Variational Instance-Adaptive Graph for EEG Emotion Recognition
IEEE Transactions on Affective Computing(2023)
摘要
The individual differences and the dynamic uncertain relationships among different electroencephalogram (EEG) regions are essential factors that limit EEG emotion recognition. To address these issues, in this article, we propose a variational instance-adaptive graph method (V-IAG) that simultaneously captures the individual dependencies among different EEG electrodes and estimates the underlying uncertain information. Specifically, we employ two branches, i.e., instance-adaptive branch and variational branch, to construct the graph. Inspired by the attention mechanism, the instance-adaptive branch generates the graph based on the input so as to characterize the individual dependencies among EEG channels. The variational branch generates the probabilistic graph, which quantifies the uncertainties. We combine these two types of graphs to extract more discriminative features. To present more precise graph representation, we propose a new operation named the multi-level and multi-graph convolution operation, which aggregates the features of EEG channels from different frequencies with different graphs. Furthermore, we design the graph coarsening and employ the sparse constraint to obtain more robust features. We conduct extensive experiments on three widely-used EEG emotion recognition databases, i.e., SJTU emotion EEG dataset (SEED), multi-modal physiological emotion recognition dataset (MPED) and DREAMER. The results demonstrate that the proposed model achieves the-state-of-the-art performance.
更多查看译文
关键词
EEG emotion recognition,graph neural network,variational inference
AI 理解论文
溯源树
样例
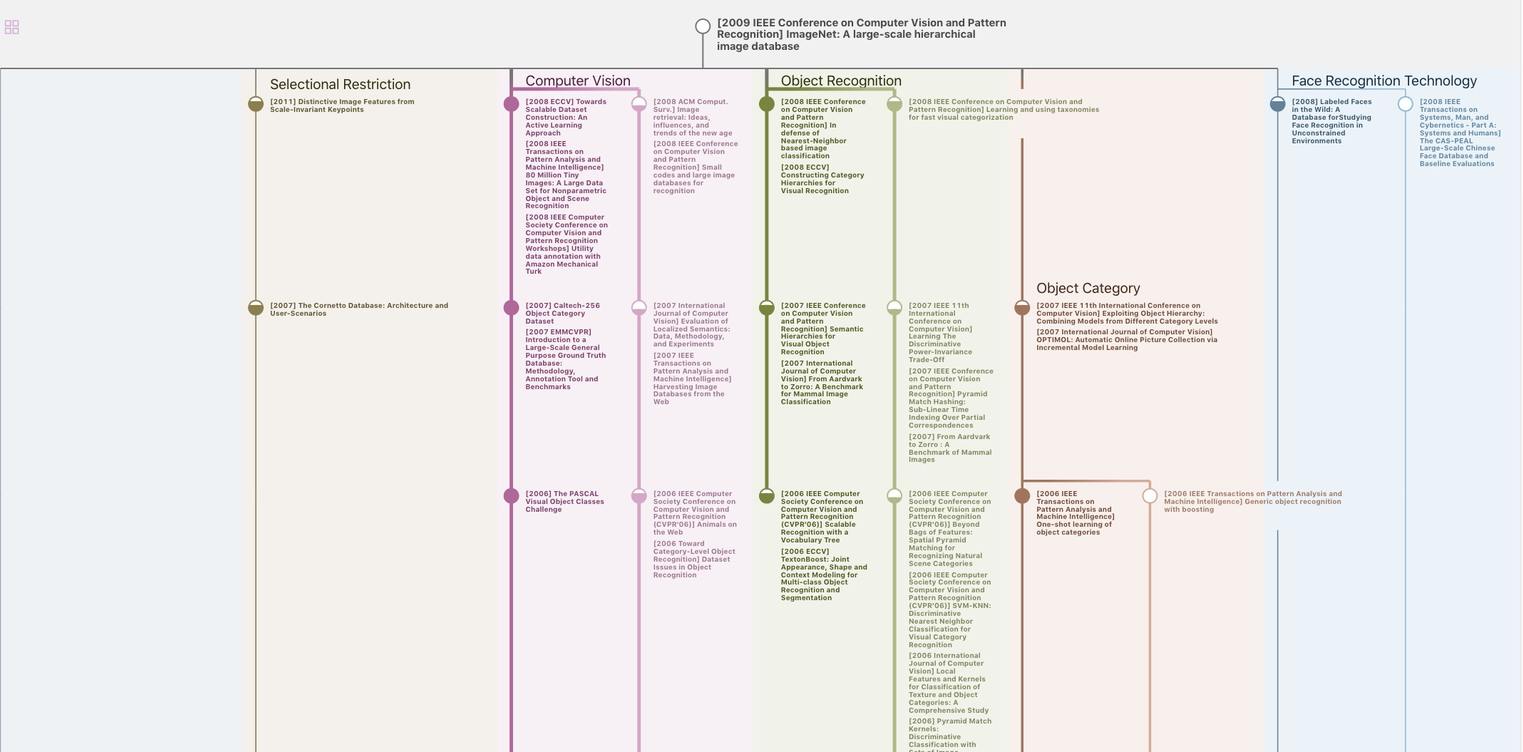
生成溯源树,研究论文发展脉络
Chat Paper
正在生成论文摘要