Fault-Tolerant Control Of Programmable Logic Controller-Based Production Systems With Deep Reinforcement Learning
JOURNAL OF MECHANICAL DESIGN(2021)
摘要
Fault-tolerant control policies that automatically restart programable logic controller-based automated production system during fault recovery can increase system availability. This article provides a proof of concept that such policies can be synthesized with deep reinforcement learning. The authors specifically focus on systems with multiple end-effectors that are actuated in only one or two axes, commonly used for assembly and logistics tasks. Due to the large number of actuators in multi-end-effector systems and the limited possibilities to track workpieces in a single coordinate system, these systems are especially challenging to learn. This article demonstrates that a hierarchical multi-agent deep reinforcement learning approach together with a separate coordinate prediction module per agent can overcome these challenges. The evaluation of the suggested approach on the simulation of a small laboratory demonstrator shows that it is capable of restarting the system and completing open tasks as part of fault recovery.
更多查看译文
关键词
agent-based design, artificial intelligence, design automation, machine learning, robotic systems
AI 理解论文
溯源树
样例
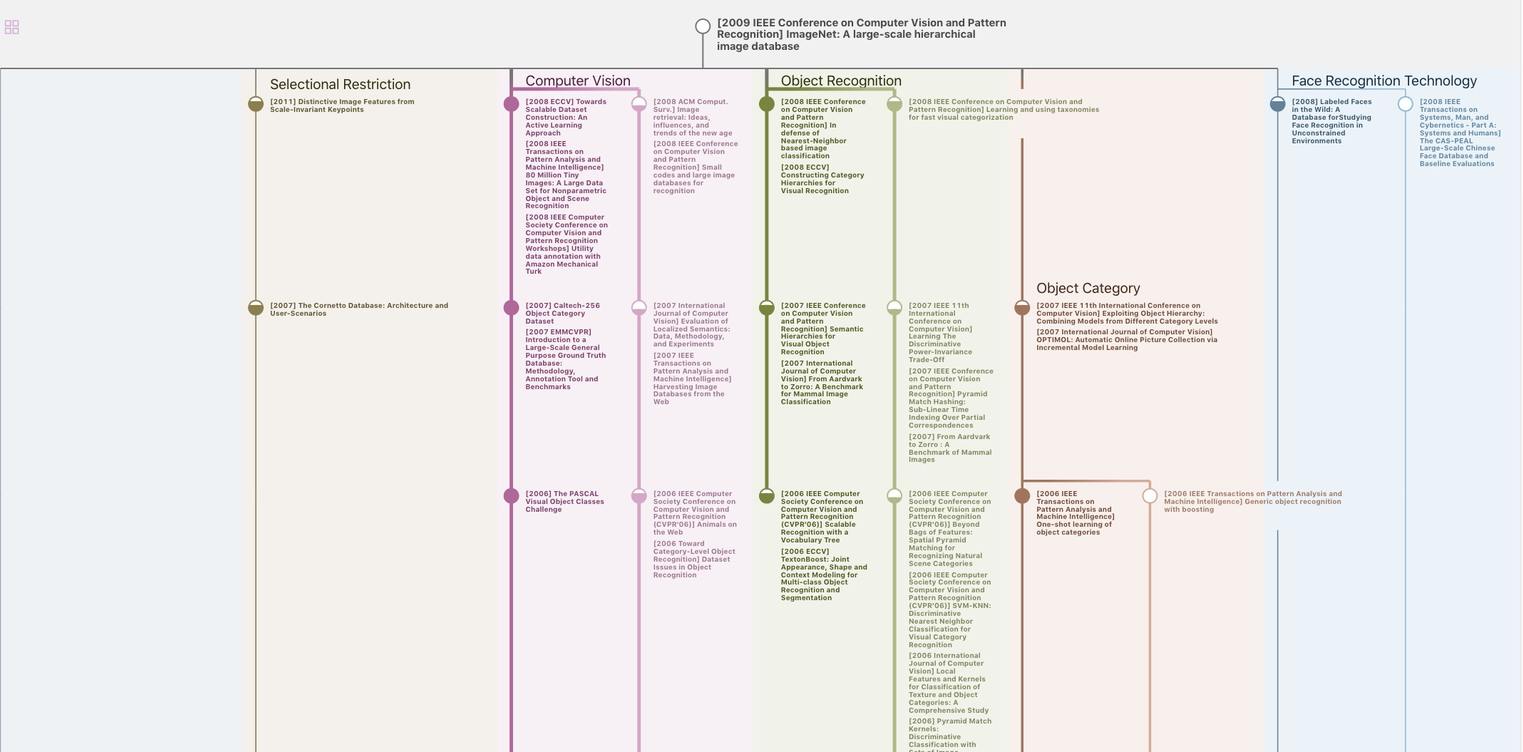
生成溯源树,研究论文发展脉络
Chat Paper
正在生成论文摘要