Adaptive Drift Detection Mechanism For Non-Stationary Data Stream
JOURNAL OF INFORMATION & KNOWLEDGE MANAGEMENT(2021)
摘要
Mining is a challenging and important task in a non-stationary data stream. It is used in financial sectors, web log analysis, sensor networks, network traffic management, etc. In this environment, data distribution may change overtime and is called concept drift. So, it is necessary to identify the changes and address them to keep the model relevant to the incoming data. Many researchers have used Drift Detection Method (DDM). However, DDM is very sensitive to detect gradual drift where the detection delay is high. In this paper, we propose Adaptive Drift Detection Method (ADDM) which improves the performance of the drift detection mechanism. The ADDM uses a new parameter to detect the gradual drift in order to reduce the detection delay. The proposed method, ADDM, experiments with six synthetic datasets and four real-world datasets. Experimental results confirm that ADDM reduces the drift detection delay and false-positive rate (FPR) while preserving high classification accuracy.
更多查看译文
关键词
Concept drift, drift detection method, data stream, Artificial Bee Colony
AI 理解论文
溯源树
样例
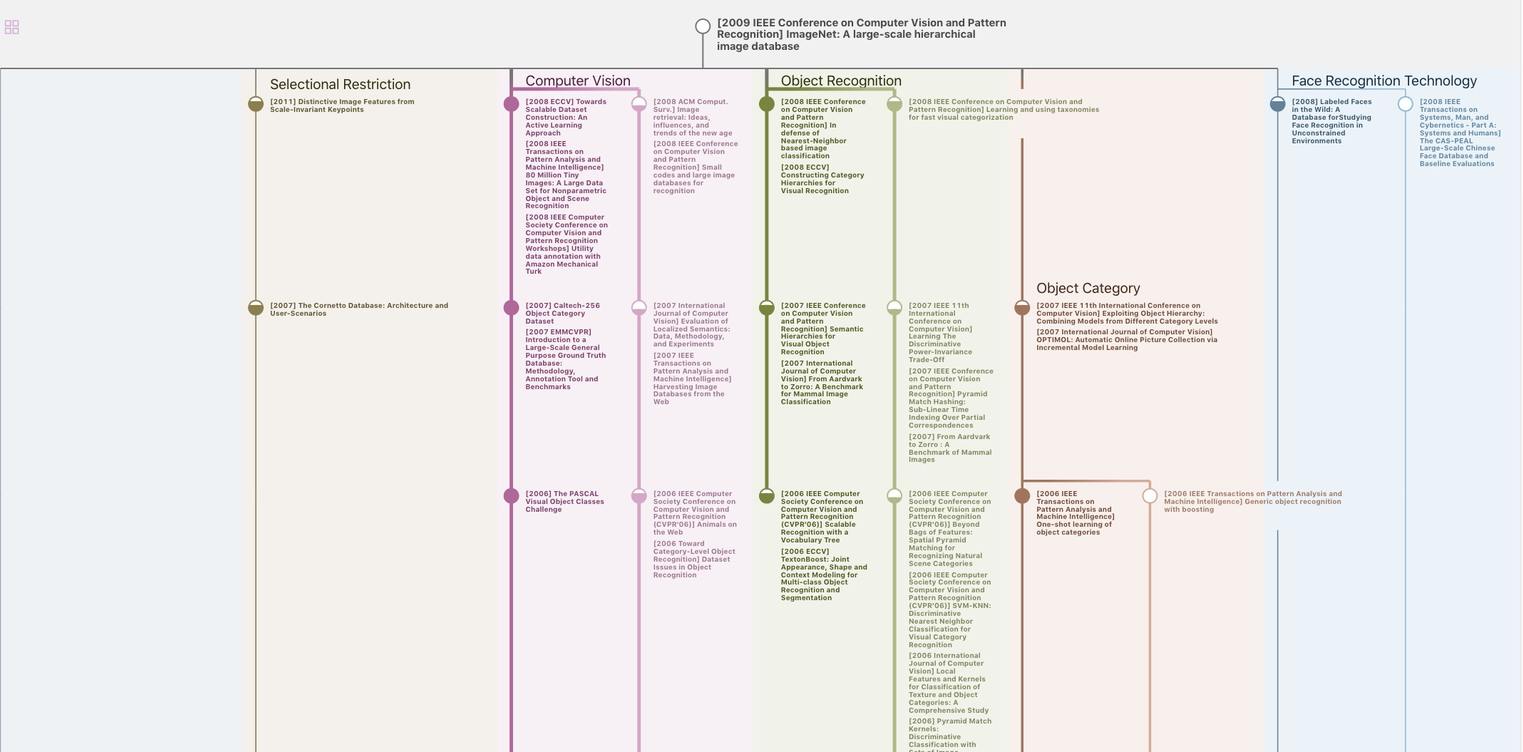
生成溯源树,研究论文发展脉络
Chat Paper
正在生成论文摘要