Improved Modeling of Spatiotemporal Variations of Fine Particulate Matter Using a Three‐Dimensional Variational Data Fusion Method
Journal of geophysical research Atmospheres(2021)
摘要
The spatiotemporal concentration of multiple pollutants is crucial information for pollution control strategies to safeguard public health. Despite considerable efforts, however, significant uncertainty remains. In this study, a three-dimensional variational model is coupled with a data assimilation (DA) system to analyze the spatiotemporal variation of PM2.5 for the whole of China. Monthly simulations of six sensitivity scenarios in different seasons, including different assimilation cycles, are carried out to assess the impact of the assimilation frequency on the PM2.5 simulations and the model simulation accuracy afforded by DA. The results show that the coupled system provides more reliable initial fields to substantially improve the model performance for PM2.5, PM10, and O-3. Higher assimilation frequency improves the simulation in all geographic areas. Two statistical indicators-the root mean square error and the correlation coefficient of PM2.5 mass concentrations in the analysis field-are improved by 12.19 mu g/m(3) (33%) and 0.21 (48%), respectively. Although the 24-h assimilation cycle considerably improves the model, assimilation at a 6-h cycle raises the performance for PM2.5 to the performance goal level. The analysis shows that assimilating at a 24-h cycle diminishes over time, whereas the positive impact of the 6-h cycle persists. One pivotal finding is that the assimilation of PM2.5 in the outermost domain results in a substantial improvement in PM2.5 prediction for the innermost domain, which is a potential alternative method to the existing domain-wide data fusion algorithm. The effect of assimilation varies among topographies, a finding that provides essential support for further model development.
更多查看译文
关键词
assimilation cycles,China,coupling system,data fusion,spatiotemporal model
AI 理解论文
溯源树
样例
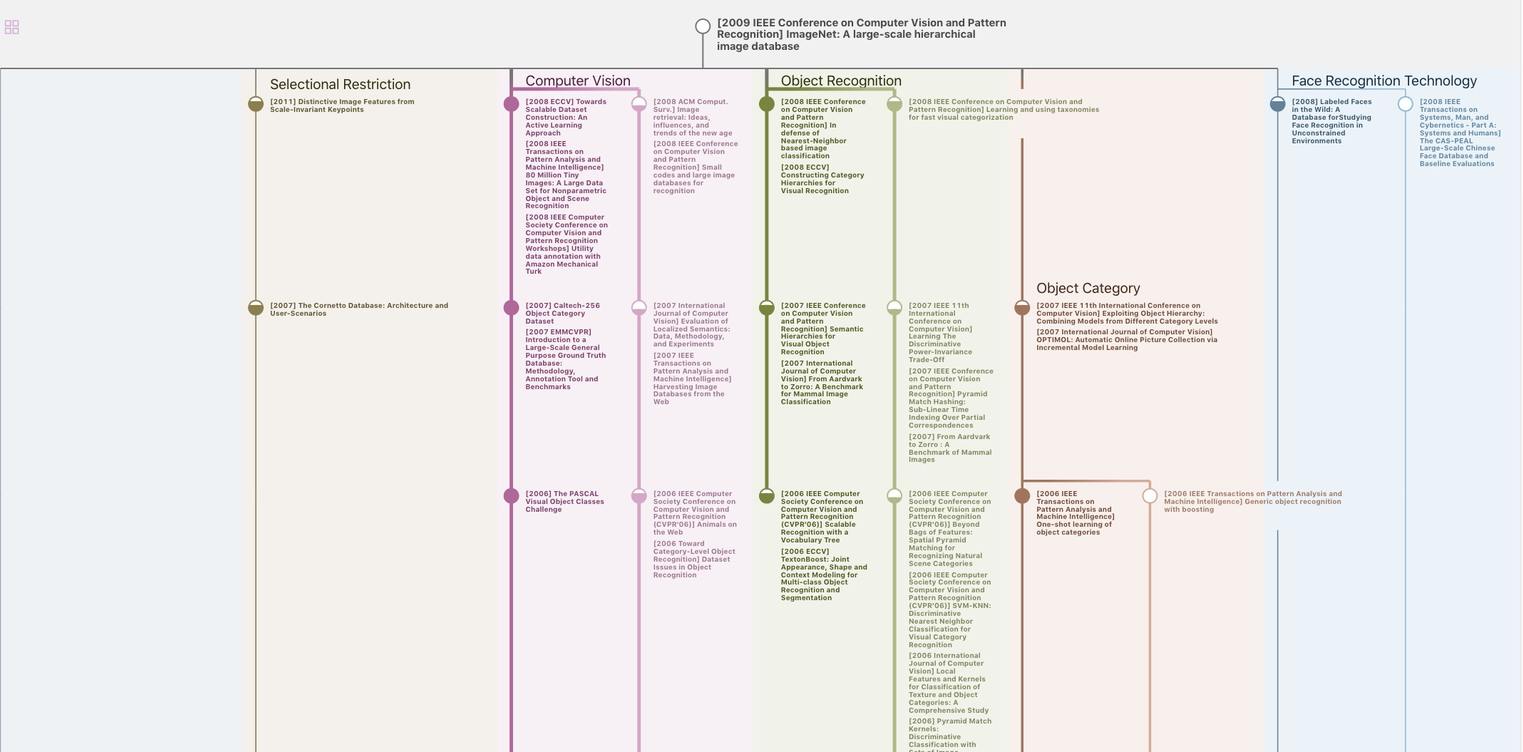
生成溯源树,研究论文发展脉络
Chat Paper
正在生成论文摘要