Comparison of Prediction Models for Two Different Peripheral Stem Cell Collection Protocols in Autologous Patients. How to Avoid Errors in Calculating Total Blood Volume to Process?
Acta Haematologica Polonica(2021)
摘要
Introduction: Calculating accurate blood volume to process is a critical practice in apheresis planning; therefore, researchers try to develop dedicated prediction models. In this analysis, we have attempted to compare three algorithms for two different apheresis collection protocols. Methods: In a retrospective study, we have analyzed 137 apheresis procedures performed on 100 autologous patients. Apheresis procedures were performed with the Spectra Optia apheresis device with two protocols: mononuclear cell collection (MNC) and continuous mononuclear cell collection (cMNC). Three algorithms: a model based on mean collection efficiency (CE2), a linear regression model, and a power regression model were validated by plotting collected CD34 + cell dose versus predicted CD34 + cell dose. Results: All models showed high predictability for MNC procedure, a high correlation of predicted CD34 + yield and actual CD34 + yield ( R 2 = 0.9547; 0.9487; 0.9474 for CE2-based model, linear and power regression model, respectively). In contrast, alteration between models for the cMNC procedure was greater ( R 2 = 0.8049, 0.7970, and 0.8169) with a higher number of overpredictions. Further analysis revealed that for low CD34 + precounts blood volume to process, calculated with the three models, differ significantly up to fivefold times. Conclusions: Utilizing regression models may lead to calculation errors, which can affect undercollection, repetition of apheresis, or even mobilization failure. Contrary to regression models, the model based on mean CE2 gave the most accurate prediction both for MNC and cMNC procedures. Although new prediction algorithms are created, this simple formula remains a reliable tool that promotes careful planning of apheresis, thus improving patient safety.
更多查看译文
AI 理解论文
溯源树
样例
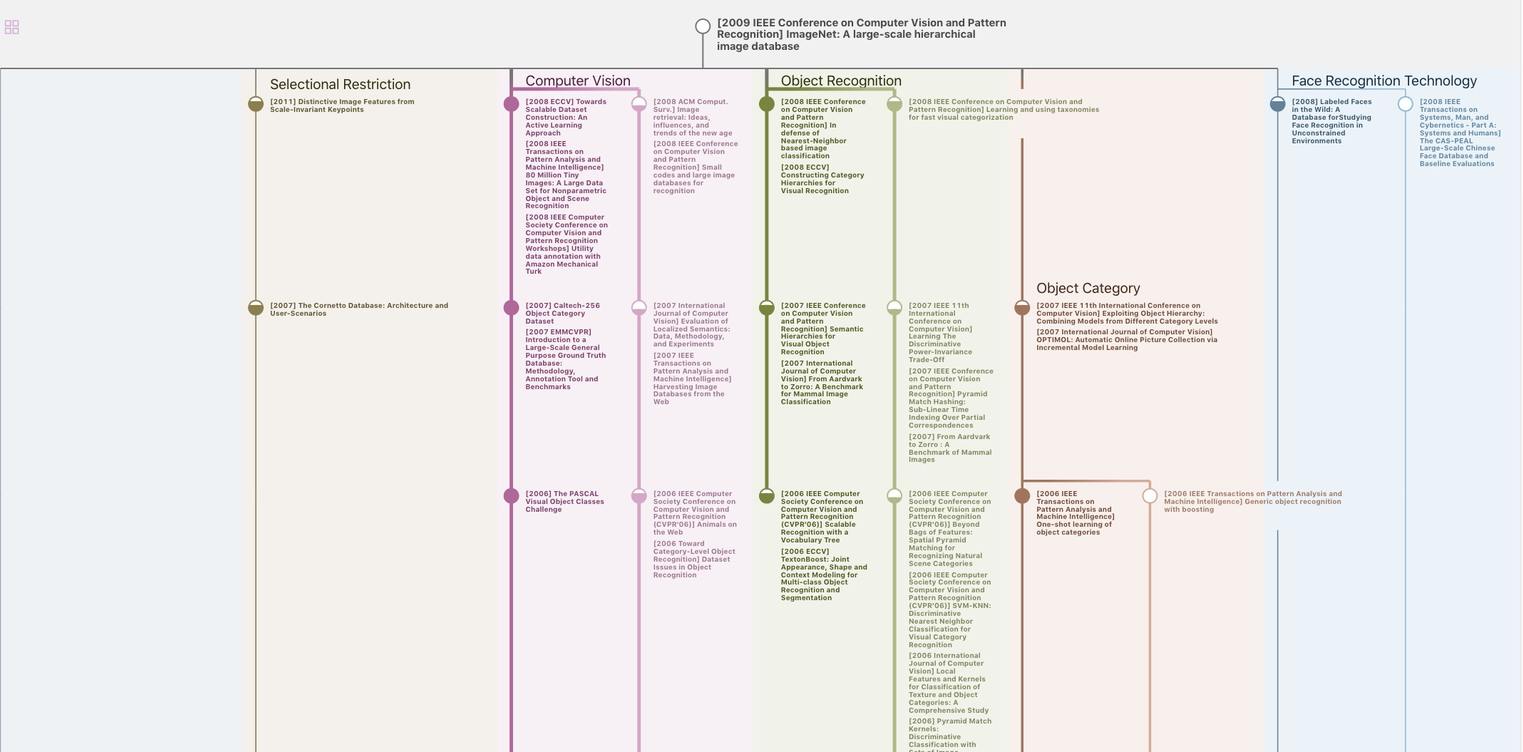
生成溯源树,研究论文发展脉络
Chat Paper
正在生成论文摘要