Knowledge Transfer in Board-Level Functional Fault Diagnosis Enabled by Domain Adaptation
IEEE Transactions on Computer-Aided Design of Integrated Circuits and Systems(2022)
摘要
High integration densities and design complexity make board-level functional fault diagnosis extremely difficult. Machine-learning techniques can identify functional faults with high accuracy, but they require a large volume of data to achieve high-prediction accuracy. This drawback limits the effectiveness of traditional machine-learning algorithms for training a model in the early stage of manufacturing, when only a limited amount of fail data and repair records are available. We propose a board-level diagnosis workflow that utilizes domain adaptation (DA) to transfer the knowledge learned from mature boards to a new board in the ramp-up phase. First, based on the requirement of fault diagnosis, we select an appropriate domain-adaptation method to reduce differences between mature boards and the new board. Second, these DA methods utilize information from both the mature and the new boards with carefully designed domain-alignment rules and train a functional fault diagnosis classifier. Experimental results using three complex boards in volume production and one new board in the ramp-up phase show that, with the help of DA and the proposed workflow, the diagnosis accuracy is improved.
更多查看译文
关键词
Domain adaptation,fault diagnosis,functional test,knowledge transfer,machine learning
AI 理解论文
溯源树
样例
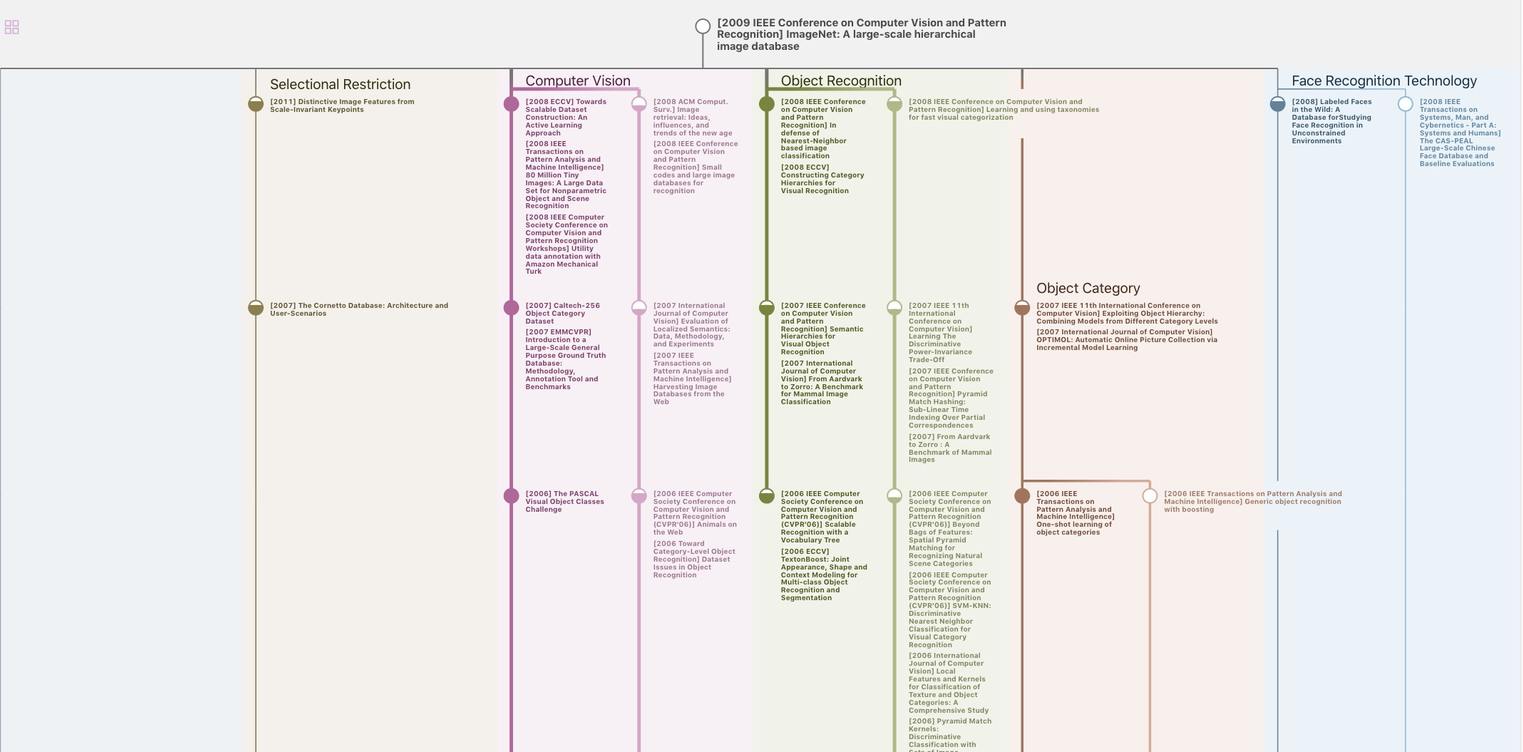
生成溯源树,研究论文发展脉络
Chat Paper
正在生成论文摘要