An Intelligent Hybrid Classification Algorithm Integrating Fuzzy Rule-Based Extraction And Harmony Search Optimization: Medical Diagnosis Applications
KNOWLEDGE-BASED SYSTEMS(2021)
摘要
Uncertainty is a critical factor in medical datasets needed to be overcome for increasing diagnosis efficiency. This paper proposes an intelligent classification algorithm comprising a fuzzy rule-based approach, a harmony search (HS) algorithm, and a heuristic algorithm to classify medical datasets intelligently. Two fuzzy approaches, as well as orthogonal and triangular fuzzy sets, are first utilized to define the attributes of data. Then, an HS algorithm is integrated with a heuristic to generate fuzzy rules to select the best rules in the fuzzy rule-based systems. Moreover, to improve the performance of the proposed classification approach, a three-phase parameter tuning approach is applied. First, the Taguchi method (phase I) is employed to tune the parameters of the HS algorithm using a fixed number of training data and find the central points of the parameters' values. Then, a nested cross-validation (CV) approach consisting of an outer CV (phase II) and an inner CV (phase III) is utilized. Using the Taguchi approach gives the advantage of not considering a wide range of parameters by the nested CV which produces better results on the medical dataset. Nine well-known medical datasets are used to evaluate the efficiency of the proposed hybrid algorithm. To this aim, the results obtained by the algorithm are compared with the ones of several related works in the literature where several statistical tests and graphical approaches are used for comparisons. The results show that the proposed methods are robust in data analysis and classification of clinical datasets. (c) 2021 Published by Elsevier B.V.
更多查看译文
关键词
Machine learning classifier, Fuzzy rule-based system, Harmony search algorithm, Taguchi method, Nested cross-validation, Medical diagnosis
AI 理解论文
溯源树
样例
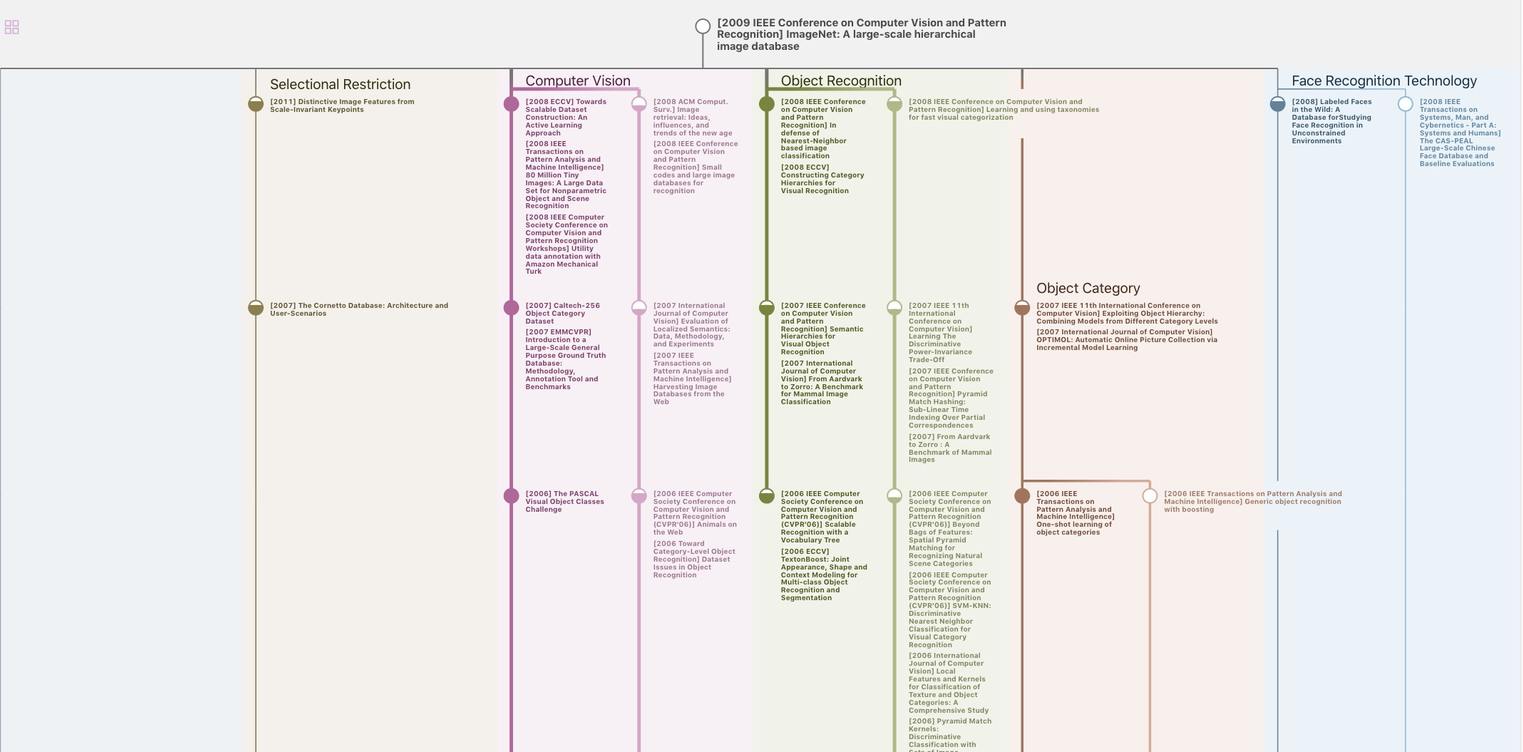
生成溯源树,研究论文发展脉络
Chat Paper
正在生成论文摘要