Sensors and Machine Learning Models to Prevent Cooktop Ignition and Ignore Normal Cooking
FIRE TECHNOLOGY(2021)
摘要
Cooking equipment is involved in nearly half of home fires in the United States, with cooktop fires the leading cause of deaths and injuries in cooking-related fires. In this study, we evaluate 16 electrochemical, optical, temperature and humidity sensors, placed in the cooktop exhaust duct, for use in predicting and preventing cooktop ignition. The sensors were evaluated in a series of 60 experiments conducted in a mock kitchen. Experiments covered a broad range of conditions, including both unattended cooking and normal cooking scenarios, where 39 experiments led to auto-ignition. The experiments involved a variety of cooking oils and foods and were conducted using either an electric coil cooktop, gas-fueled cooktop, or electric oven. The sensor data collected in the experiments were used in two types of analysis, threshold analysis and neural-network analysis, to estimate the performance of the sensors for predicting ignition and ignoring normal cooking conditions. The combined information from multiple sensors was evaluated in sensor ratios with threshold analysis, and in the neural-network models developed using selected pairs of sensor inputs. Some of the multiple-sensor cases performed as well as or better than the individual sensor thresholds and individual sensor models. Consistently across threshold and machine learning analysis, the best performing sensor was the sensor measuring volatile organic compounds. This sensor was also included in all of the best performing sensor ratios and machine learning models.
更多查看译文
关键词
Cooktop ignition, Sensor analysis, Neural networks, Ignition prevention
AI 理解论文
溯源树
样例
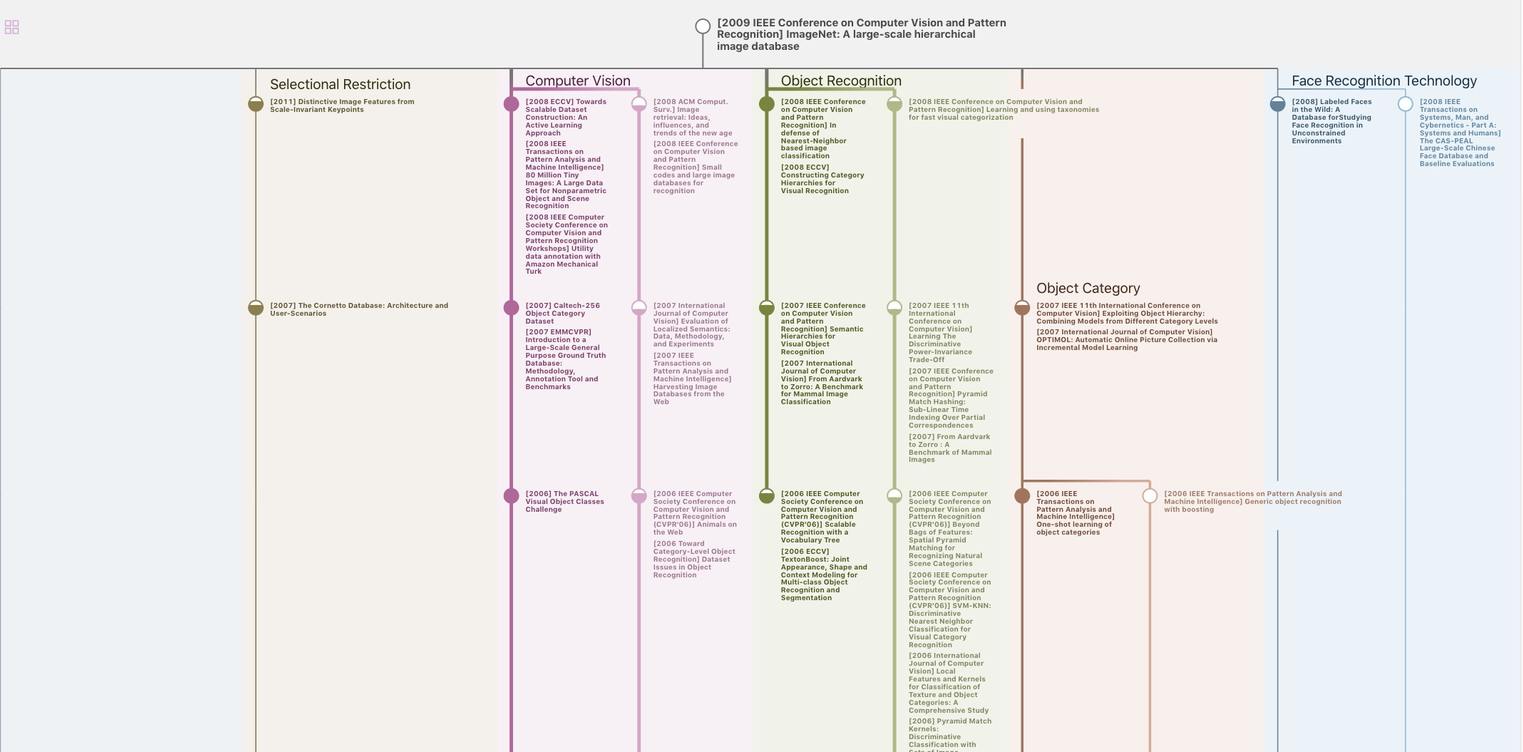
生成溯源树,研究论文发展脉络
Chat Paper
正在生成论文摘要