Machine Learning To Classify Suicidal Thoughts And Behaviors: Implementation Within The Common Data Elements Used By The Military Suicide Research Consortium
CLINICAL PSYCHOLOGICAL SCIENCE(2021)
摘要
Suicide rates among military-connected populations have increased over the past 15 years. Meta-analytic studies indicate prediction of suicide outcomes is lacking. Machine-learning approaches have been promoted to enhance classification models for suicide-related outcomes. In the present study, we compared the performance of three primary machine-learning approaches (i.e., elastic net, random forests, stacked ensembles) and a traditional statistical approach, generalized linear modeling (i.e., logistic regression), to classify suicide thoughts and behaviors using data from the Military Suicide Research Consortium's Common Data Elements (CDE; n = 5,977-6,058 across outcomes). Models were informed by (a) selected items from the CDE or (b) factor scores based on exploratory and confirmatory factor analyses on the selected CDE, items. Results indicated similar classification performance across models and sets of features. In this study, we suggest the need for robust evidence before adopting more complex classification models and identify measures that are particularly relevant in classifying suicide-related outcomes.
更多查看译文
关键词
classification, machine learning, statistical analysis, suicide prevention
AI 理解论文
溯源树
样例
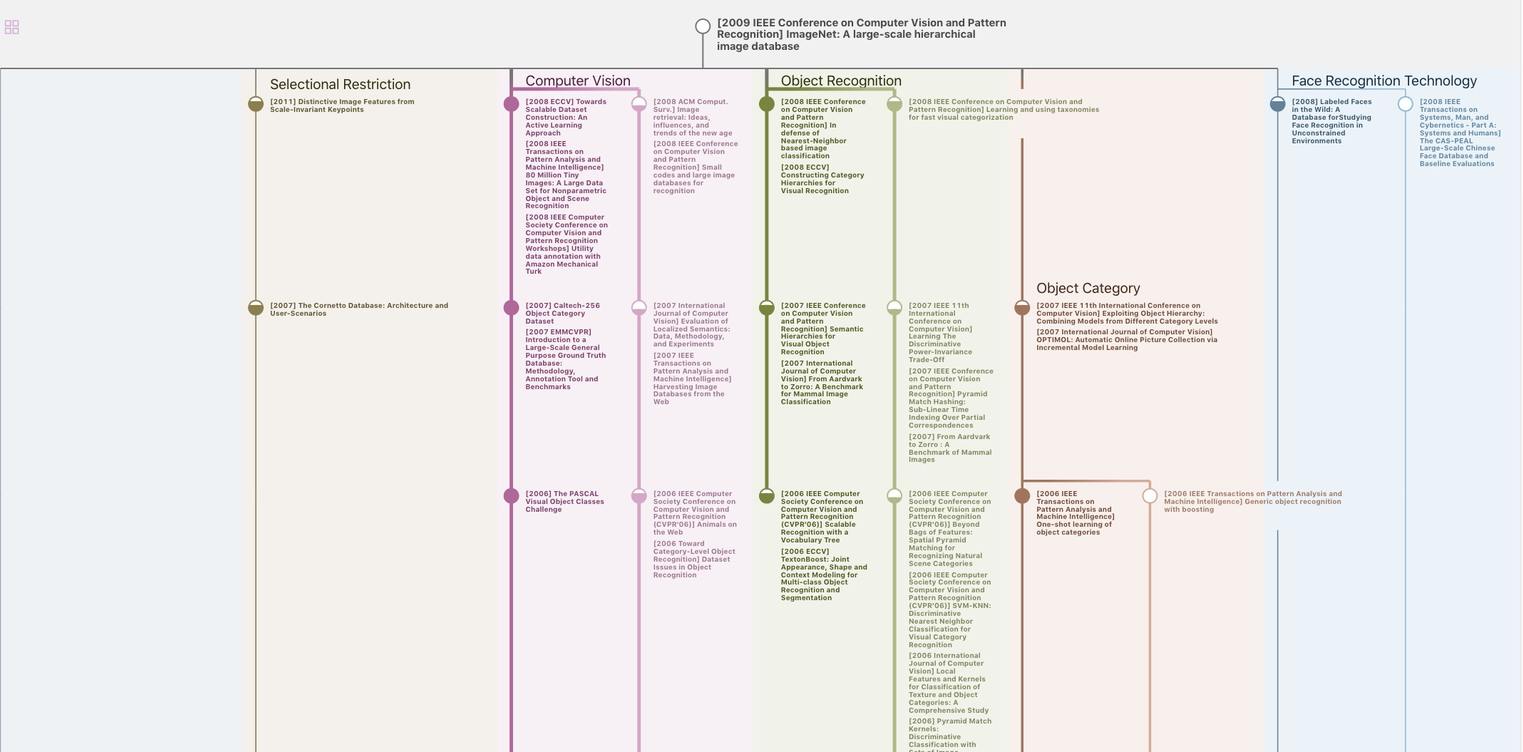
生成溯源树,研究论文发展脉络
Chat Paper
正在生成论文摘要