A comprehensive and fast microplastics identification based on near-infrared hyperspectral imaging (HSI-NIR) and chemometrics
Environmental Pollution(2021)
摘要
Microplastic pollution is a global concern theme, and there is still the need for less laborious and faster analytical methods aiming at microplastics detection. This article describes a high throughput screening method based on near-infrared hyperspectral imaging (HSI-NIR) to identify microplastics in beach sand automatically with minimum sample preparation. The method operates directly in the entire sample or on its retained fraction (150 μm–5 mm) after sieving. Small colorless microplastics (<600 μm) that would probably be imperceptible as a microplastic by visual inspection, or missed during manual pick up, can be easily detected. No spectroscopic subsampling was performed due to the high-speed analysis of line-scan instrumentation, allowing multiple microplastics to be assessed simultaneously (video available). This characteristic is an advantage over conventional infrared (IR) spectrometers. A 75 cm2 scan area was probed in less than 1 min at a pixel size of 156 × 156 μm. An in-house comprehensive spectral dataset, including weathered microplastics, was used to build multivariate supervised soft independent modelling of class analogy (SIMCA) classification models. The chemometric models were validated for hundreds of microplastics (primary and secondary) collected in the environment. The effect of particle size, color and weathering are discussed. Models' sensitivity and specificity for polyethylene (PE), polypropylene (PP), polyamide-6 (PA), polyethylene terephthalate (PET) and polystyrene (PS) were over 99% at the defined statistical threshold. The method was applied to a sand sample, identifying 803 particles without prior visual sorting, showing automatic identification was robust and reliable even for weathered microplastics analyzed together with other matrix constituents. The HSI-NIR-SIMCA described is also applicable for microplastics extracted from other matrices after sample preparation. The HSI-NIR principals were compared to other common techniques used to microplastic chemical characterization. The results show the potential to use HSI-NIR combined with classification models as a comprehensive microplastic-type characterization screening.
更多查看译文
关键词
Microplastic,Marine debris,Near-infrared hyperspectral imaging,SWIR hyperspectral imaging,Machine learning
AI 理解论文
溯源树
样例
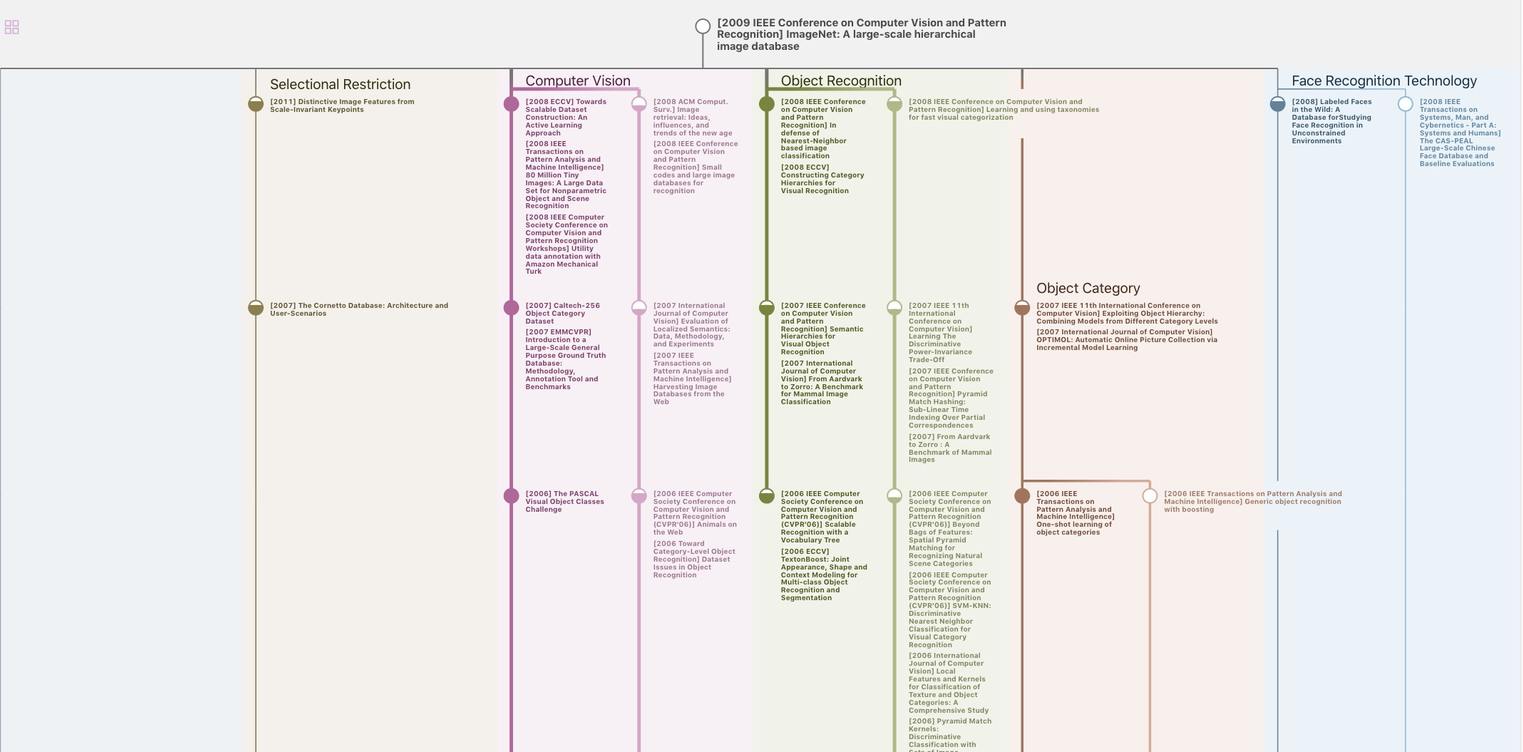
生成溯源树,研究论文发展脉络
Chat Paper
正在生成论文摘要