Assimilation of soil moisture and canopy cover data improves maize simulation using an under-calibrated crop model
Agricultural Water Management(2021)
摘要
Parameter calibration is normally required prior to crop model simulation, which can be a time-consuming and data-intensive task. Meanwhile, the growth stages of different hybrids/cultivars of the same crop often show some similarities, which implies that phenological parameters calibrated for one hybrid/cultivar may be useful for the simulation of another. In this study, a data assimilation framework is proposed to reduce the requirement for parameter calibration for maize simulation using AquaCrop. The phenological parameters were uniformly scaled from previous research performed in a different location for a different maize hybrid, and other parameters were taken from default settings in the model documentation. To constrain simulation uncertainties, soil moisture and canopy cover observations were assimilated both separately and jointly in order to update model states. The methodology was tested across a rain-fed field in Nebraska for 6 growing seasons. The results suggested that the under-calibrated model with uniformly scaled phenological parameters captured the temporal dynamics of crop growth, but may lead to large estimation bias. Data assimilation effectively improved model performance, and the joint assimilation outperformed single-variable assimilation. When soil moisture and canopy cover were jointly assimilated, the overall yield estimates (RMSE = 1.24 t/ha, nRMSE = 11.48%, R2 = 0.695) were improved over the no-assimilation case (RMSE = 2.01 t/ha, nRMSE = 18.61%, R2 = 0.338). Sensitivity analyses suggested that the improvement was still evident with temporally sparse soil moisture observations and a small ensemble size. Further testing using observations within 90 days after planting demonstrated that the method was able to predict yield around 3 months before harvest (RMSE = 1.7 t/ha, nRMSE = 15.74%). This study indicated that maize yield can be estimated and predicted accurately by monitoring the soil moisture and canopy status, which has potential for regional applications using remote sensing data.
更多查看译文
关键词
AquaCrop,Calibration,Data assimilation,Canopy cover,Soil moisture,Yield prediction
AI 理解论文
溯源树
样例
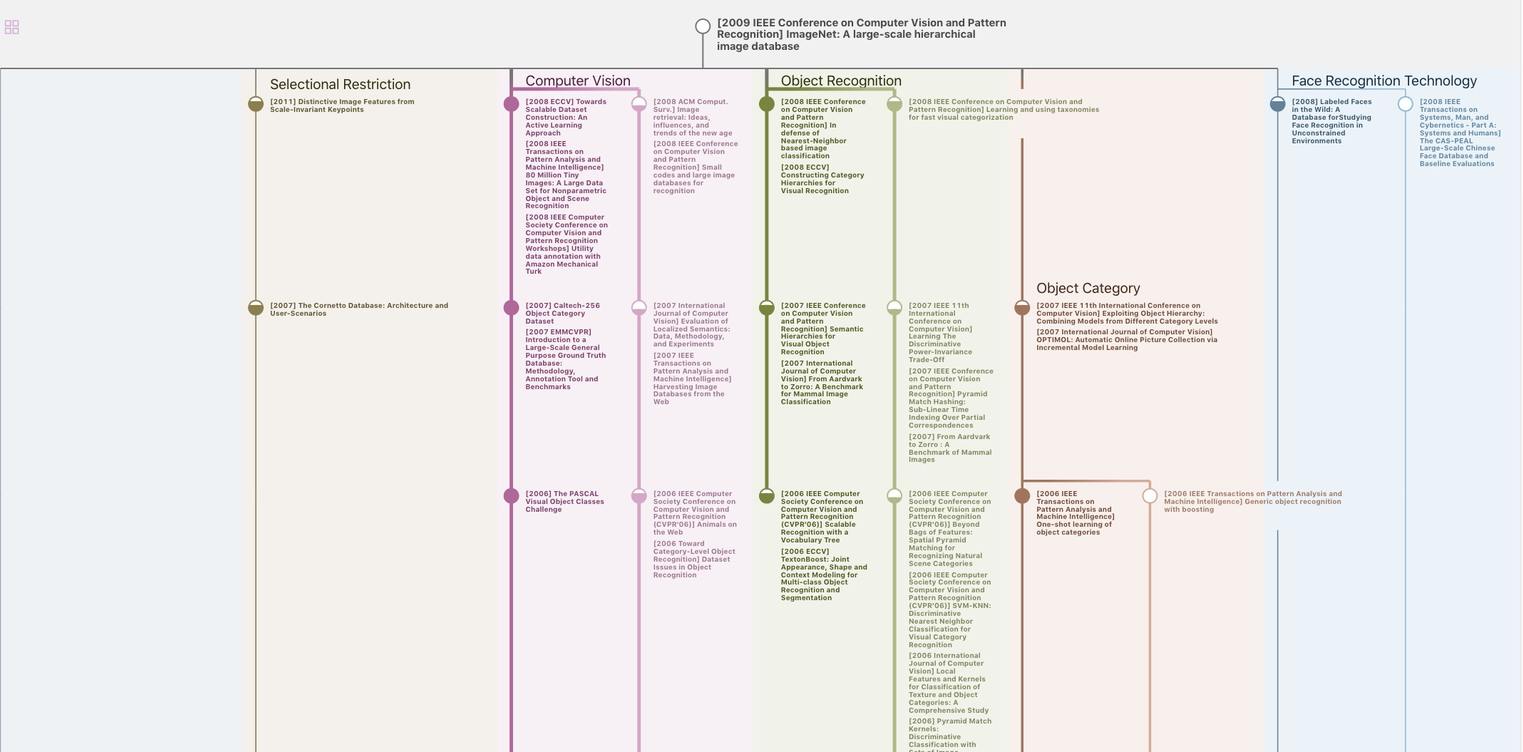
生成溯源树,研究论文发展脉络
Chat Paper
正在生成论文摘要