Learning Convergence Prediction Of Astrobots In Multi-Object Spectrographs
JOURNAL OF ASTRONOMICAL TELESCOPES INSTRUMENTS AND SYSTEMS(2021)
摘要
Astrobot swarms are used to capture astronomical signals to generate the map of the observable universe for the purpose of dark energy studies. The convergence of each swarm in the course of its coordination has to surpass a particular threshold to yield a satisfactory map. The current coordination methods do not always reach desired convergence rates. Moreover, these methods are so complicated that one cannot formally verify their results without resource demanding simulations. Thus we use support vector machines to train a model that can predict the convergence of a swarm based on the data of previous coordination of that swarm. Given a fixed parity, i.e., the rotation direction of the outer arm of an astrobot, corresponding to a swarm, our algorithm reaches a better predictive performance compared to the state of the art. Additionally, we revise our algorithm to solve a more generalized convergence prediction problem according to which the parities of astrobots may differ. We present the prediction results of a generalized scenario, associated with a 487-astrobot swarm, which are interestingly efficient and collision free given the excessive complexity of this scenario compared to the constrained one. (C) 2021 Society of Photo-Optical Instrumentation Engineers (SPIE)
更多查看译文
关键词
astrobots, coordination, convergence prediction, multi-object spectrographs, massive spectroscpic surveys
AI 理解论文
溯源树
样例
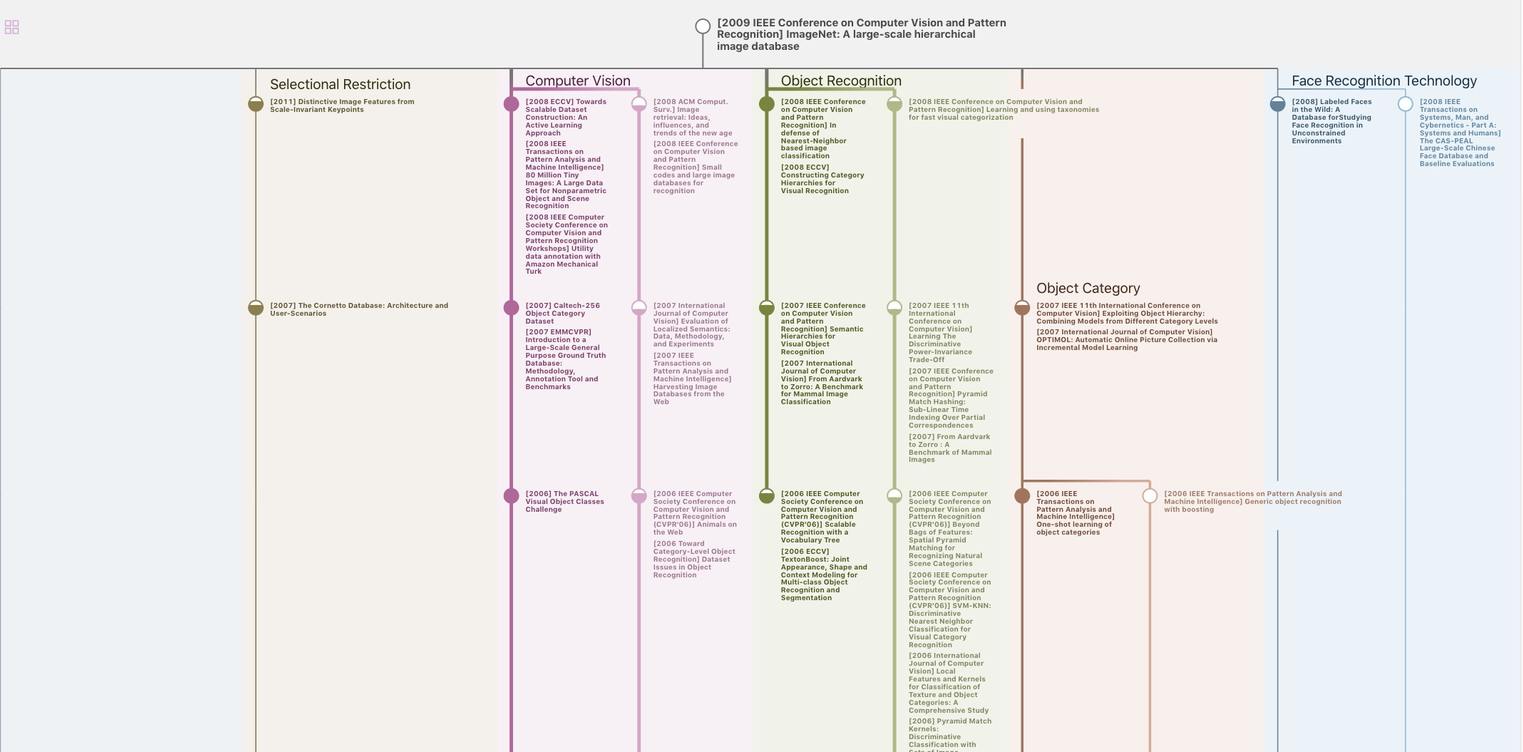
生成溯源树,研究论文发展脉络
Chat Paper
正在生成论文摘要