Statistical learning for the estimation of Judd-Ofelt parameters: A case study of Er3+: Doped tellurite glasses
Journal of Luminescence(2021)
摘要
The active development of optical materials and their corresponding applications requires an in-depth understanding of their optical properties for which Judd-Ofelt (JO) theory is often used. However, calculating the JO parameters (Ω2, Ω4, Ω6) requires extensive experimental and theoretical work, making conventional calculation often inaccessible. In an endeavour to mitigate these difficulties, we have investigated the relationship between the JO parameters and the bulk matrix composition of a suite of Er3+ doped tellurite glasses. The matrix compositions were used to predict the three JO parameters using Support Vector Machine regression, coupled with sparse Principal Component Analysis. Preliminary results demonstrate a strong relationship between the bulk composition and the JO parameters (root-mean-squared error from 0.2053 × 10−20 to 0.5915 × 10−20) and (mean-absolute-error ranging from 0.4038 × 10−20 to 1.2291 × 10−20), suggesting that with further consideration to the data, and additional covariates, we may, in the near future, be able to characterize such materials precisely with no, or minimal experimental work.
更多查看译文
关键词
Statistics,Machine learning,Judd-Ofelt theory,Material science,Erbium
AI 理解论文
溯源树
样例
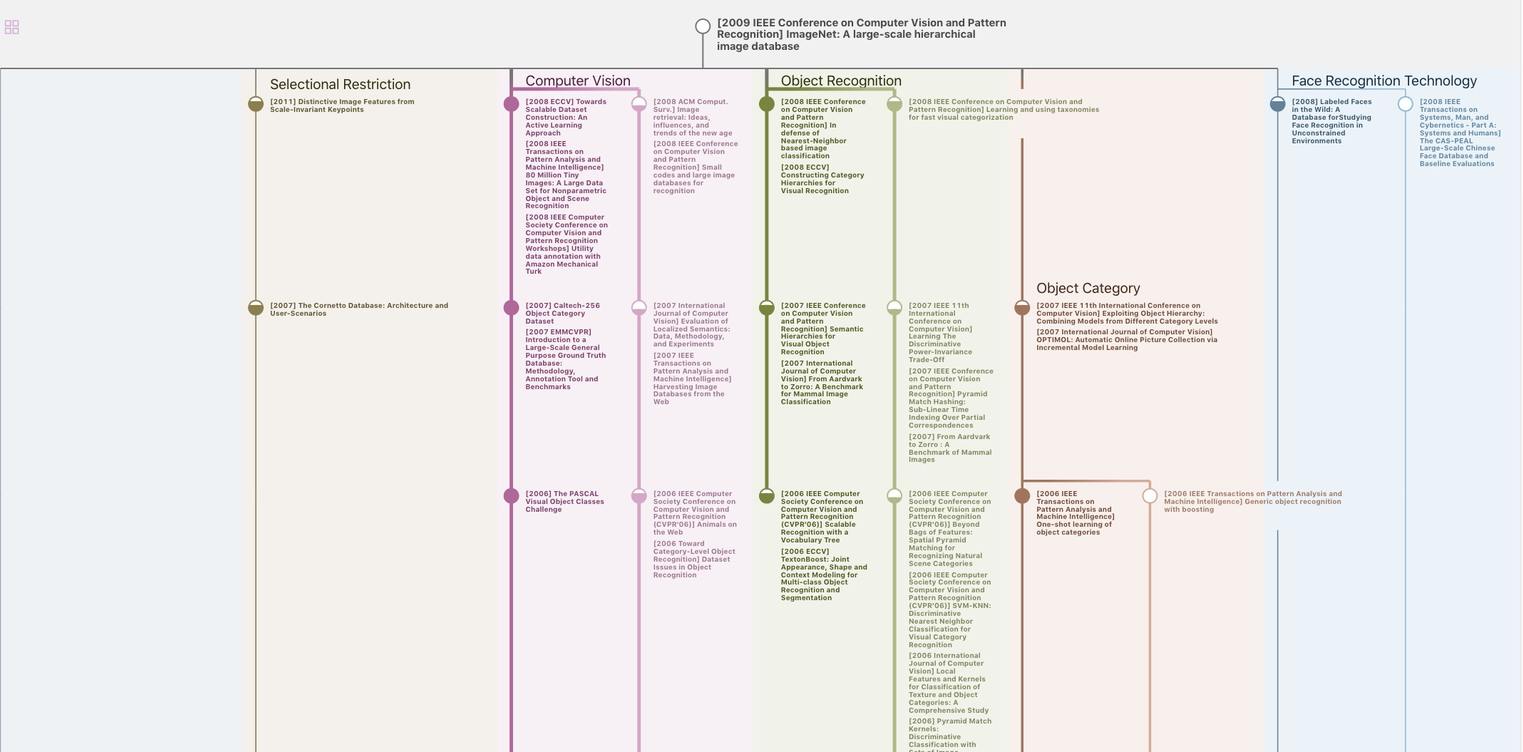
生成溯源树,研究论文发展脉络
Chat Paper
正在生成论文摘要