Cloud Detection From Remote Sensing Imagery Based on Domain Translation Network
IEEE GEOSCIENCE AND REMOTE SENSING LETTERS(2022)
摘要
Cloud detection in optical imagery has drawn remarkable attention in the era of big Earth observation data analytic. While multiple supervised learning models have been developed for such purpose, large volumes of paired training samples annotated at the pixel level are essential to ensure the model's generalization capacity. However, constructing a comprehensive cloud detection training database is a tedious and time-consuming process. To tackle this dilemma, we simply regard cloud-contaminated remote sensing (RS) imagery as the combination of cloud and background domains and propose a cloud detection framework based on image-to-image domain translation network (DTNet) to separate cloud-contaminated RS imagery into two target domains of cloud and background object images without using any paired and pixel-level annotation training data. The framework was evaluated with multispectral images from two types of sensors, Landsat-8 Operational Land Imager (OLI) (30 m) and GaoFen-1 (16 m), and demonstrated superior or comparable performance compared with several state-of-the-art cloud detection models.
更多查看译文
关键词
Remote sensing, Earth, Generators, Artificial satellites, Training, Data models, Satellites, Cloud detection, domain translation network (DTNet), remote sensing (RS) imagery
AI 理解论文
溯源树
样例
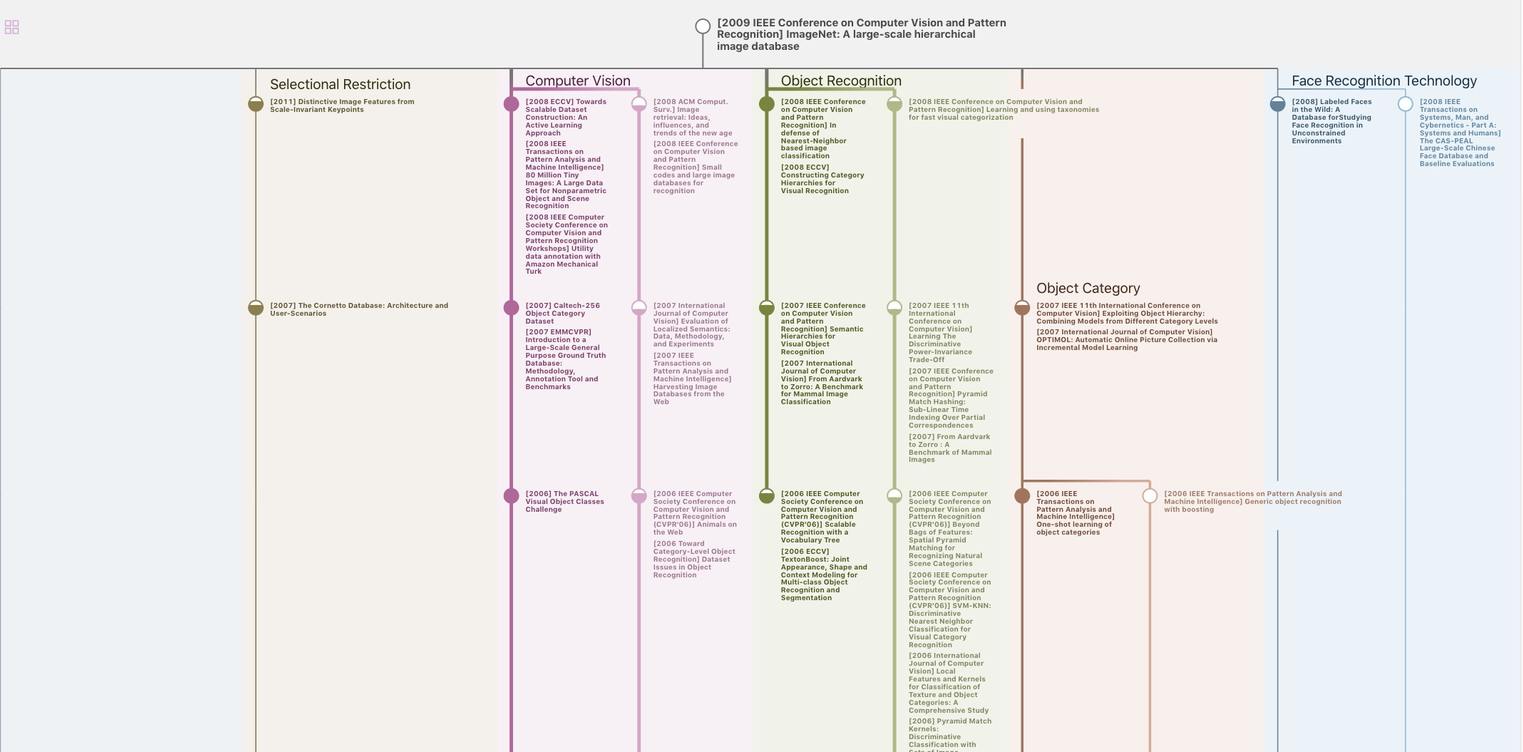
生成溯源树,研究论文发展脉络
Chat Paper
正在生成论文摘要