Super-Resolution Reconstruction Of Single Remote Sensing Images Based On Residual Channel Attention
JOURNAL OF APPLIED REMOTE SENSING(2021)
摘要
The existing methods of remote sensing image super-resolution reconstruction based on deep learning have some problems, such as insufficient feature extraction abilities, blurred image edges, and difficult model training. To solve these problems, a super-resolution reconstruction method combining residual channel attention (CA) is proposed. Based on the framework of generative adversarial networks, the residual structure is designed to enhance the ability of deep feature extraction ability for remote sensing images. The CA module is added to extract the deep feature information of remote sensing images, and the shallow features and deep features are fused using the skip connection. The perceptual loss function is combined with the loss function represented by the Wasserstein distance to improve the stability of model training. The experimental results show that this method is superior to the comparison algorithms in the objective evaluation criteria of the peak-signal-to-noise ratio and structural similarity of the reconstructed remote sensing images. After optimizing the model training process, the reconstructed remote sensing images are visually clearer and have sharper edges. (C) 2021 Society of Photo-Optical Instrumentation Engineers (SPIE)
更多查看译文
关键词
remote sensing image, super-resolution reconstruction, generative adversarial network, residual channel attention
AI 理解论文
溯源树
样例
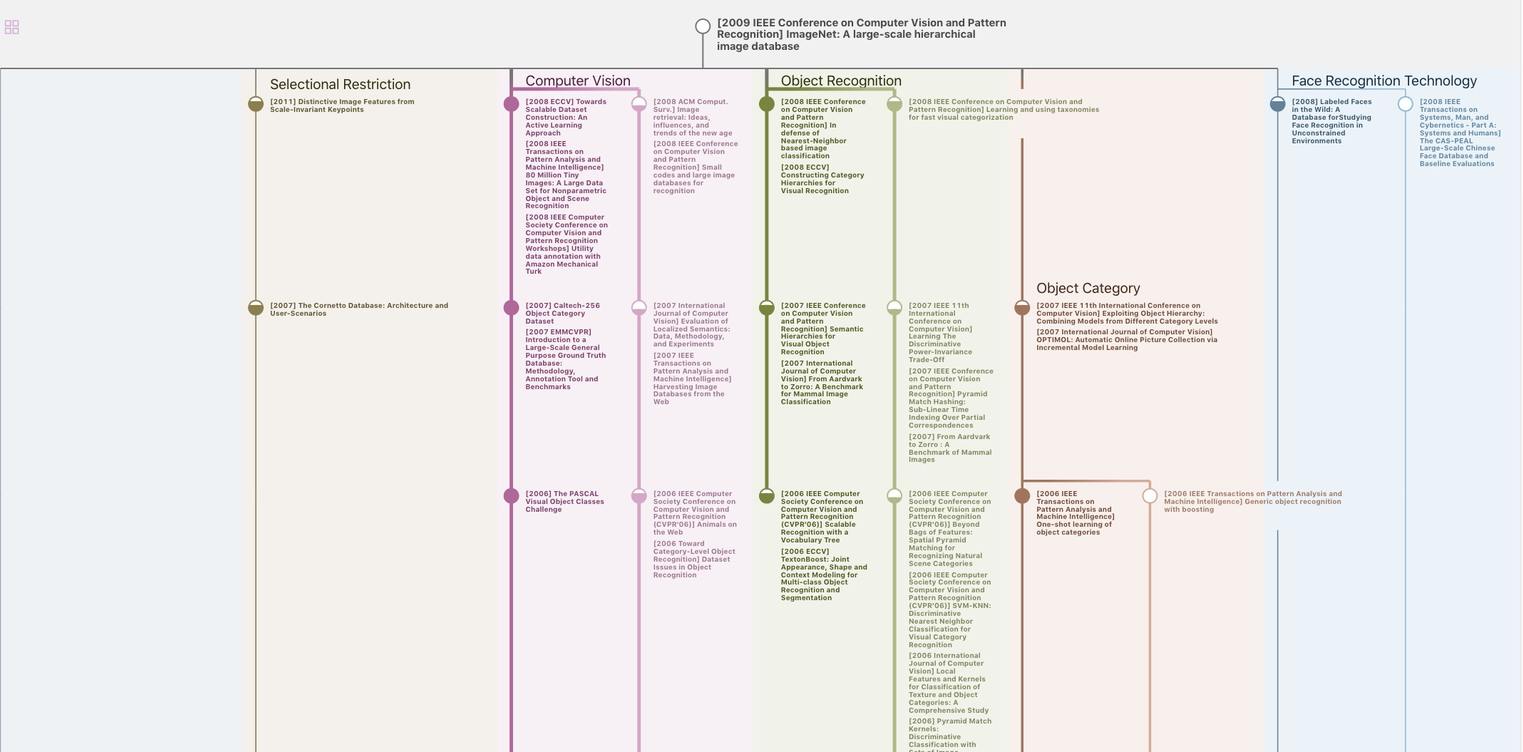
生成溯源树,研究论文发展脉络
Chat Paper
正在生成论文摘要