Noninvasive Detection Of Appliance Utilization Patterns In Residential Electricity Demand
ENERGIES(2021)
摘要
Smart meters with automatic meter reading functionalities are becoming popular across the world. As a result, load measurements at various sampling frequencies are now available. Several methods have been proposed to infer device usage characteristics from household load measurements. However, many techniques are based on highly intensive computations that incur heavy computational costs; moreover, they often rely on private household information. In this paper, we propose a technique for the detection of appliance utilization patterns using low-computational-cost algorithms that do not require any information about households. Appliance utilization patterns are identified only from the system status behavior, represented by large system status datasets, by using dimensionality reduction and clustering algorithms. Principal component analysis, k-means, and the elbow method are used to define the clusters, and the minimum spanning tree is used to visualize the results that show the appearance of utilization patterns. Self organizing maps are used to create a system status classifier. We applied our techniques to two public datasets from two different countries, the United Kingdom (UK-DALE) and the US (REDD), with different usage patterns. The proposed clustering techniques enable effective demand-side management, while the system status classifier can detect appliance malfunctions only through system status analyses.
更多查看译文
关键词
usage pattern recognition, data mining, dimensionality reduction, k-means, principal component analysis, self organizing maps, classifiers
AI 理解论文
溯源树
样例
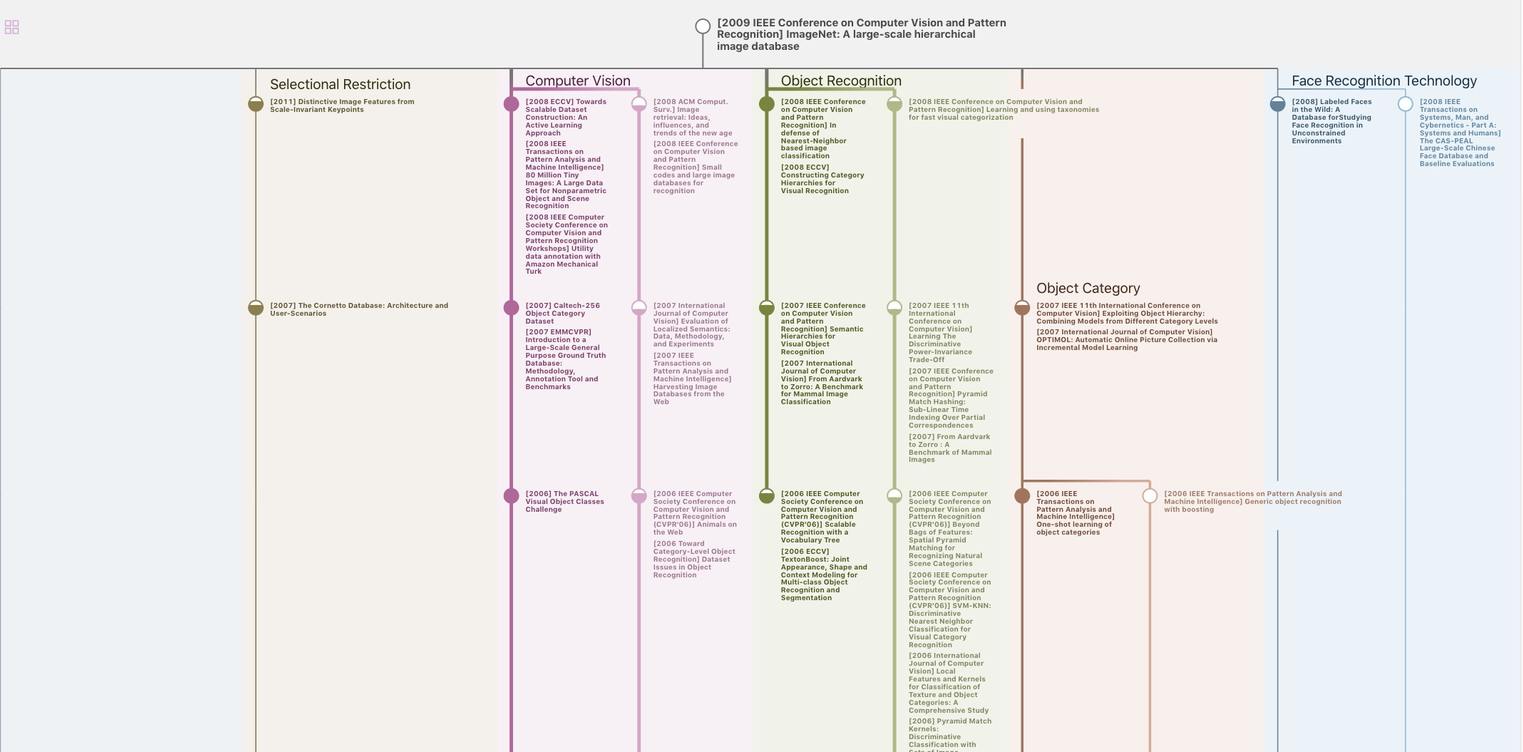
生成溯源树,研究论文发展脉络
Chat Paper
正在生成论文摘要