Super Resolution for Multi-Sources Image Stream Data Using Smooth and Sparse Tensor Completion and Its Applications in Data Acquisition of Additive Manufacturing
TECHNOMETRICS(2022)
摘要
Recent developments of advanced imaging systems spur their applications in many areas, ranging from satellite remote sensing for geographic information to thermal imaging analysis for manufacturing process monitoring and control. Due to different specifications of imaging systems, the resulting image stream data (videos) have different spatial and temporal resolutions. This proposed work is based on the image stream data captured by multiple imaging systems for the same object with different but complementary spatial and temporal resolutions. For example, one system has high spatial but low temporal resolutions while the other one has opposite resolutions. The goal of this article is to develop a new super resolution method that integrates these different types of image stream data to improve both spatial and temporal resolutions, which is critical to obtaining more insightful information for more effective quality control of targeted processes or systems. To fulfill this goal, a new tensor completion model is developed by considering both smooth and sparse features simultaneously and is thus termed smooth and sparse tensor completion (SSTC). The results of the extensive case studies illustrate the superiority of our method over the elaborately selected benchmark methods.
更多查看译文
关键词
CANDECOMP, PARAFAC (CP) decomposition, Rank estimation, Smooth and sparse decomposition
AI 理解论文
溯源树
样例
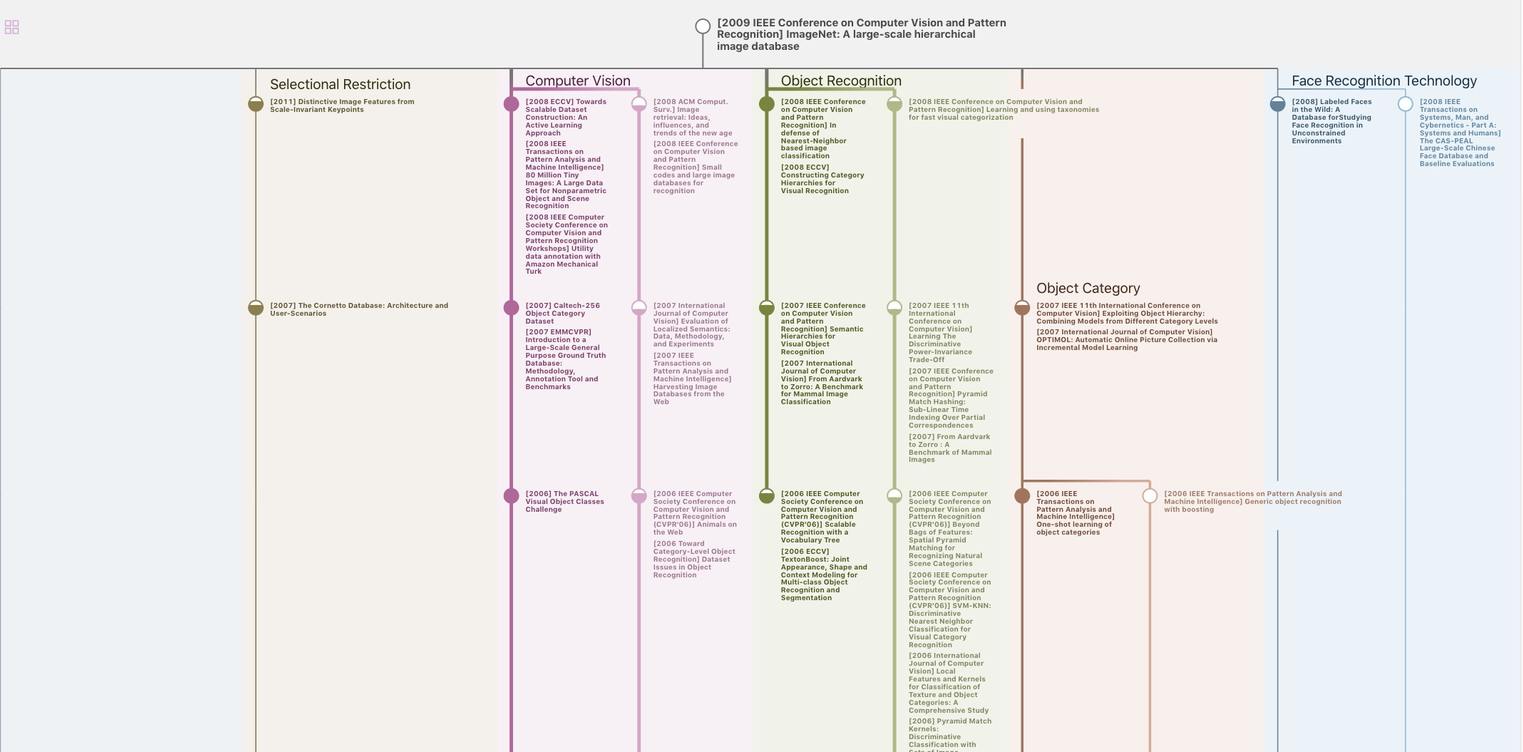
生成溯源树,研究论文发展脉络
Chat Paper
正在生成论文摘要