Efficient Parallel Branch Network With Multi-Scale Feature Fusion For Real-Time Overhead Power Line Segmentation
IEEE SENSORS JOURNAL(2021)
摘要
Image-based segmentation of overhead power lines is critical for power line inspection. Real-time segmentation helps the inspection robot avoid obstacles or land on the wire during the inspection task. It is challenging for several studies to achieve real-time overhead power line segmentation with high accuracy. In addition, cluttered background brings great difficulties to overhead power lines segmentation. To address these issues, an efficient parallel branch network for real-time overhead power line segmentation is proposed. Our framework combines a context branch that generates useful global information with a spatial branch that preserves high-resolution segmentation details. The asymmetric factorized depth-wise bottleneck (AFDB) module is designed in the context branch to achieve more efficient short-range feature extraction and provide a large receptive field. Furthermore, the subnetwork-level skip connections in the classifier are proposed to fuse long-range features and lead to high accuracy. Experiments demonstrate that our framework achieves more than 90% segmentation accuracy.
更多查看译文
关键词
Convolution, Feature extraction, Image segmentation, Inspection, Wires, Sensors, Real-time systems, Real-time segmentation, lightweight network, dilated depth-wise convolution, power line inspection
AI 理解论文
溯源树
样例
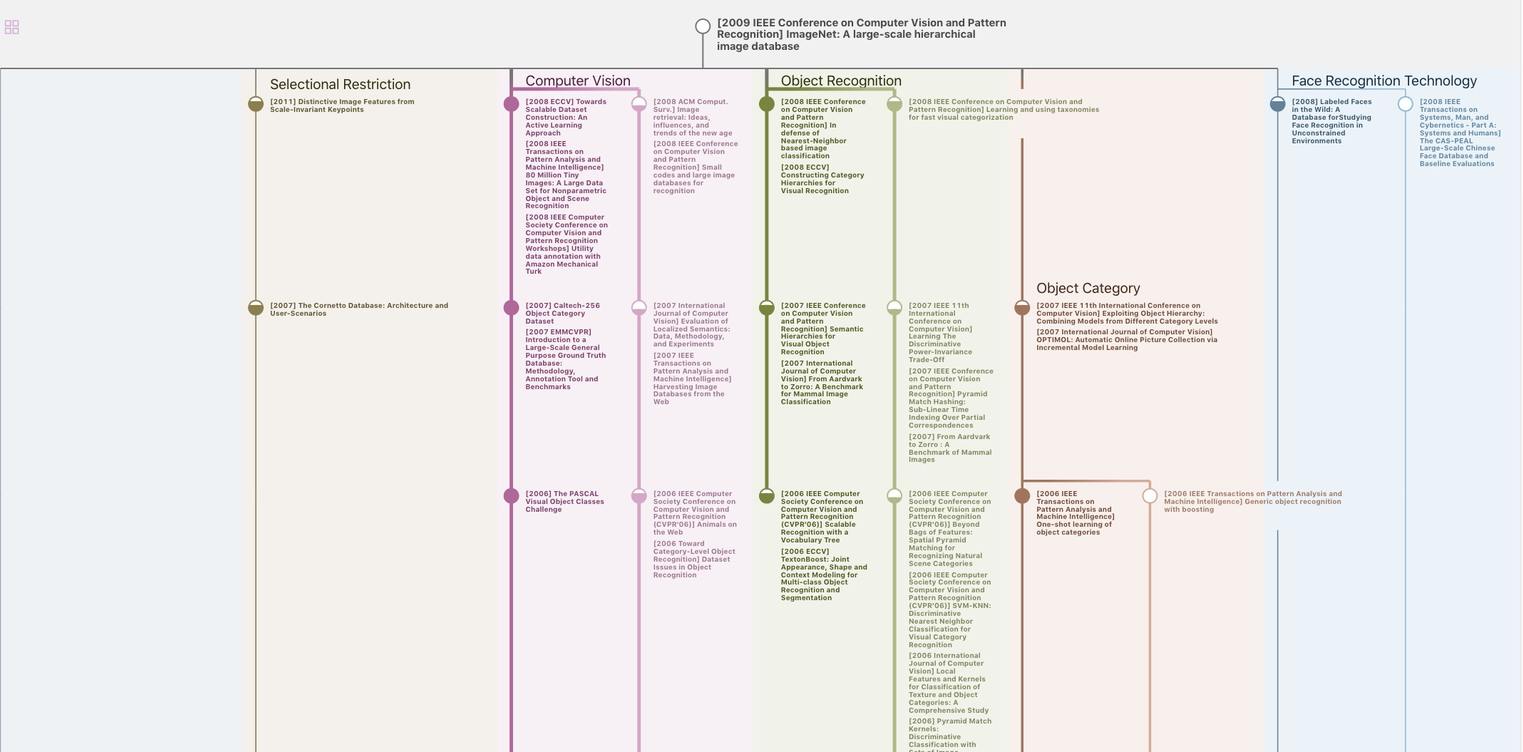
生成溯源树,研究论文发展脉络
Chat Paper
正在生成论文摘要