Abstract 10: Radiomic Signature of the White Matter Hyperintensity Burden Correlates with Clinical Phenotypes
Stroke(2021)
摘要
Introduction: Structural integrity of cerebral parenchyma is an essential radiographic equivalent of brain health; but its assessment usually requires dedicated advanced image acquisitions. Radiomics analyses bear the potential to describe radiophenotypes beyond what meets the naked eye. We sought to: 1) evaluate this novel approach to predict white matter hyperintensity (WMH) burden and 2) uncover latent clinico-radiological associations. Methods: An international, multi-site cohort of 4,164 acute ischemic stroke (AIS) patients with FLAIR MRI (MRI-GENIE study) underwent total brain and WMH lesion segmentation using convolutional neural networks. Radiomic features (n=1905) were extracted from clinical FLAIR images outside of the WMH (brain mask - WMH mask). Prediction of the WMH burden using radiomics was done using LASSO regression. Radiomic signature of WMH was built with the most stable selected features, then compared to the clinical variables using canonical correlation analysis. Results: In this cohort, (mean age=62.8±15.0, median WMH volume=4.2cc IQR 1.4-11.2), radiomic features were highly predictive of WMH burden (R2=0.8±0.012). Radiomic signature of WMH included 68 features. All 7 pairs of extracted canonical variates (CV) were statistically significant with respective canonical correlations of 0.79, 0.64, 0.44, 0.21, 0.16, 0.15 (Bonferroni corrected p-values CV1-6 CV7 =.003). Upon examination, CV1 was mainly influenced by age, CV2 by sex, CV3 by history of smoking and diabetes mellitus (DM), CV4 by hypertension, CV5 by atrial fibrillation (AF) and DM, CV6 by coronary artery disease (CAD) and CV7 by CAD and DM. Conclusion: Radiomics extracted from clinical grade FLAIR images of AIS patients seem able to capture structural integrity of the cerebral parenchyma and to correlate with clinical phenotypes. Further research could evaluate radiomics to predict the progression of cerebral small vessel disease on longitudinal data.
更多查看译文
关键词
Brain Health,Cerebrovascular disorders,Machine Learning,Ischemic stroke,Magnetic resonance neuroimaging
AI 理解论文
溯源树
样例
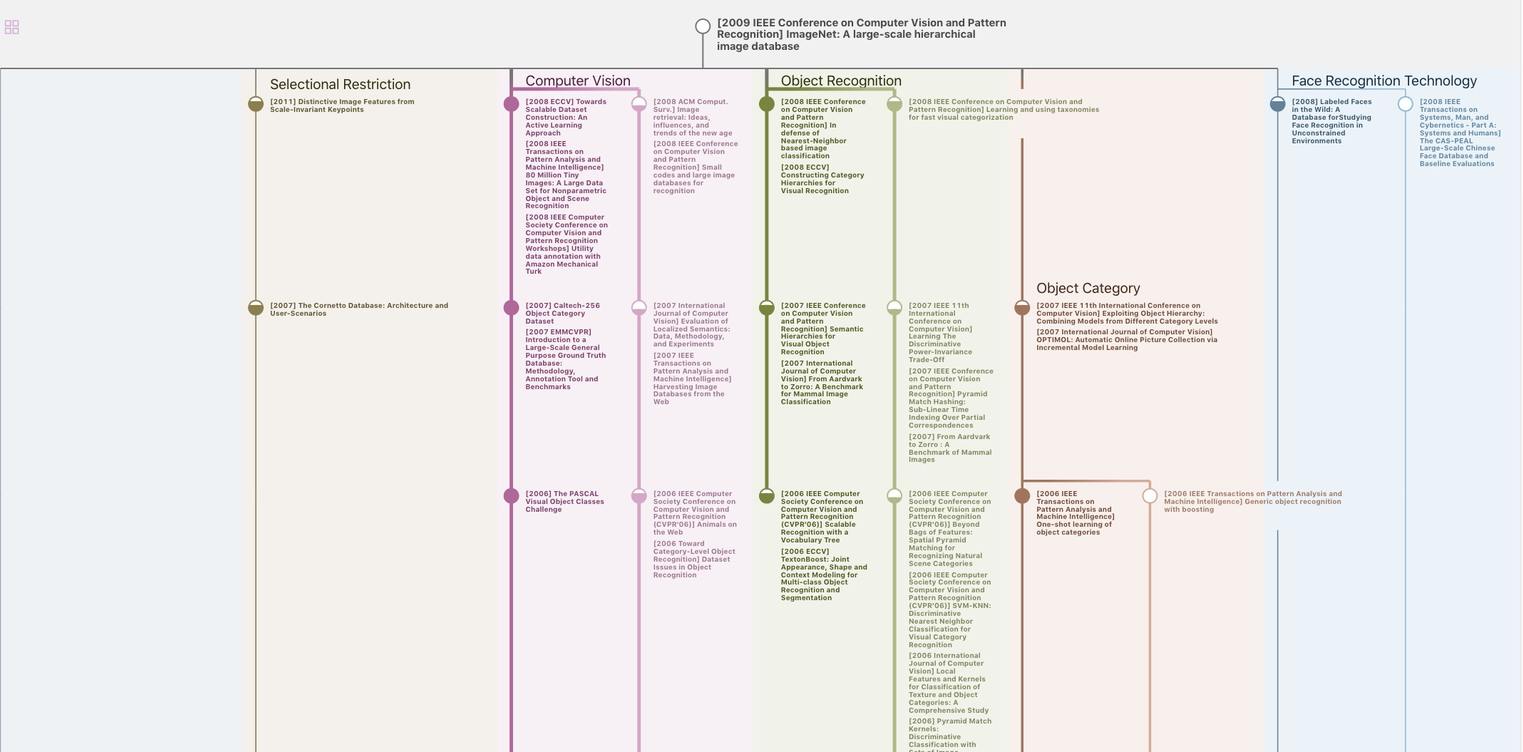
生成溯源树,研究论文发展脉络
Chat Paper
正在生成论文摘要