Image Tampering Localization Using A Dense Fully Convolutional Network
IEEE TRANSACTIONS ON INFORMATION FORENSICS AND SECURITY(2021)
摘要
The emergence of powerful image editing software has substantially facilitated digital image tampering, leading to many security issues. Hence, it is urgent to identify tampered images and localize tampered regions. Although much attention has been devoted to image tampering localization in recent years, it is still challenging to perform tampering localization in practical forensic applications. The reasons include the difficulty of learning discriminative representations of tampering traces and the lack of realistic tampered images for training. Since Photoshop is widely used for image tampering in practice, this paper attempts to address the issue of tampering localization by focusing on the detection of commonly used editing tools and operations in Photoshop. In order to well capture tampering traces, a fully convolutional encoder-decoder architecture is designed, where dense connections and dilated convolutions are adopted for achieving better localization performance. In order to effectively train a model in the case of insufficient tampered images, we design a training data generation strategy by resorting to Photoshop scripting, which can imitate human manipulations and generate large-scale training samples. Extensive experimental results show that the proposed approach outperforms state-of-the-art competitors when the model is trained with only generated images or fine-tuned with a small amount of realistic tampered images. The proposed method also has good robustness against some common post-processing operations.
更多查看译文
关键词
Location awareness, Tools, Training, Feature extraction, Deep learning, Decoding, Transform coding, Image forensics, image tampering localization, fully convolutional network, densely convolutional network, dilated convolution, Photoshop scripting
AI 理解论文
溯源树
样例
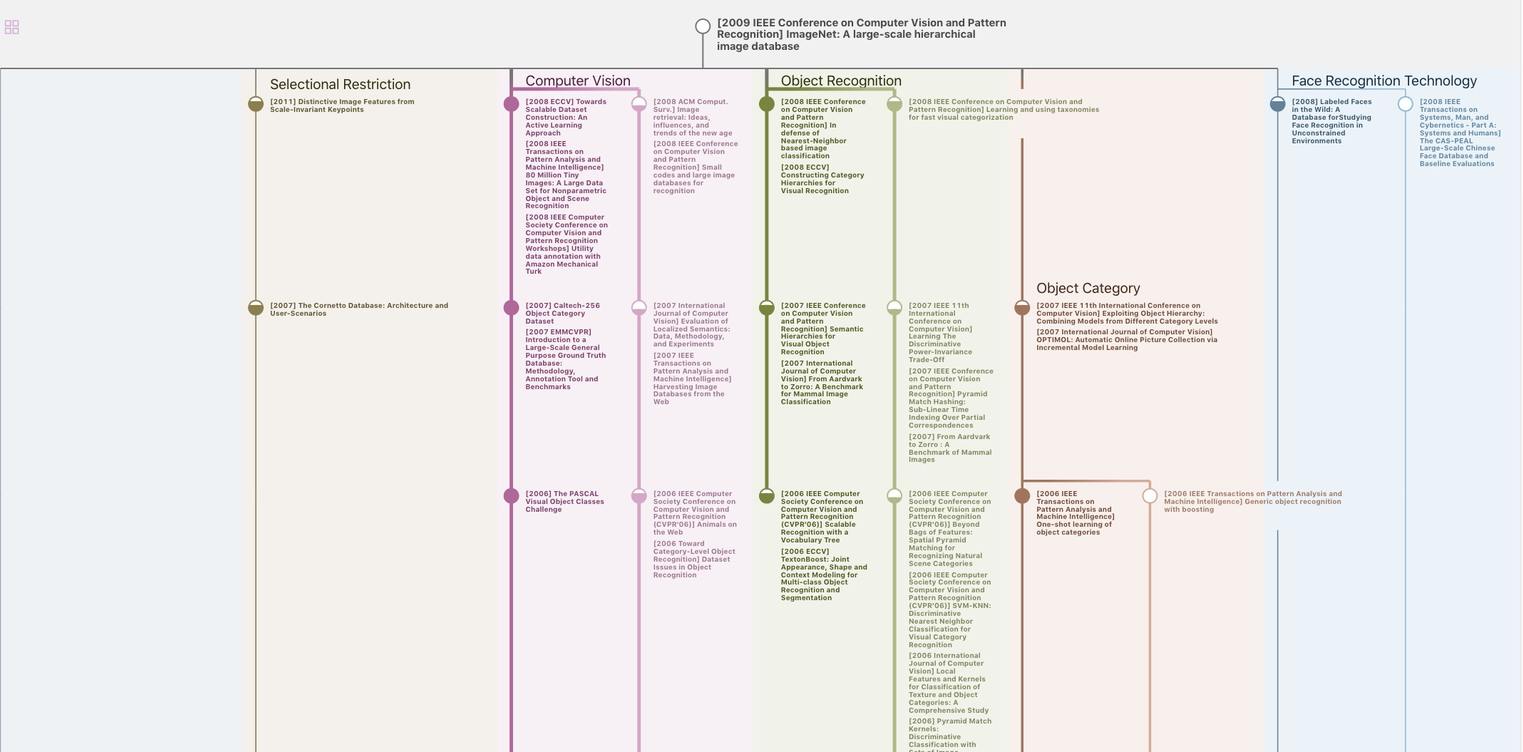
生成溯源树,研究论文发展脉络
Chat Paper
正在生成论文摘要