Modeling Gully Erosion Susceptibility in Phuentsholing, Bhutan Using Deep Learning and Basic Machine Learning Algorithms
Environmental earth sciences(2021)
摘要
The present study attempts to demarcate the areas susceptible to gully erosion in Phuentsholing, Bhutan, using Deep Learning CNN (convolution neural network) and artificial neuron network (ANN), Support Vector Machine (SVM) and maximum entropy, three basic machine learning techniques in the GIS setting. Application of deep learning technique is new in the field of gully erosion. Considering the 240 gully pixels and seventeen gully erosion conditioning factors (GECFs), the gully erosion susceptibility maps (GESMs) were prepared. Out of the 240 gully pixels, 70% were used as training datasets and 30% were used as validation datasets for modeling and judging the GESMs. The GECFs were selected based on the previous literatures and multi-collinearity test. The importance of the GECFs was assessed by the chi-square attribute evaluation (CSEA) and random forest (RF) methods. Finally, applying the receiver operating characteristics’ area under curve (AUC-ROC), RMSE, MAE and R-index, the robustness of the GESMs was evaluated and compared. The GESMs were classified using natural break classification method into very high, high, moderate, low and very low susceptible classes. Nearly, 20% of the study area has very high susceptibility to gully erosion. As per the results of CSEA and RF methods, sand concentration, land use\cover and altitudes have the largest contribution in making the area very susceptible to gully erosion. Results of the validation techniques recognized the entire selected model as accurate and robust. Among the selected models, the capability of CNN model (AUC = 0.910, MAE = 0.029, RMSE = 0.171 for training data and AUC = 0.929, MAE = 0.089, RMSE = 0.299 for testing data) in predicting the gully erosion susceptibility is higher than other models. The produced GESMs will be helpful to the researchers as well as decision makers in establishing gully erosion management strategies.
更多查看译文
关键词
Deep learning,Gully erosion susceptibility,CNN,Chi-square attribute evaluation,Phuentsholing
AI 理解论文
溯源树
样例
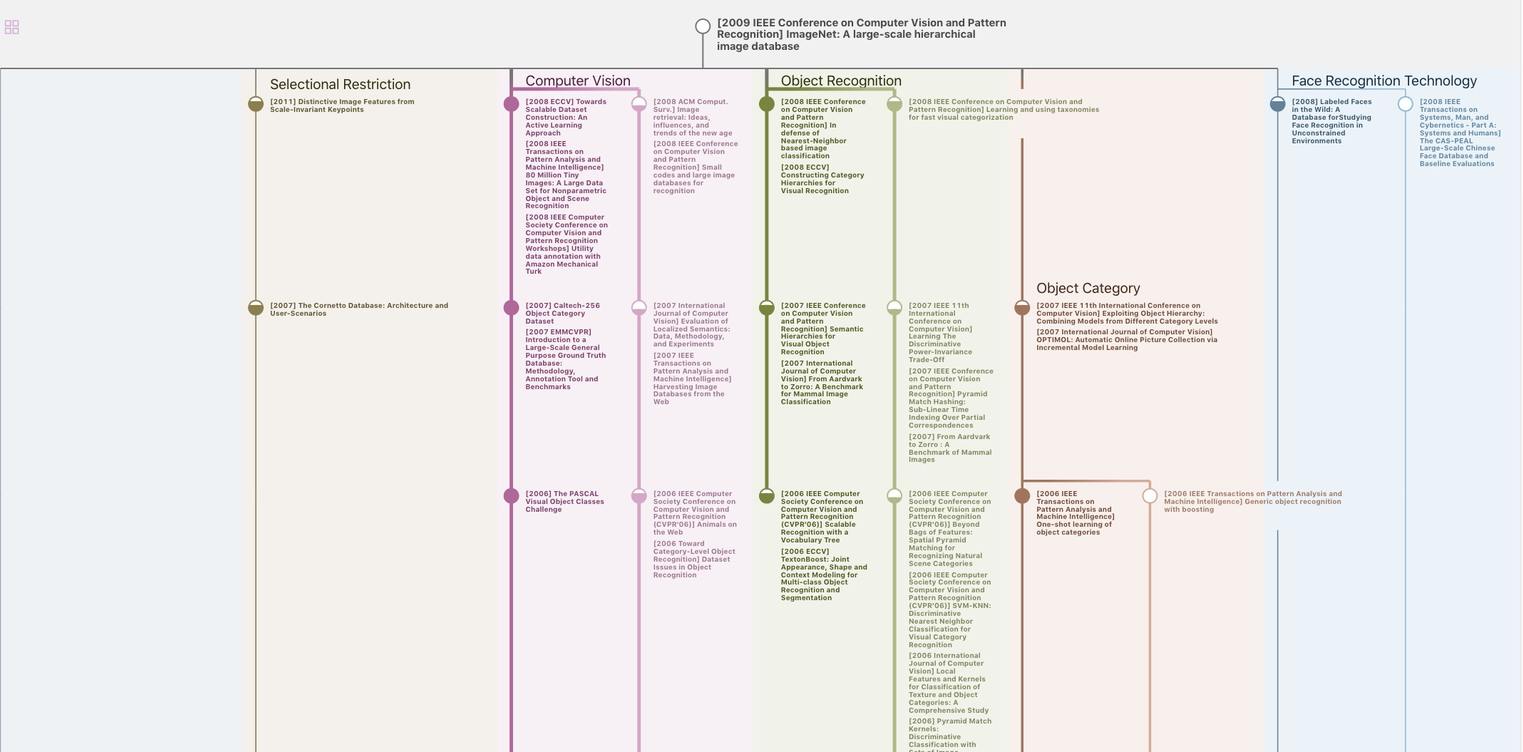
生成溯源树,研究论文发展脉络
Chat Paper
正在生成论文摘要