Incremental learning of iterated dependencies
Machine Learning(2021)
摘要
We study some learnability problems in the family of Categorial Dependency Grammars (CDG), a class of categorial grammars defining dependency structures. CDG is a formal system, where types are attached to words, combining the classical categorial grammars’ elimination rules with valency pairing rules defining non-projective (discontinuous) dependencies; very importantly, the elimination rules are naturally extended to the so called “iterated dependencies” expressed by a specific type constructor and related elimination rules. This paper first reviews key points on negative results: even the rigid (one type per word) CDG cannot be learned neither from function/argument structures, nor even from dependency structures themselves. Such negative results prove the impossibility to define a learning algorithm for these grammar classes. Nevertheless, we show that the CDG satisfying reasonable and linguistically valid conditions on the iterated dependencies are incrementally learnable in the limit from dependency structures. We provide algorithms and also discuss these aspects for recent variants of the formalism that allow the inference of CDG from linguistic treebanks.
更多查看译文
关键词
Grammatical inference,Categorial grammar,Dependency grammar,Incremental learning,Iterated types,Treebanks
AI 理解论文
溯源树
样例
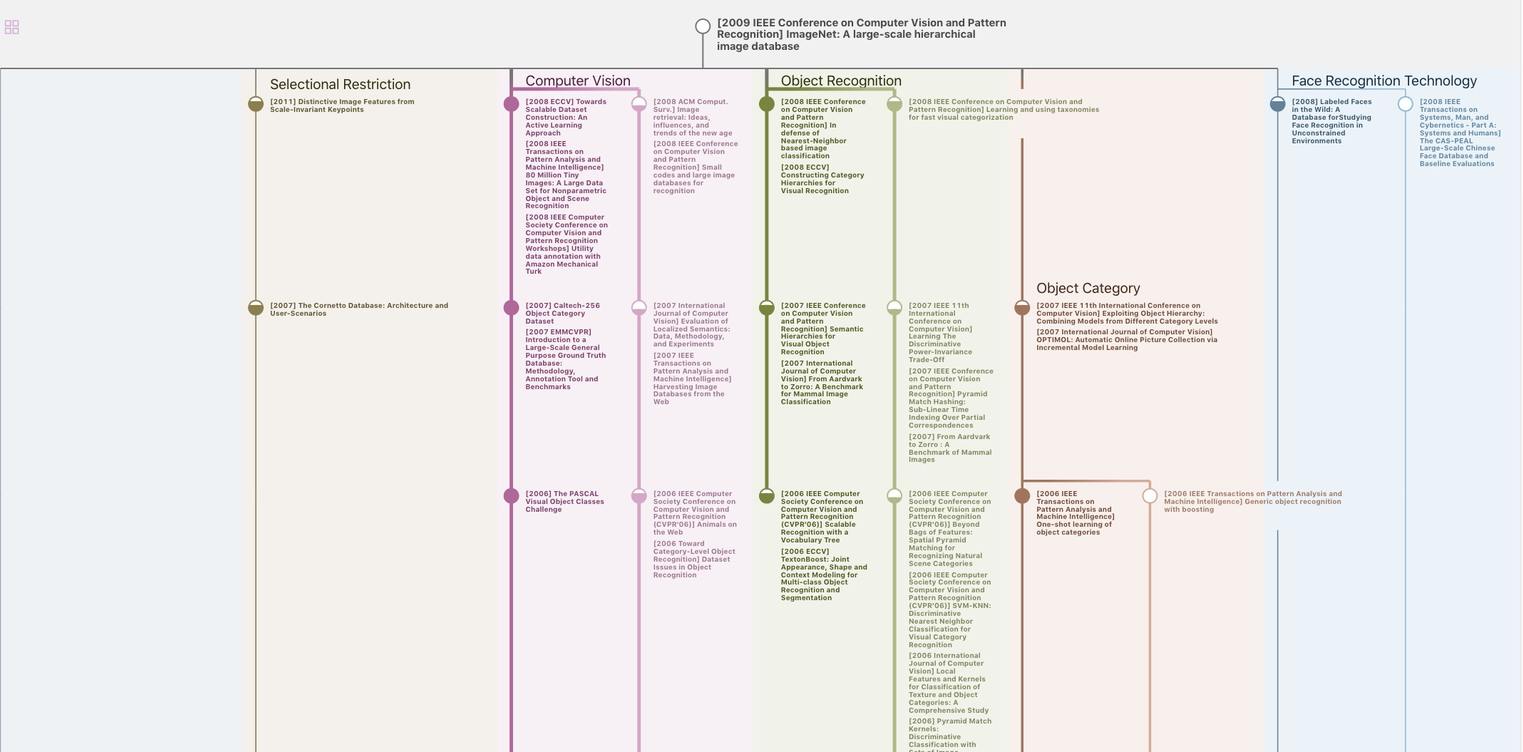
生成溯源树,研究论文发展脉络
Chat Paper
正在生成论文摘要