Matching Visual Features to Hierarchical Semantic Topics for Image Paragraph Captioning
International Journal of Computer Vision(2022)
摘要
Observing a set of images and their corresponding paragraph-captions, a challenging task is to learn how to produce a semantically coherent paragraph to describe the visual content of an image. Inspired by recent successes in integrating semantic topics into this task, this paper develops a plug-and-play hierarchical-topic-guided image paragraph generation framework, which couples a visual extractor with a deep topic model to guide the learning of a language model. To capture the correlations between the image and text at multiple levels of abstraction and learn the semantic topics from images, we design a variational inference network to build the mapping from image features to textual captions. To guide the paragraph generation, the learned hierarchical topics and visual features are integrated into the language model, including Long Short-Term Memory and Transformer, and jointly optimized. Experiments on public datasets demonstrate that the proposed models, which are competitive with many state-of-the-art approaches in terms of standard evaluation metrics, can be used to both distill interpretable multi-layer semantic topics and generate diverse and coherent captions.
更多查看译文
关键词
Image paragraph generation, Deep topic model, Language model, Image and text
AI 理解论文
溯源树
样例
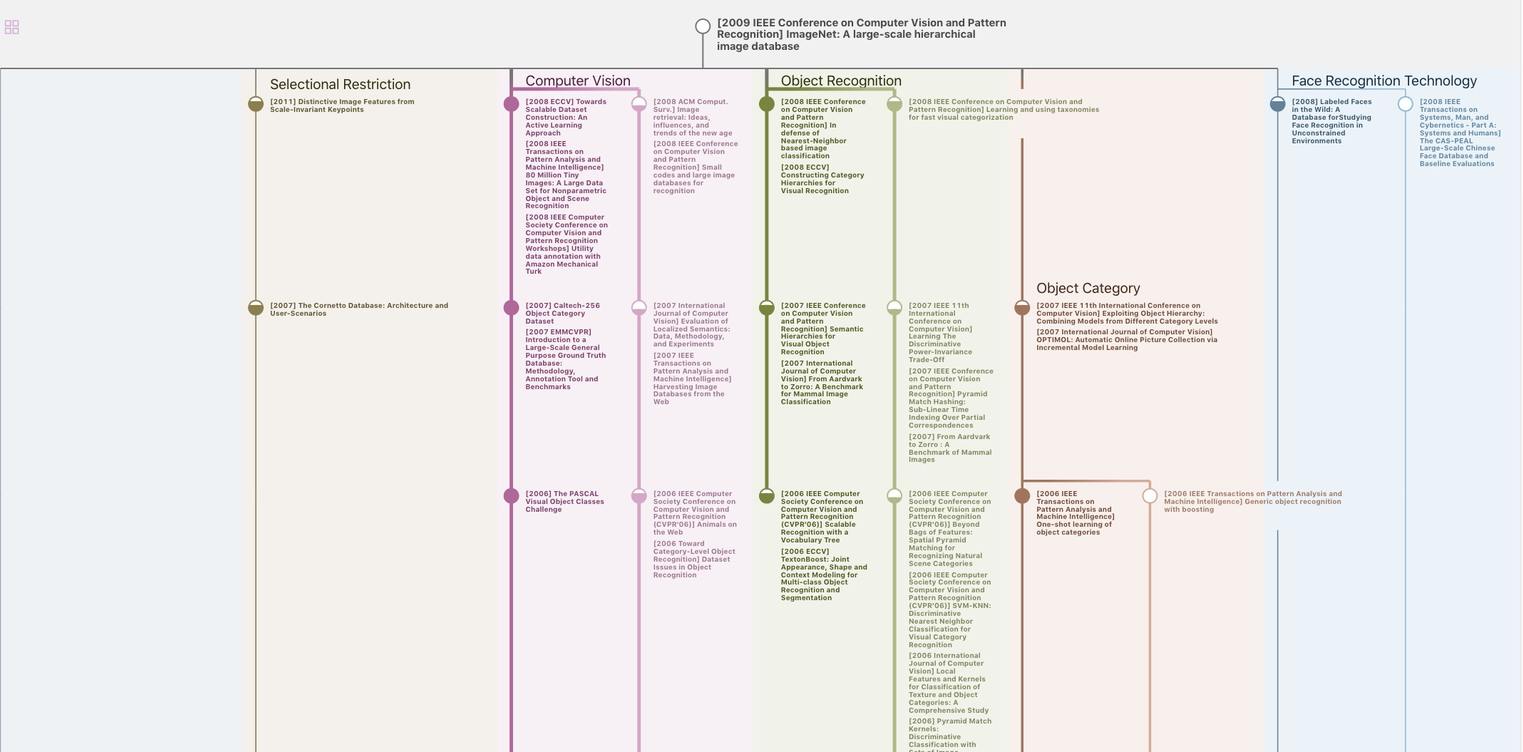
生成溯源树,研究论文发展脉络
Chat Paper
正在生成论文摘要