Estimating Ground-Level Hourly PM2.5 Concentrations over North China Plain with Deep Neural Networks
Photonirvachak(2021)
摘要
Fine particulate matter (PM 2.5 ) has a considerable impact on the environment, climate change, and human health. Herein, we introduce a deep neural network model for deriving ground-level, hourly PM 2.5 concentrations by Himawari-8 aerosol optical depth, meteorological variables, and land cover information. A total of 151,726 records were collected from 313 ground-level PM 2.5 monitoring stations (spread across the North China Plain) to calibrate and test the proposed model. The sample- and site-based cross-validation yielded satisfactory performance, with correlation coefficients > 0.8 ( R = 0.86 and 0.83, respectively). Furthermore, the variation in mean ground-level hourly PM 2.5 concentrations, using 2017 data, showed that the proposed method could be applied for spatiotemporal continuous PM 2.5 monitoring. This study will serve as a reference for the application of geostationary meteorological satellite to perform ground-level PM 2.5 estimation and the utilization in atmospheric monitoring.
更多查看译文
关键词
Fine particulate matter,Deep neural networks,North China Plain,Himawari-8,Aerosol optical depth
AI 理解论文
溯源树
样例
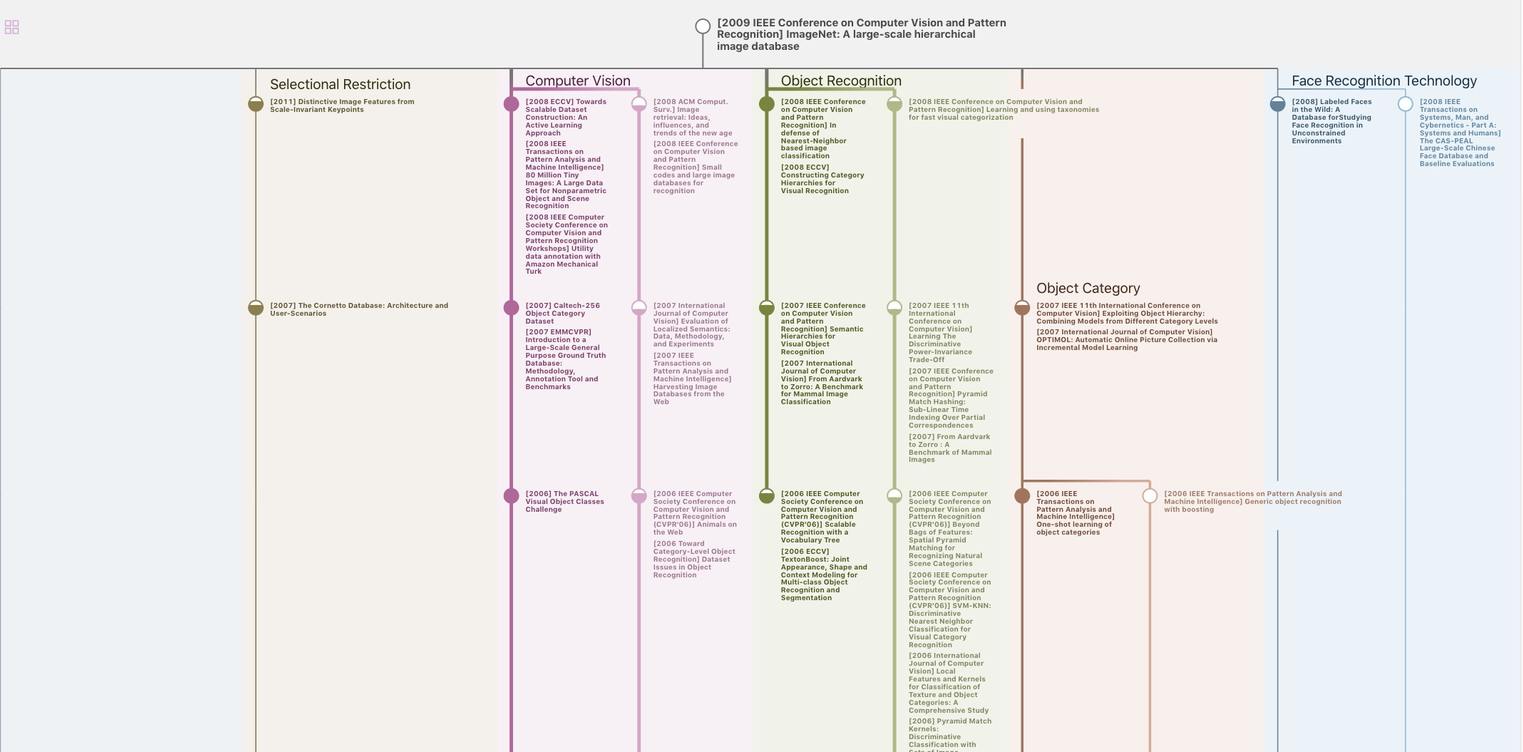
生成溯源树,研究论文发展脉络
Chat Paper
正在生成论文摘要