Feature selection procedures for combined density functional theory - artificial neural network schemes
Physica Scripta(2021)
摘要
We propose a workflow which includes the essential step of feature selection in order to optimize combined density functional theory-machine learning schemes (DFT-ML). Here, the energy gaps of hybrid graphene-boron nitride nanoflakes with randomly distributed domains are predicted using artificial neural networks (ANNs). The training data is obtained by associating structural information to the target quantity of interest, i.e. the energy gap, obtained by DFT calculations. The selection of proper feature vectors is important for an accurate and efficient ANN model. However, finding an optimal set of features is generally not trivial. We compare different approaches for selecting the feature vectors, ranging from random selection of the features to guided approaches like removing the features with lowest variance and by using the mutual information regression selection technique. We show that the feature selection procedures provides a significant reduction of the input space dimensionality. In addition, a selection method based on the ranking of the cutting radius is proposed and evaluated. This may not only be important for establishing optimal ANN models, but may offer insights into the minimum information required to map certain targeted properties.
更多查看译文
关键词
feature selection, neural networks, density functional theory
AI 理解论文
溯源树
样例
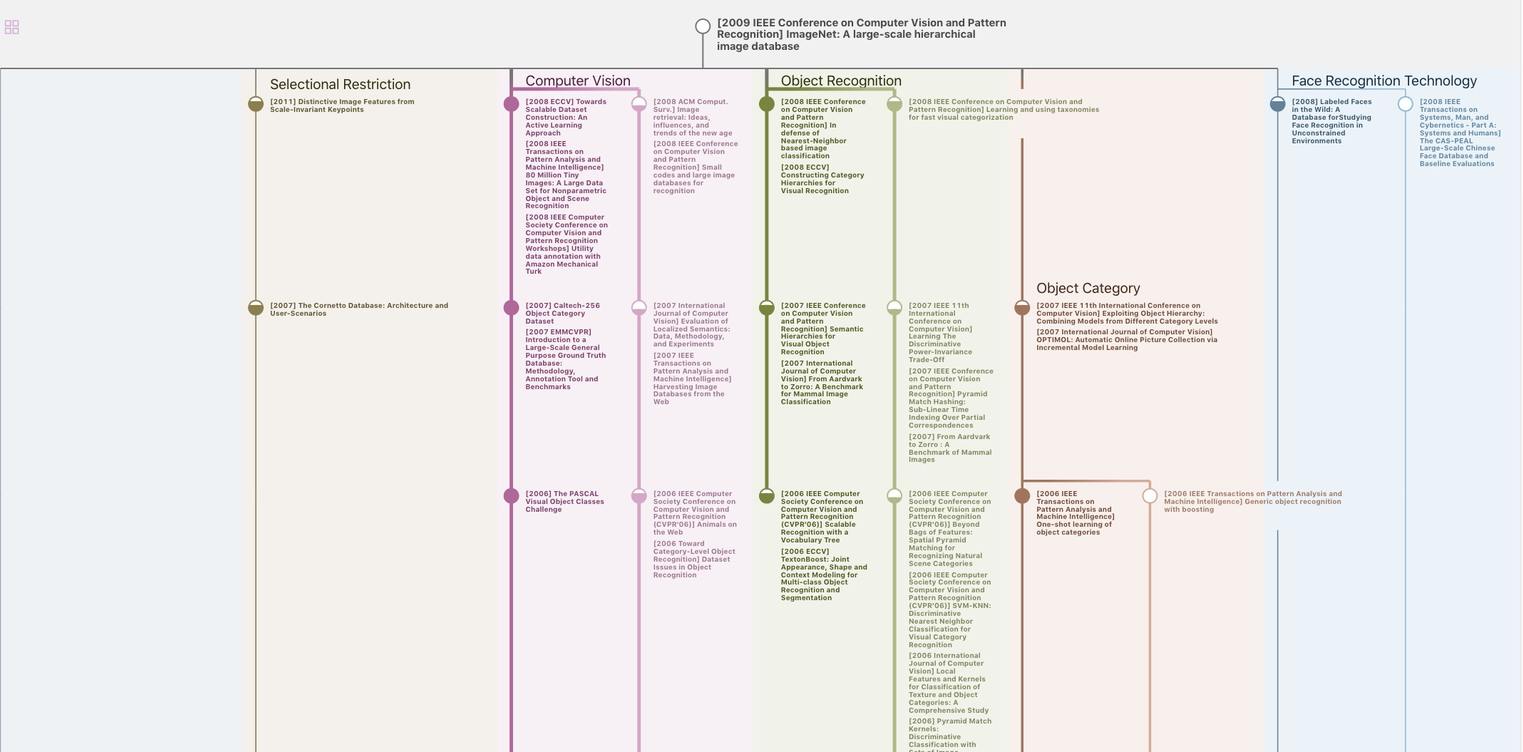
生成溯源树,研究论文发展脉络
Chat Paper
正在生成论文摘要