Near-lossless Compression for Sparse Source Using Convolutional Low Density Generator Matrix Codes
2021 Data Compression Conference (DCC)(2021)
摘要
In this paper, we present a new coding approach to near-lossless compression for binary sparse sources by using a special class of low density generator matrix (LDGM) codes. On the theoretical side, we proved that such a class of block LDGM codes are universal in the sense that any source with an entropy less than the coding rate can be compressed and reconstructed with an arbitrarily low bit-error rate (BER). On the practical side, we employ spatially coupled LDGM codes to reduce the complexity of reconstruction by implementing an iterative sliding window decoding algorithm. Figure of merits of the proposed scheme include its flexibility and universality. The encoder does not require the knowledge of the source statistic, while the decoder can estimate easily the source parameter as required by the iterative decoding. The implementation complexity is analyzed and the performance is simulated. Numerical results show that the proposed scheme performs well over a wide range of sources.
更多查看译文
关键词
Convolutional codes,Data compression,Generators,Encoding,Iterative algorithms,Entropy,Complexity theory
AI 理解论文
溯源树
样例
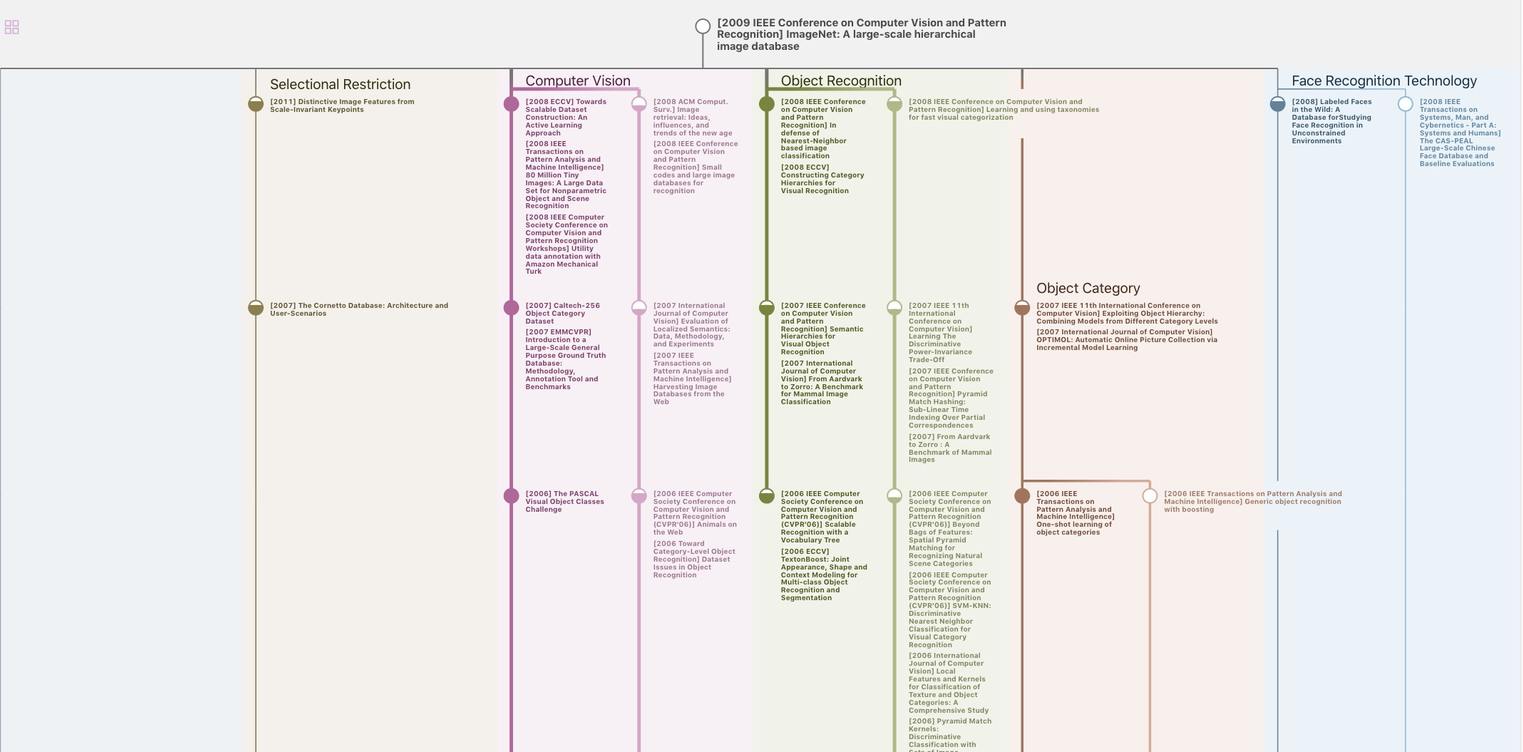
生成溯源树,研究论文发展脉络
Chat Paper
正在生成论文摘要