Fully Memristive SNNs with Temporal Coding for Fast and Low-power Edge Computing
International Electron Devices Meeting(2020)
摘要
SNNs with temporal coding (TC), inspired by the human visual system, have a powerful ability to enable fast and low-power neuromorphic computing. Memristive devices show excellent performance on emulating spiking neurons and synapses in hardware. However, the neuron circuits used for implementing a fully memristive TC SNN are absent. In this work, for the first time, we demonstrate a LIF neuron based on a NbO x device to meet the requirements for the hardware implementation of TC SNNs. The neuron fires at most one spike within an inference window, and its spiking latency inverse to the input current intensity. Using such a neuron, we further experimentally demonstrated a fully memristive TC SNN (256 × 5) to recognize the Olivetti face patterns. Attributing to the one-spike scheme, the TC SNN achieves a sparser spiking number (~ 72 × reductions), faster inference speed (> 1.5 × improvement), lower power (~ 53 × reductions) than what happens in rate-coding SNNs.
更多查看译文
关键词
memristive SNN,temporal coding,human visual system,low-power neuromorphic computing,memristive devices,spiking neurons,neuron circuits,fully memristive TC SNN,LIF neuron,hardware implementation,spiking latency inverse,one-spike scheme,sparser spiking number,rate-coding SNN
AI 理解论文
溯源树
样例
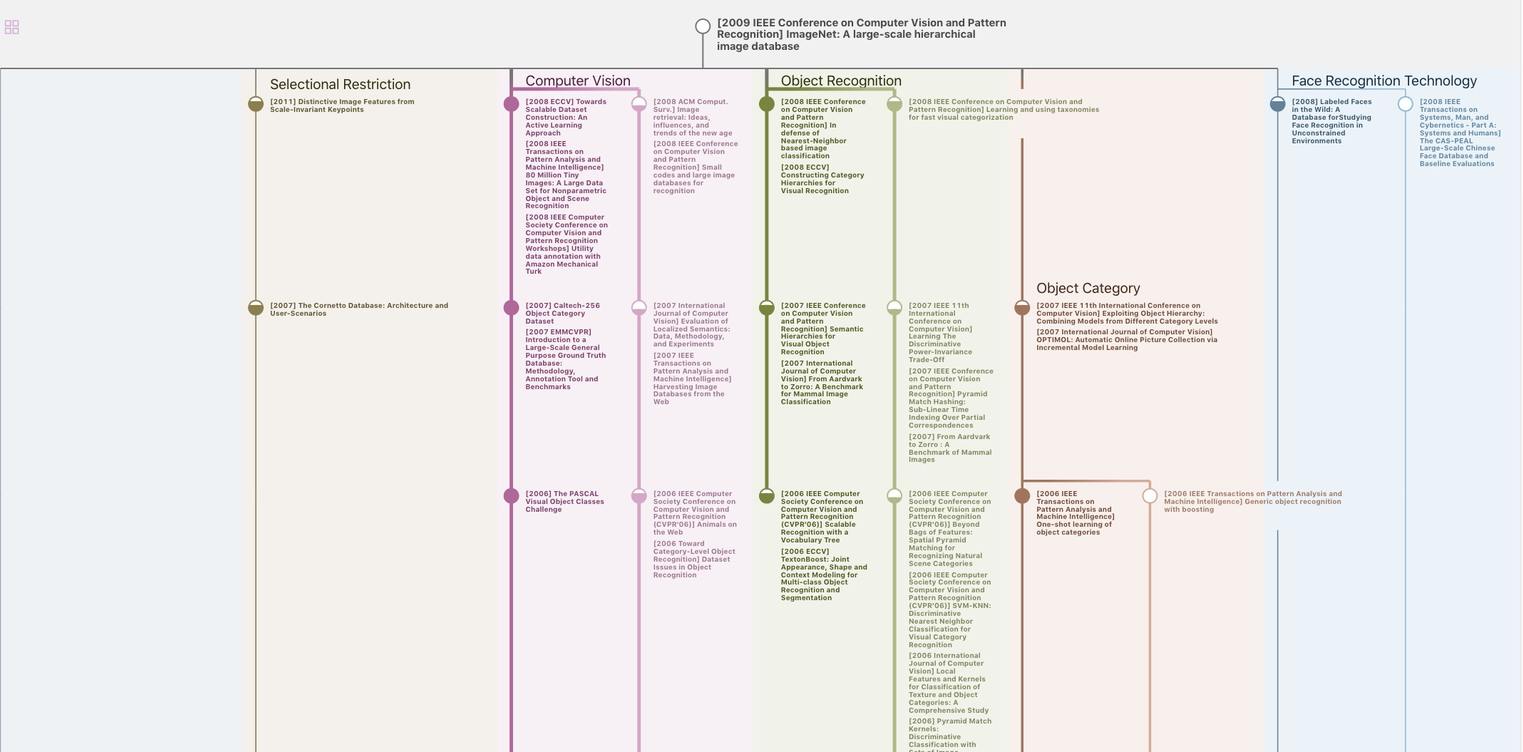
生成溯源树,研究论文发展脉络
Chat Paper
正在生成论文摘要