Towards Coding For Human And Machine Vision: Scalable Face Image Coding
IEEE TRANSACTIONS ON MULTIMEDIA(2021)
摘要
The past decades have witnessed the rapid development of image and video coding techniques in the era of big data. However, the signal fidelity-driven coding pipeline design limits the capability of the existing image/video coding frameworks to fulfill the needs of both machine and human vision. In this paper, we come up with a novel face image coding framework by leveraging both the compressive and the generative models, to support machine vision and human perception tasks jointly. Given an input image, the feature analysis is first applied, and then the generative model is employed to reconstruct image with compact structure and color features, where sparse edges are extracted to connect both kinds of vision and a key reference pixel selection method is proposed to determine the priorities of the reference color pixels for scalable coding. The compact edge map serves as the basic layer for machine vision tasks, and the reference pixels act as an enhanced layer to guarantee signal fidelity for human vision. By introducing advanced generative models, we train a decoding network to reconstruct images from compact structure and color representations, which is flexible to accept inputs in a scalable way and to control the imagery effect of the outputs between signal fidelity and visual realism. Experimental results and comprehensive performance analysis over the face image dataset demonstrate the superiority of our framework in both human vision tasks and machine vision tasks, which provide useful evidence on the emerging standardization efforts on MPEG VCM (Video Coding for Machine).
更多查看译文
关键词
Image coding, Machine vision, Task analysis, Image reconstruction, Visualization, Feature extraction, Image color analysis, Generative compression, image coding, scalable coding, video coding for machine
AI 理解论文
溯源树
样例
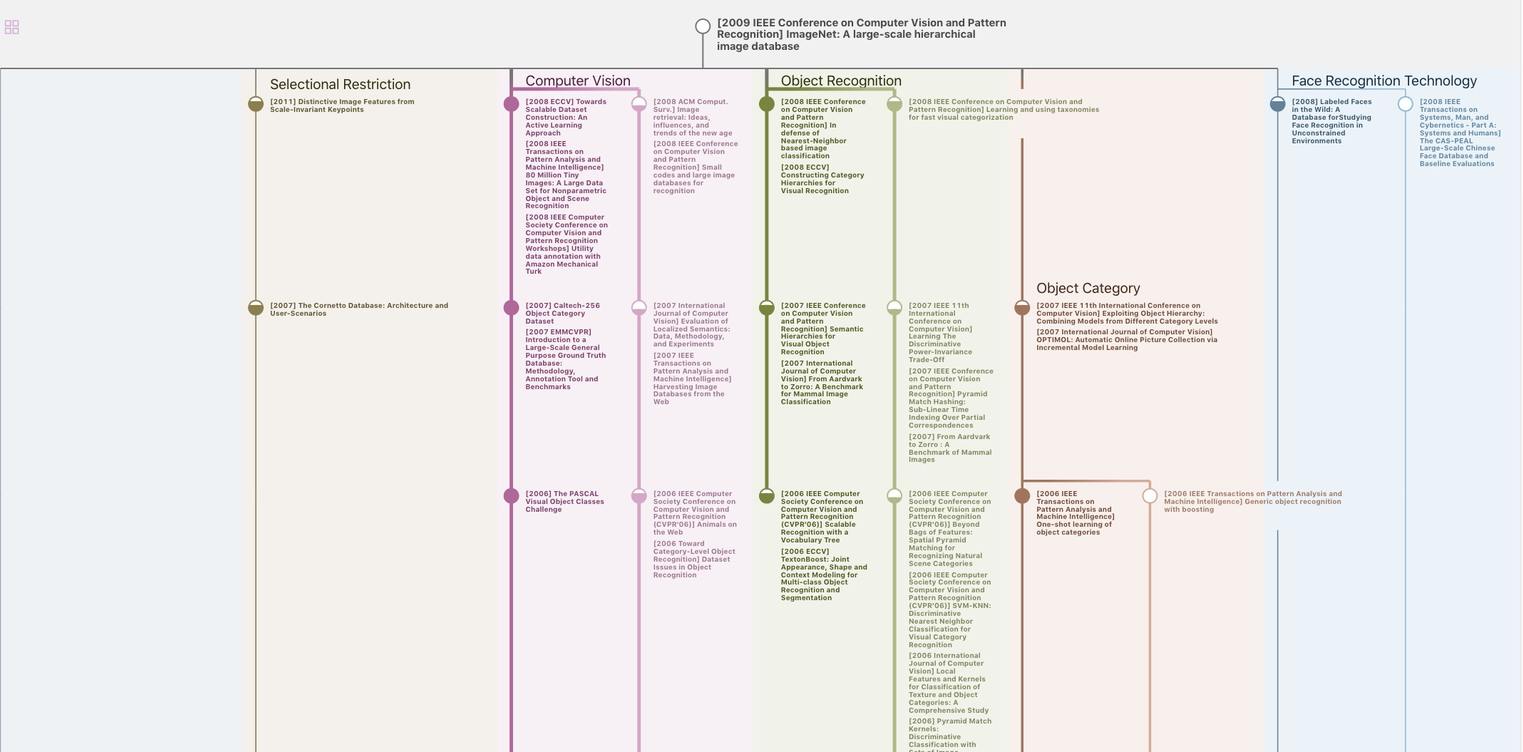
生成溯源树,研究论文发展脉络
Chat Paper
正在生成论文摘要