Combined i-Vector and Extreme Learning Machine Approach for Robust Speaker Identification and Evaluation with SITW 2016, NIST 2008, TIMIT Databases
Circuits, Systems, and Signal Processing(2021)
摘要
In this article, a novel combined i-vector and an Extreme Learning Machine (ELM) is proposed for speaker identification. The ELM is chosen because it is fast to train and has a universal approximator property. Four combinations of features based on Mel Frequency Cepstral Coefficient and Power Normalized Cepstral Coefficient are used. Besides, seven fusion methods are exploited. The system is evaluated with three different databases, namely: the SITW 2006, NIST 2008, and the TIMIT database. This work employs the 2016 SITW database for the first time for speaker identification using the integration between the ELM and i-vector approach. From each database, 120 speakers with 1200 speech utterances are used (overall 360 speakers with 3600 speech utterances). Furthermore, comprehensive evaluations are exploited with a wide range of realistic background noise types (Stationary noise AWGN and Non-Stationary Noise types) with the handset effect. The proposed system is compared with the Gaussian Mixture Model-Universal Background Model (GMM-UBM) and other states of the art approaches. The results show that the i-vector method outperforms the GMM-UBM approach and other state- of-the-art methods under specific conditions, and that fusion techniques can be used to improve robustness to noise and handset effects.
更多查看译文
关键词
Text independent speaker identification, Non-stationary Noise, Additive Gaussian noise, TIMIT database
AI 理解论文
溯源树
样例
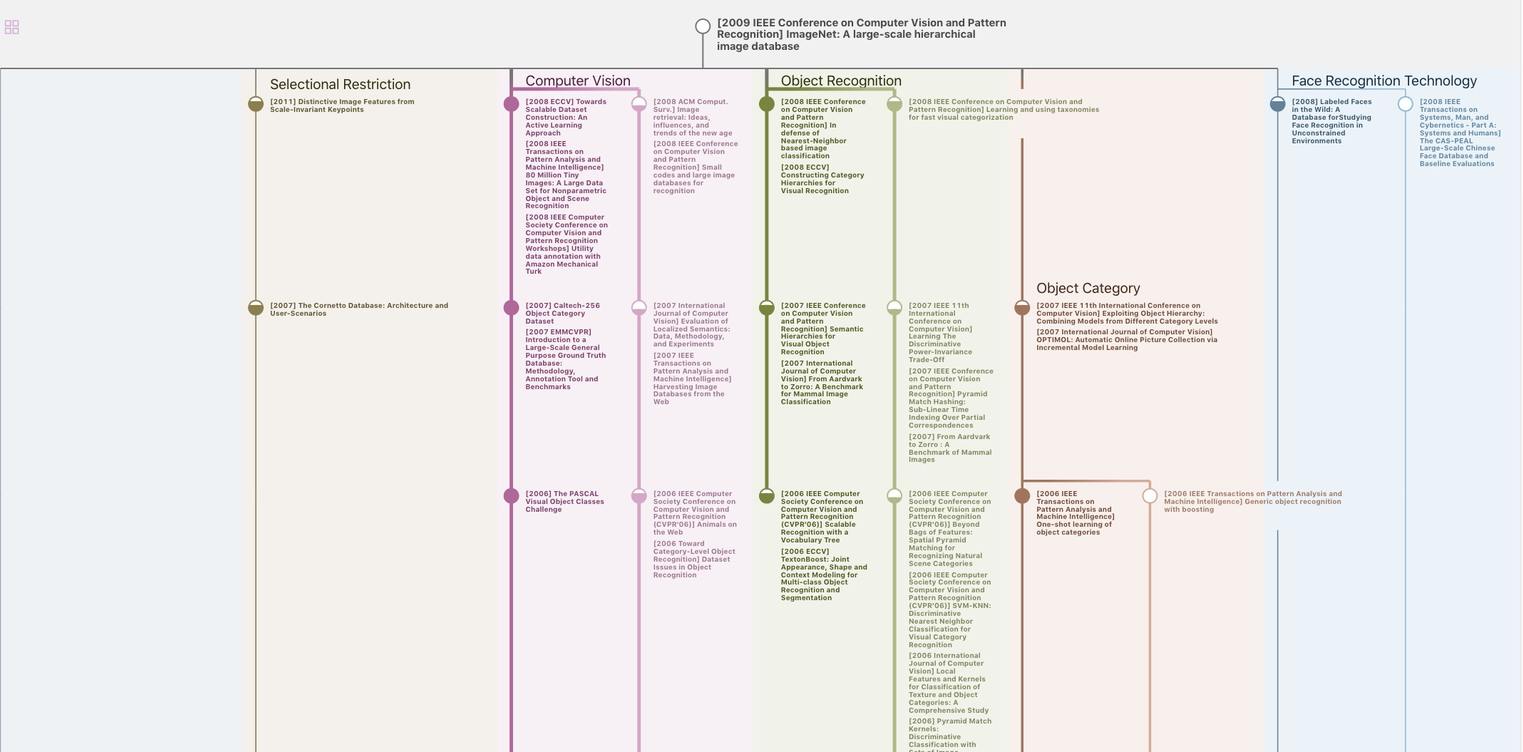
生成溯源树,研究论文发展脉络
Chat Paper
正在生成论文摘要