How Do Simple Transformations of Text and Image Features Impact Cosine-Based Semantic Match?
european conference on information retrieval(2021)
摘要
Practitioners often resort to off-the-shelf feature extractors such as language models (e.g., BERT or Glove) for text or pre-trained CNNs for images. These features are often used without further supervision in tasks such as text or image retrieval and semantic similarity with cosine-based semantic match. Although cosine similarity is sensitive to centering and other feature transforms, their impact on task performance has not been systematically studied. Prior studies are limited to a single domain (e.g., bilingual embeddings) and one data modality (text). Here, we systematically study the effect of simple feature transforms (e.g., standardizing) in 25 datasets with 6 tasks covering semantic similarity and text and image retrieval. We further back up our claims in ad-hoc laboratory experiments. We include 15 (8 image + 7 text) embeddings, covering the state-of-the-art models. Our second goal is to determine whether the common practice of defaulting to the cosine similarity is empirically supported. Our findings reveal that: (i) some feature transforms provide solid improvements, suggesting their default adoption; (ii) cosine similarity fares better than Euclidean similarity, thus backing up standard practices. Ultimately, our takeaways provide actionable advice for practitioners.
更多查看译文
关键词
Feature transform, Cosine similarity, Image retrieval, Text retrieval, Semantic similarity, Text embeddings, Image embeddings
AI 理解论文
溯源树
样例
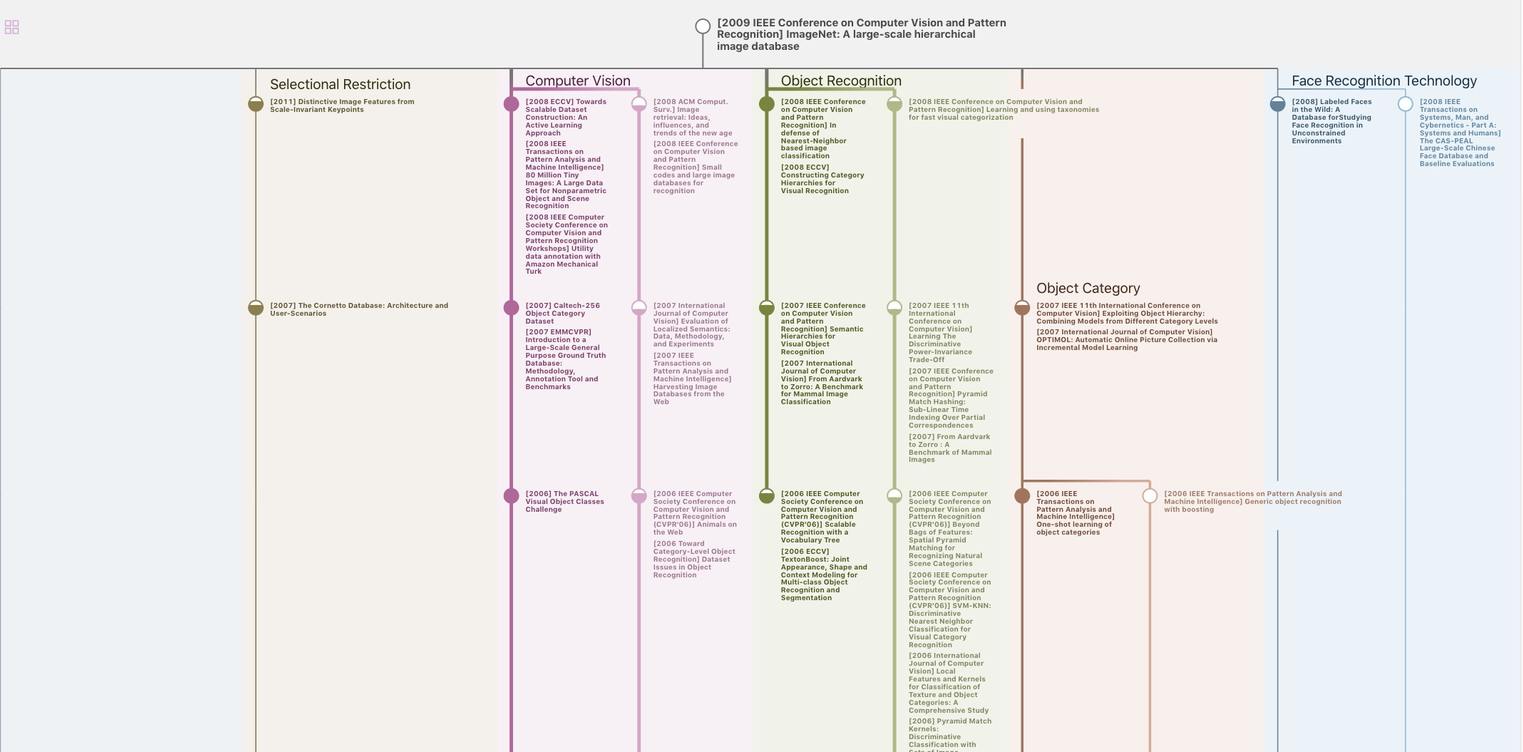
生成溯源树,研究论文发展脉络
Chat Paper
正在生成论文摘要