Enhancing Trajectory Prediction Using Sparse Outputs: Application to Team Sports
Neural computing & applications(2021)
摘要
Sophisticated trajectory prediction models that effectively mimic team dynamics have many potential uses for sports coaches, broadcasters and spectators. However, through experiments on soccer data we found that it can be surprisingly challenging to train a deep learning model for player trajectory prediction which outperforms linear extrapolation on average distance between predicted and true future trajectories. We propose and test a novel method for improving training by predicting a sparse trajectory and interpolating using constant acceleration, which improves performance for several models. This interpolation can also be used on models that are not trained with sparse outputs, and we find that this consistently improves performance for all tested models. Additionally, we find that the accuracy of predicted trajectories for a subset of players can be improved by conditioning on the full trajectories of the other players, and that this is further improved when combined with sparse predictions. We also propose a novel architecture using graph networks and multi-head attention (GraN–MA) which achieves better performance than other tested state-of-the-art models on our dataset and is trivially adapted for both sparse trajectories and full-trajectory conditioned trajectory prediction.
更多查看译文
关键词
Deep learning,Trajectory prediction,Multi-agent trajectory prediction,Sparse predictions,Motion model
AI 理解论文
溯源树
样例
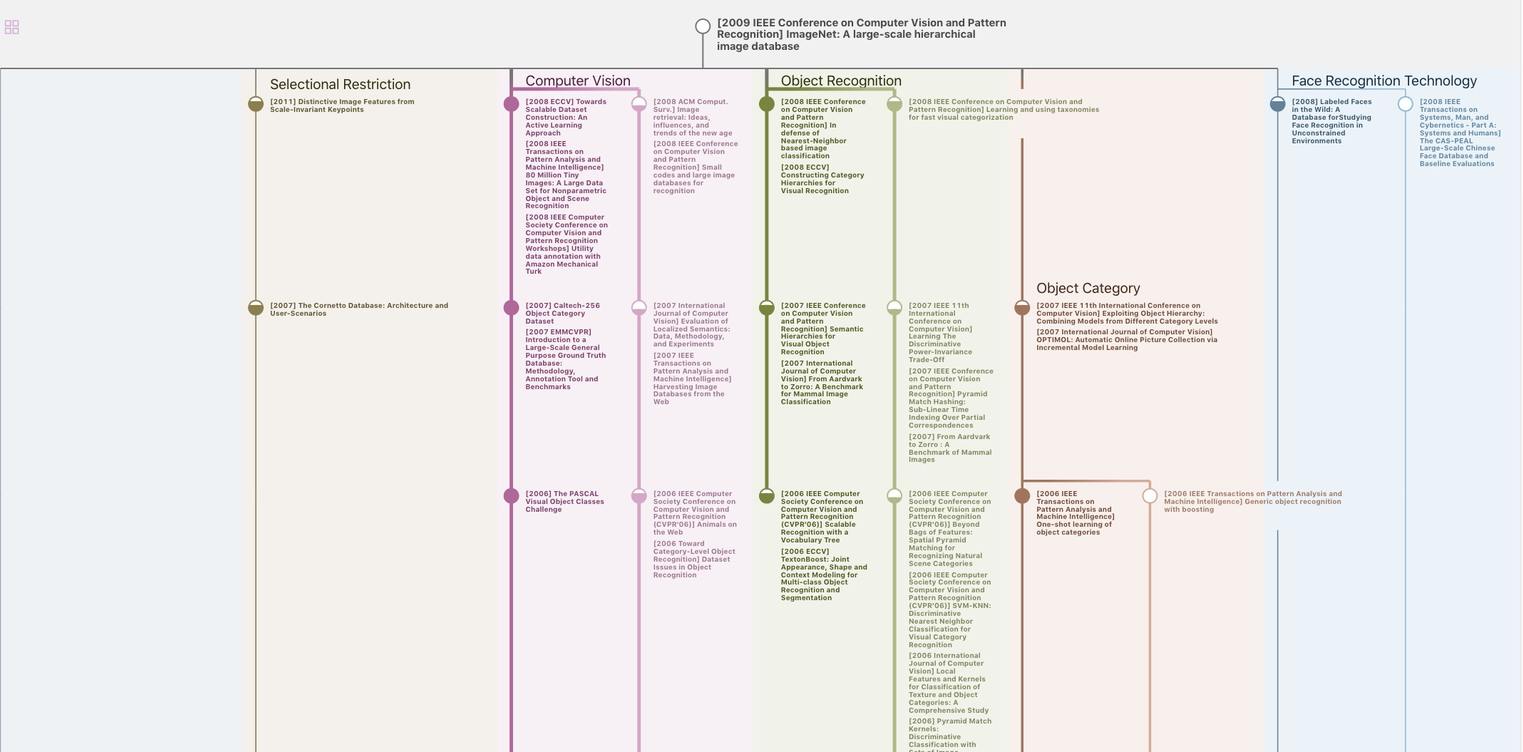
生成溯源树,研究论文发展脉络
Chat Paper
正在生成论文摘要