Learning From Mistakes: Improving Spelling Correction Performance With Automatic Generation Of Realistic Misspellings
EXPERT SYSTEMS(2021)
摘要
Sequence to sequence models (seq2seq) require a large amount of labelled training data to learn the mapping between the input and output. A large set of misspelled words together with their corrections is needed to train a seq2seq spelling correction system. Low-resource languages such as Turkish usually lack such large annotated datasets. Although misspelling-reference pairs can be synthesized with a random procedure, the generated dataset may not well match to genuine human-made misspellings. This might degrade the performance in realistic test scenarios. In this paper, we propose a novel procedure to automatically introduce human-like misspellings to legitimate words in Turkish language. Generated human-like misspellings are used to improve the performance of a seq2seq spelling correction system. The proposed system consists of two separate models; a misspelling generator and a spelling corrector. The generator is trained using a relatively small number of human-made misspellings and their manual corrections. Reference words and their misspellings are used as inputs and outputs of the generator, respectively. As a result, it is trained to add realistic spelling errors to the valid words. Training data of the spelling corrector is augmented by the generator's human-like misspellings. In the experiments, we observe that the data augmentation significantly improves the spelling correction performance. Our proposed method yields 5% absolute improvement over the state-of-the-art Turkish spelling correction systems in a test set which contains human-made misspellings from Twitter messages.
更多查看译文
关键词
deep learning, machine learning, natural language processing, neural network
AI 理解论文
溯源树
样例
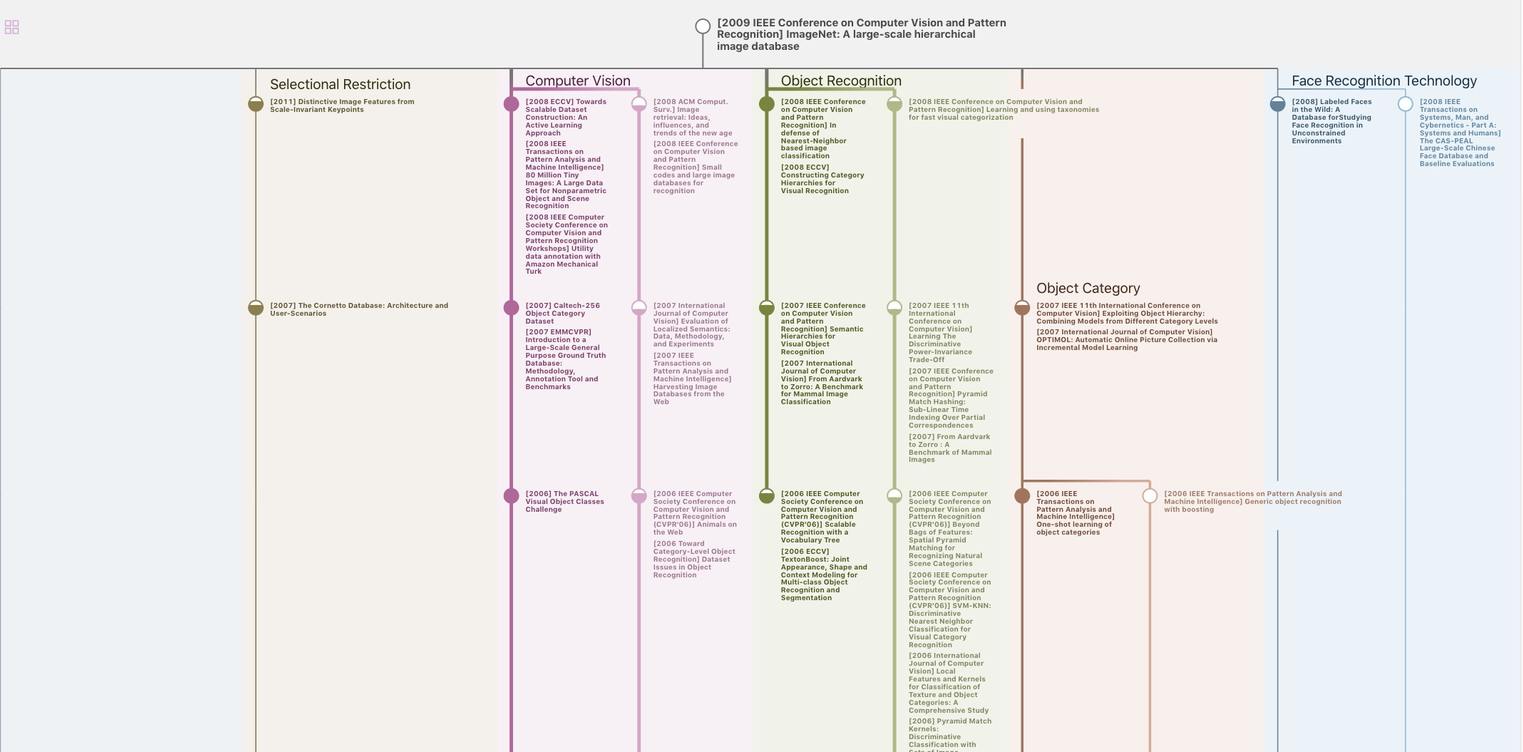
生成溯源树,研究论文发展脉络
Chat Paper
正在生成论文摘要