Soft Attention Improves Skin Cancer Classification Performance
arxiv(2021)
摘要
In clinical applications, neural networks must focus on and highlight the most important parts of an input image. Soft-Attention mechanism enables a neural network to achieve this goal. This paper investigates the effectiveness of Soft-Attention in deep neural architectures. The central aim of Soft-Attention is to boost the value of important features and suppress the noise-inducing features. We compare the performance of VGG, ResNet, Inception ResNet v2 and DenseNet architectures with and without the Soft-Attention mechanism, while classifying skin lesions. The original network when coupled with Soft-Attention outperforms the baseline [16] by 4.7% while achieving a precision of 93.7% on HAM10000 dataset [25]. Additionally, Soft-Attention coupling improves the sensitivity score by 3.8% compared to baseline [31] and achieves 91.6% on ISIC-2017 dataset [2]. The code is publicly available at github (https://github.com/skrantidatta/Attention-based-Skin-Cancer-Classification).
更多查看译文
关键词
skin cancer classification performance,skin cancer,attention
AI 理解论文
溯源树
样例
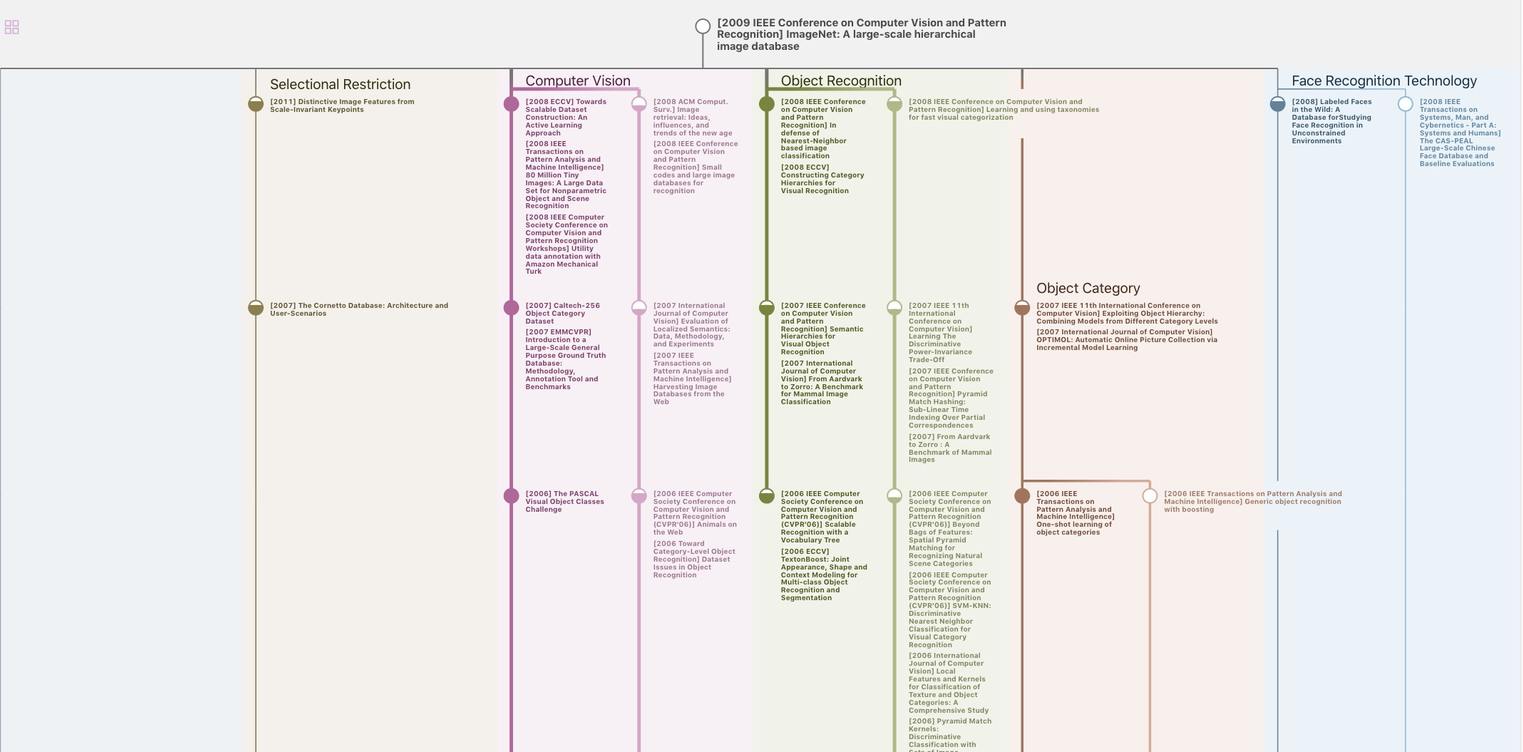
生成溯源树,研究论文发展脉络
Chat Paper
正在生成论文摘要