Evaluation of Rainfall Forecasts by Three Mesoscale Models during the Mei-yu Season of 2008 in Taiwan. Part I: Subjective Comparison
Asia-Pacific Journal of Atmospheric Sciences(2021)
摘要
This study assesses relative merit of three mesoscale models – the Cloud-Resolving Storm Simulator (CReSS), the Central Weather Bureau (CWB) Weather Research and Forecasting (WRF) model, and the CWB Non-hydrostatic Forecast System (NFS) – that participated in the Southwest Monsoon Experiment (SoWMEX) in 2008. The Mei-yu quantitative precipitation forecasts (QPFs) in May–June of the three models are compared against merged data from ~400 rain gauges and the Tropical Rainfall Measuring Mission (TRMM). The three models have a grid size of 3.5 or 5 km, and their QPFs over the forecast range of 12–36 h are verified by subjective means, with particular foci on rainfall spatial pattern, intra-seasonal variability, and diurnal cycle during the entire season, its sub-periods, and Intensive Observation Periods (IOPs). The three models each has advantages and disadvantages. The CReSS performs best in seasonal and diurnal characteristics of rainfall. Despite higher resolution, it suffers from over-forecast and location error problems. Without a data assimilation system, its single domain with a limited size fabricates it more sensitive to errors in initial and boundary conditions (IC/BCs) from the global model. Although the CWB WRF is most stable among three and performs well in simulating spatial distribution of rainfall, it exhibits under-prediction with an inadequate intra-seasonal variation. In both the entire period and IOPs, the CWB NFS simulates rainfall pattern closest to observation with only slight under-prediction. However, the diurnal cycle captured by the CWB NFS has a phase lag of 12 h and thus a timing error that is likely associated with delayed development of convection due to systematic bias.
更多查看译文
关键词
SoWMEX, CReSS, CWB WRF, CWB NFS, QPF, IOP
AI 理解论文
溯源树
样例
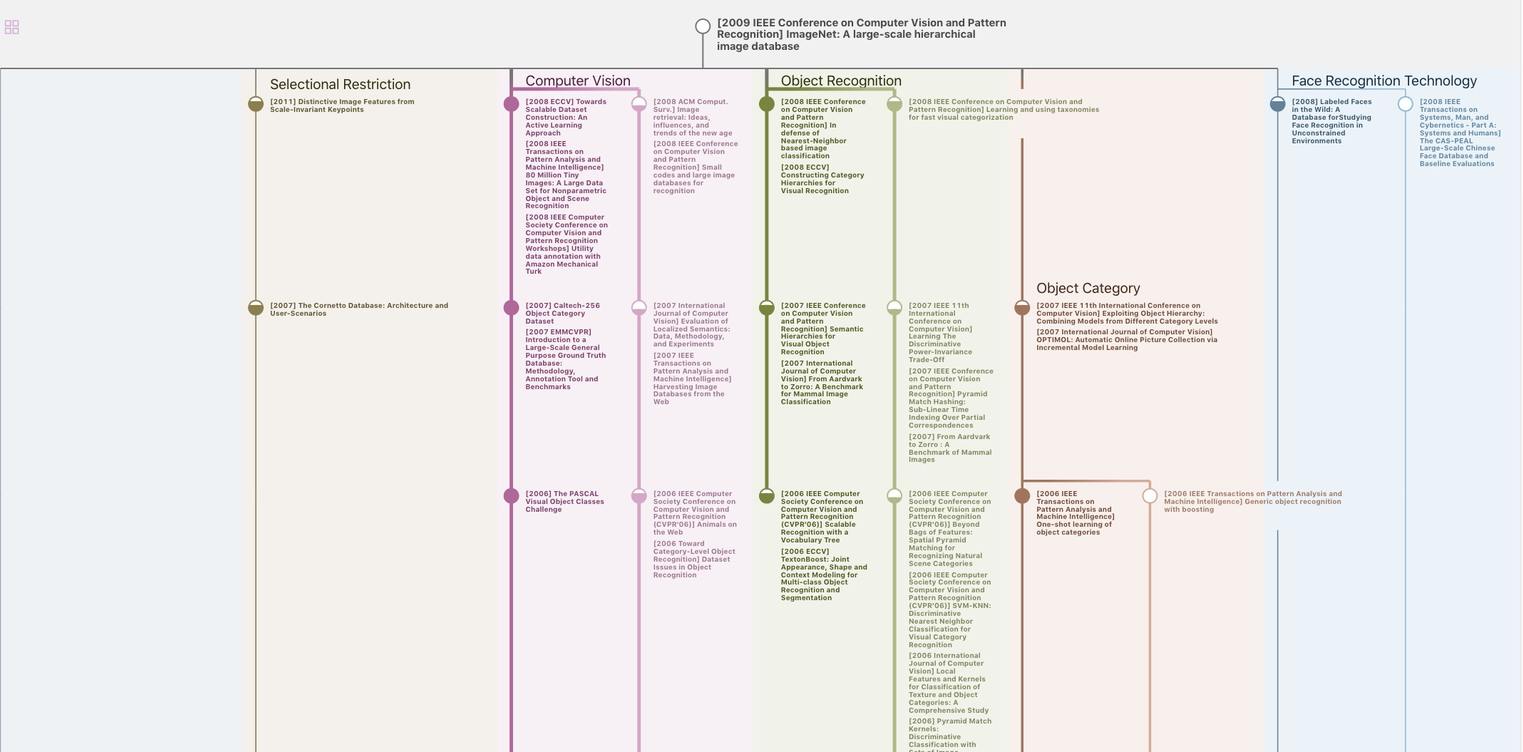
生成溯源树,研究论文发展脉络
Chat Paper
正在生成论文摘要