A novel robust fixed-time convergent zeroing neural network for solving time-varying noise-polluted nonlinear equations
INTERNATIONAL JOURNAL OF COMPUTER MATHEMATICS(2021)
摘要
Solving nonlinear equations is a crucial step in the territories of science and engineering, as many practical problems could be mathematically described by nonlinear equations. In this paper, a novel robust fast convergence zeroing neural network (RFCZNN) by utilizing a reconstructed activation function (AF) is presented and investigated for the dynamic nonlinear equations (DNE) solving problems in predictable period. Comparing with recently reported finite-time nonlinear recurrent neural network, the presented RFCZNN solves the DNE in settled theoretical time and possesses better robustness in noise-polluted environments. Unlike the finite-time convergent neural network models, the time consumption of the presented RFCZNN in convergence process can be calculated directly by mathematics without considering modelling initial states. The comparative experimental results for solving high-order (second and third order) DNE and tracking robotic motional trail are presented separately to further represent that the proposed fixed-time convergent RFCZNN model is more robust and efficient.
更多查看译文
关键词
Nonlinear equation (NE), zeroing neural network (ZNN), robust fast convergence ZNN (RFCZNN), activation function (AF), fixed-time convergence
AI 理解论文
溯源树
样例
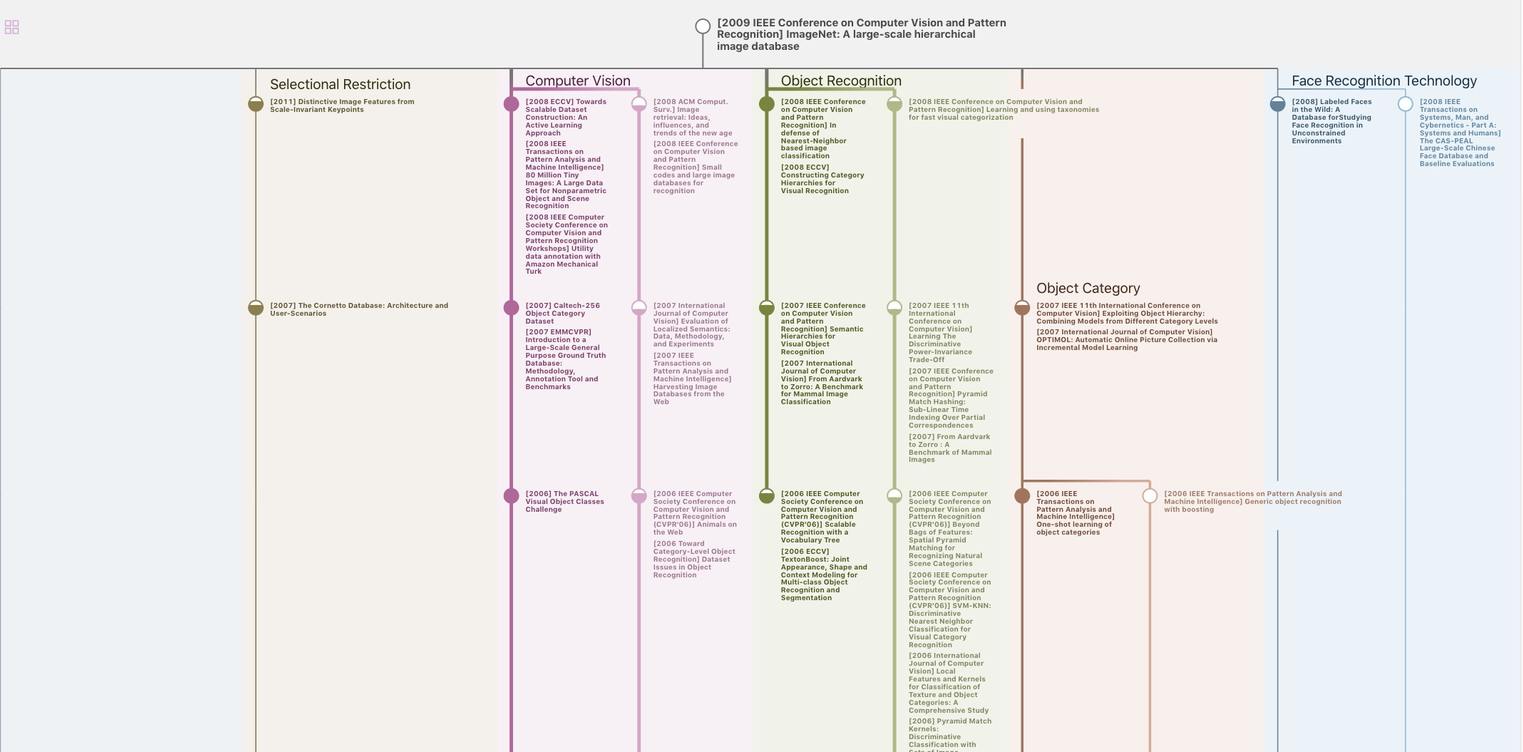
生成溯源树,研究论文发展脉络
Chat Paper
正在生成论文摘要