Learning to Detect Soft Shadow from Limited Data
The visual computer/The visual computer(2021)
摘要
Soft shadow is more challenging to detect than hard shadow due to its ambiguous boundary. Existing shadow detection methods pay more attention to hard shadow scene since collecting and annotating hard shadow images is more effortless. Motivated by that soft shadow has similar characteristics with hard shadow, and many traditional hard shadow datasets are publicly available, we propose a novel soft shadow detection method (namely Soft-DA) based on adversarial learning and domain adaptation scheme. Specifically, we create a limited soft shadow dataset, containing 1K soft shadow images with various scenes and shapes. Note that we just only need to annotate 0.4K shadow masks for semi-supervised learning. Besides, to tackle obvious domain discrepancy and potential intention difference between different datasets and similar tasks, we first align data distributions between domains by feature adversarial adaptation. And then, we introduce a novel detector separation strategy to tackle the intention difference issue. In this way, Soft-DA can effectively detect soft shadow with only a small number of soft shadow annotations. Extensive experiments demonstrate that our method can achieve superior performance to state of the arts.
更多查看译文
关键词
Deep learning,Image processing,Semi-supervised learning,Domain adaptation,Soft shadow detection
AI 理解论文
溯源树
样例
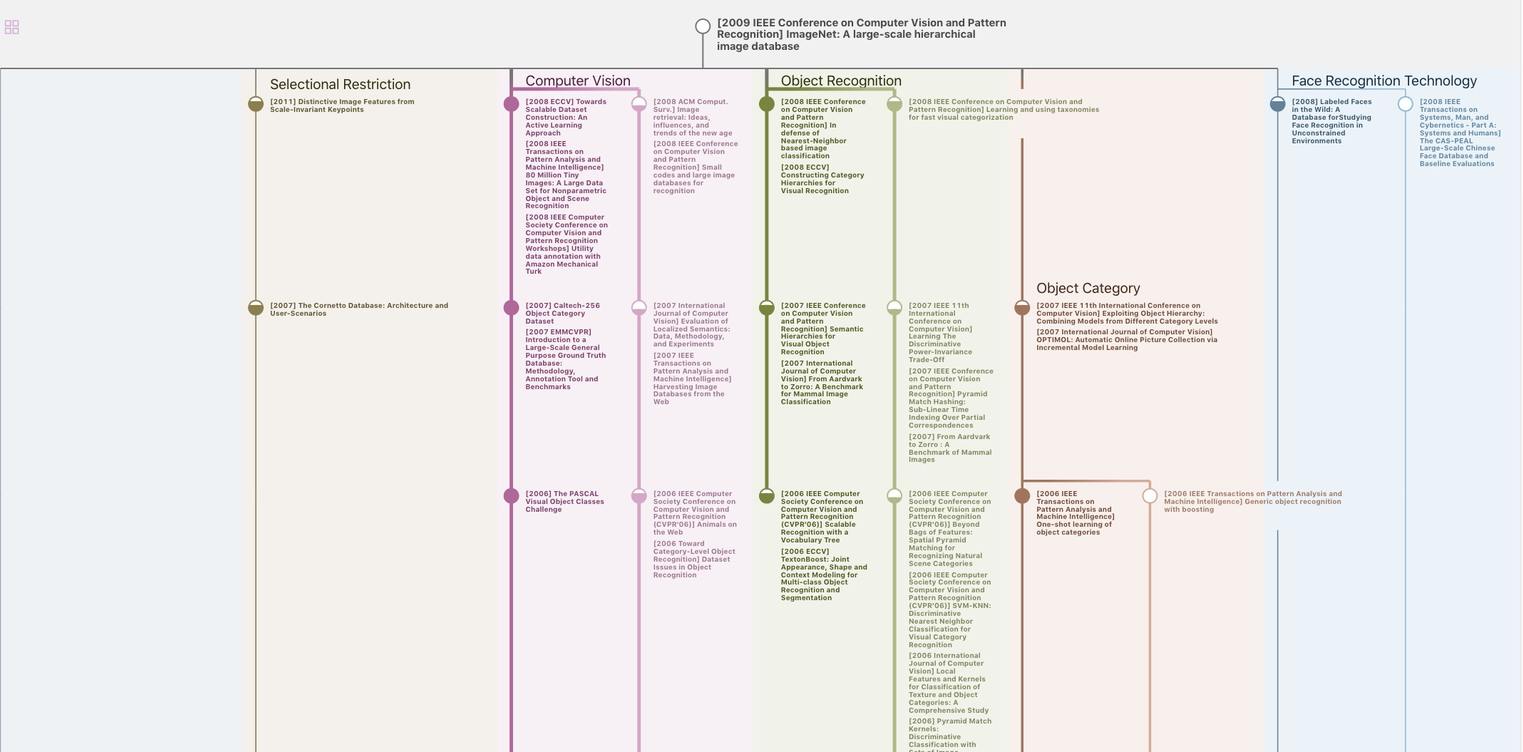
生成溯源树,研究论文发展脉络
Chat Paper
正在生成论文摘要