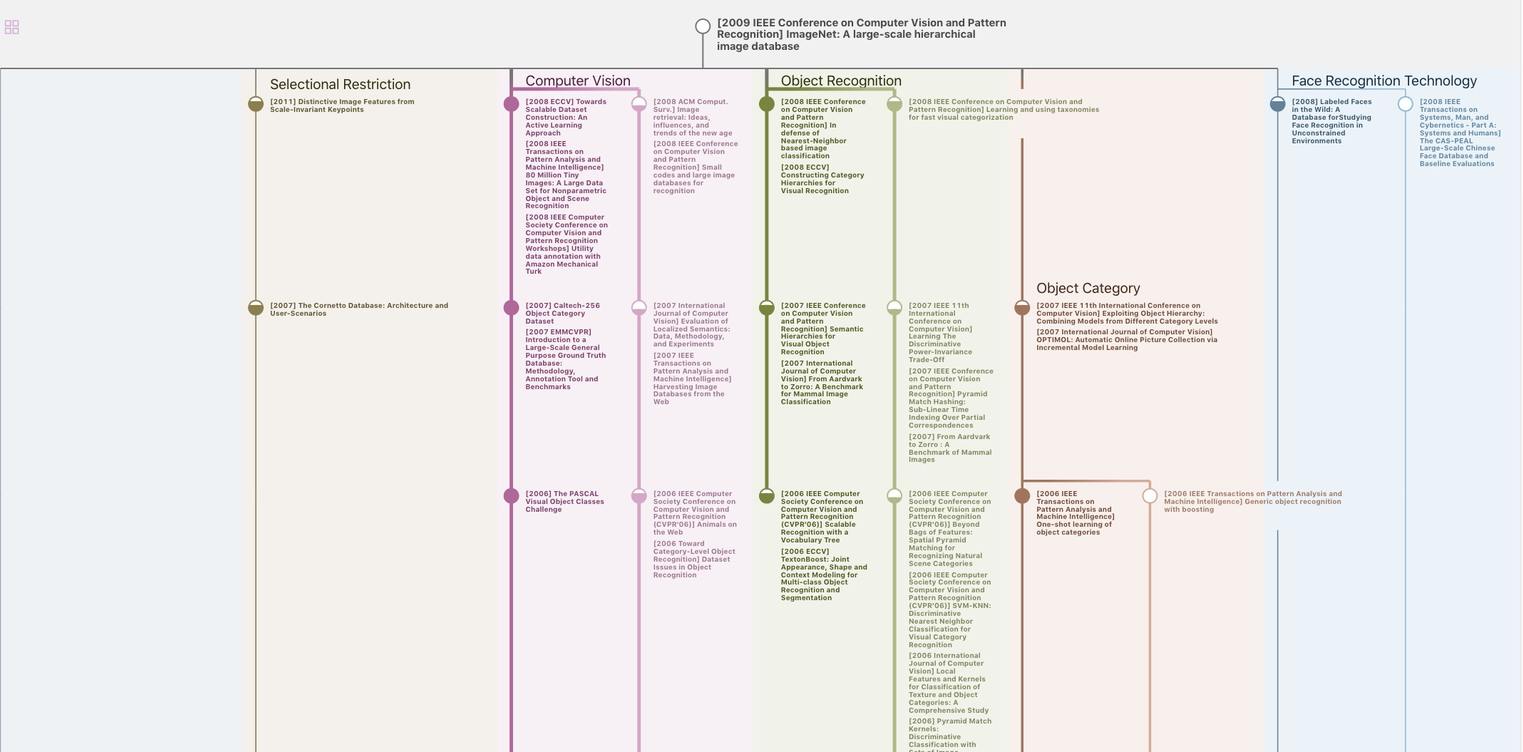
生成溯源树,研究论文发展脉络
Chat Paper
正在生成论文摘要