Multiclass Classification of Astronomical Objects in the Galaxy M81 using Machine Learning Techniques
2020 24th International Computer Science and Engineering Conference (ICSEC)(2020)
摘要
Data in astronomy usually contain various classes of astronomical objects. In this study, we explore the application of multiclass classification in classifying astronomical objects in the galaxy MS1. Our objective is to specify machine learning techniques that are best suited to our data and our classification goal. We used the archival data retrieved from the Canada-France-Hawaii Telescope (CFHT) data archive. The imaging data were transformed into data tables, then classified based on their visual appearances into five classes, including star, globular cluster, rounded galaxy, elongated galaxy, and fuzzy object. The classified data were used for supervised machine learning model building and testing. We investigated seven classification techniques, including Random Forest, Multilayer Perceptron, Weightless neural network (WiSARD), Deep learning (Weka deep learning), Logistic Regression, Support Vector Machine (SVM), and Multiclass Classifier. Our experiments show that Random Forest and Multilayer Perceptron archived the highest overall performances and are the best-suited model for classifying astronomical objects in the CFHT data of the galaxy M81.
更多查看译文
关键词
Multiclass Classification,Astronomy,Machine Learning,Random Forest,Multilayer Perceptron,Weightless Neural Network
AI 理解论文
溯源树
样例
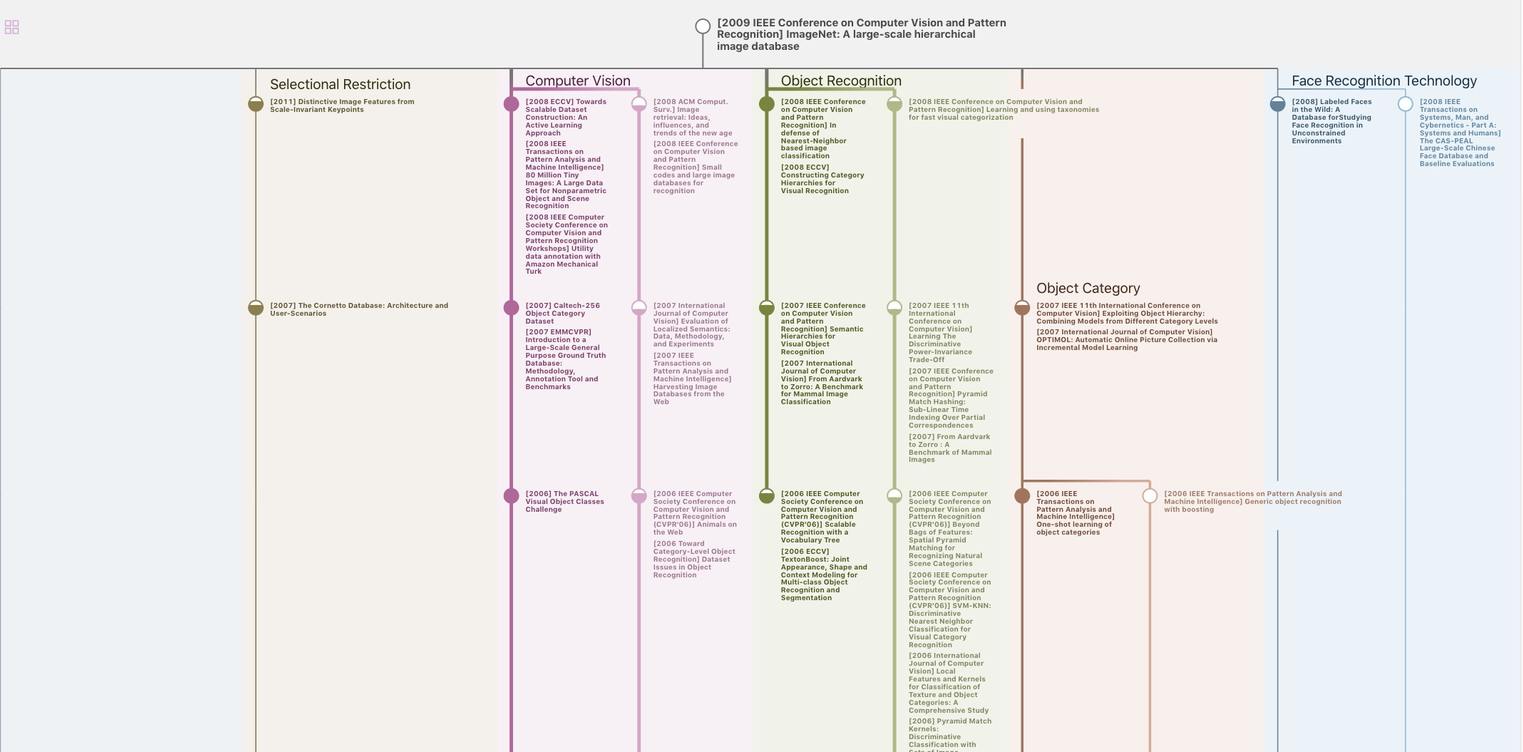
生成溯源树,研究论文发展脉络
Chat Paper
正在生成论文摘要