Spatio-Temporal K-Nn Prediction Of Traffic State Based On Statistical Features In Neighbouring Roads
JOURNAL OF INTELLIGENT & FUZZY SYSTEMS(2021)
摘要
Traffic congestion on a road results in a ripple effect to other neighbouring roads. Previous research revealed existence of spatial correlation on neighbouring roads. Similar traffic patterns with regards to day and time can be seen amongst roads in a neighbouring area. Presently, nonlinear models of neural network are applied on historical data to predict traffic congestion. Even though neural network has successfully modelled complex relationships, more time is needed to train the network. A non-parametric approach, the k-nearest neighbour (K-NN) is another method for forecasting traffic condition which can capture the nonlinear characteristics of traffic flow. An earlier study has been done to predict traffic flow using K-NN based on connected roads (both downstream and upstream). However, impact of road congestion is not only to connected roads, but also to roads surrounding it. Surrounding roads that are impacted by road congestion are those having 'high relationship' with neighbouring roads. Thus, this study aims to predict traffic state using K-NN by determining high relationship roads within neighbouring roads. We determine the highest relationship neighbouring roads by clustering the surrounding roads by combining grey level co-occurrence matrix (GLCM) with k-means. Our experiments showed that prediction of traffic state using K-NN based on high relationship roads using both GLCM and k-means produced better accuracy than using k-means only.
更多查看译文
关键词
Classification algorithm, clustering algorithm, machine learning algorithm, nearest neighbour search, intelligent transportation system
AI 理解论文
溯源树
样例
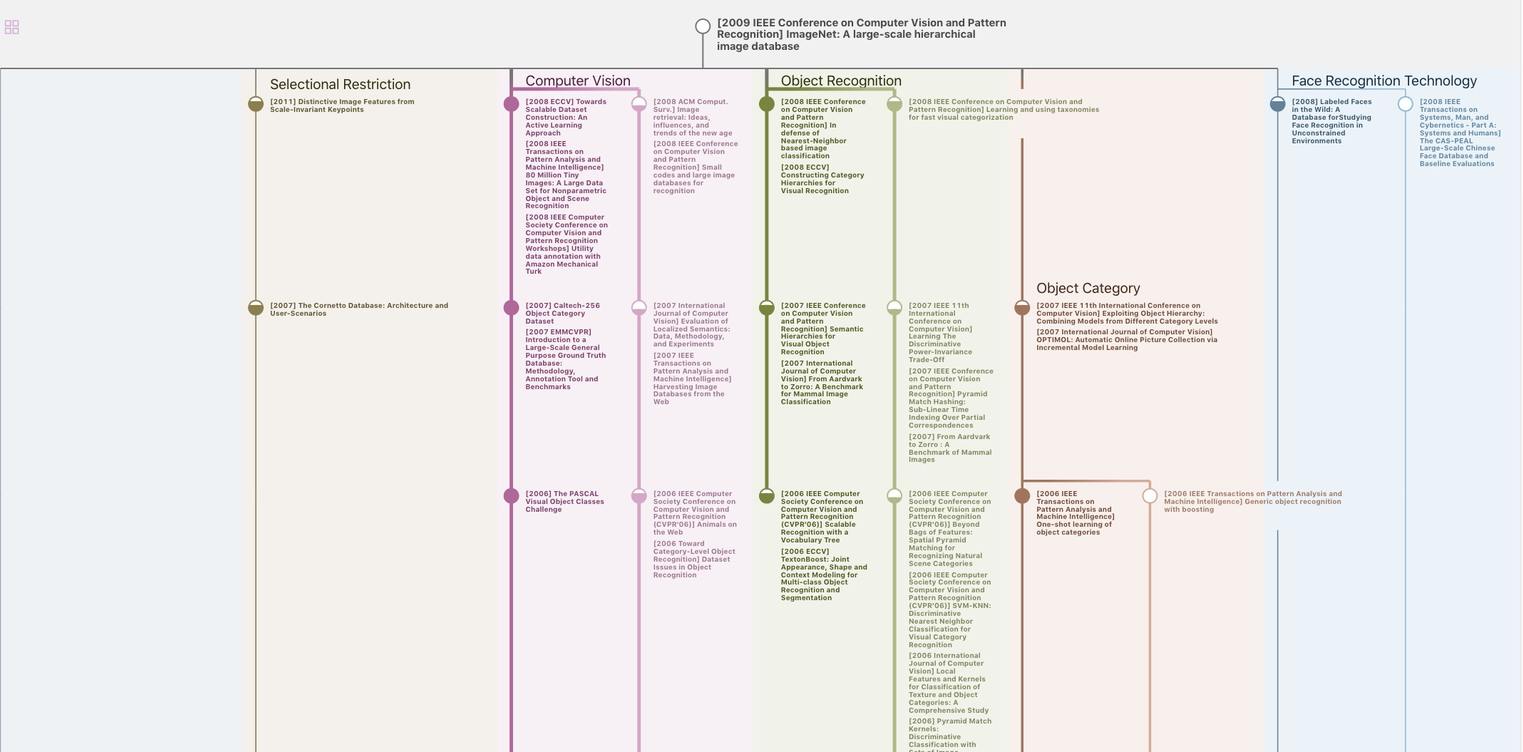
生成溯源树,研究论文发展脉络
Chat Paper
正在生成论文摘要