Spatial Pyramid Attention for Deep Convolutional Neural Networks
IEEE TRANSACTIONS ON MULTIMEDIA(2021)
摘要
Attention mechanisms have shown great success in computer vision. However, the commonly used global average pooling in some implementations aggregates a three-dimensional feature map to a one-dimensional attention map, leading a significant loss of structural information in the attention learning. In this article, we present a novel Spatial Pyramid Attention Network (SPANet), which exploits the structural information and channel relationships for better feature representation. SPANet enhances a base network by adding Spatial Pyramid Attention (SPA) blocks laterally. By rethinking the self-attention mechanism design, we further present three topology structures of attention path connection for our SPANet. They can be flexibly applied to various CNN architectures. SPANet is conceptually simple but practically powerful. It uses both structural regularization and structural information to achieve better learning capability. We have comprehensively evaluated the performance of SPANet on four benchmark datasets for different visual tasks. The experimental results show that SPANet significantly improves the recognition accuracy without adding much computation overhead. Using SPANet, we achieve an improvement of 1.6% top-1 classification accuracy on the ImageNet 2012 benchmark based on ResNet50, and SPANet outperforms SENet and other attention methods. SPANet also significantly improves the object detection performance by a clear margin with negligible additional computation overhead. When applying SPANet to RetinaNet based on the ResNet50 backbone, we improve the performance of the baseline model by 2.3 mAP and the enhanced model outperforms SENet and GCNet by 1.1 mAP and 1.7 mAP respectively. The code of SPANet is made publicly available.
1
更多[Online]. Available:
查看译文
关键词
Object detection, Feature extraction, Convolutional codes, Computer architecture, Benchmark testing, Topology, Task analysis, Attention mechanism, convolutional neural network, image classification, object detection, spatial pyramid structure, structural regularization, structural information
AI 理解论文
溯源树
样例
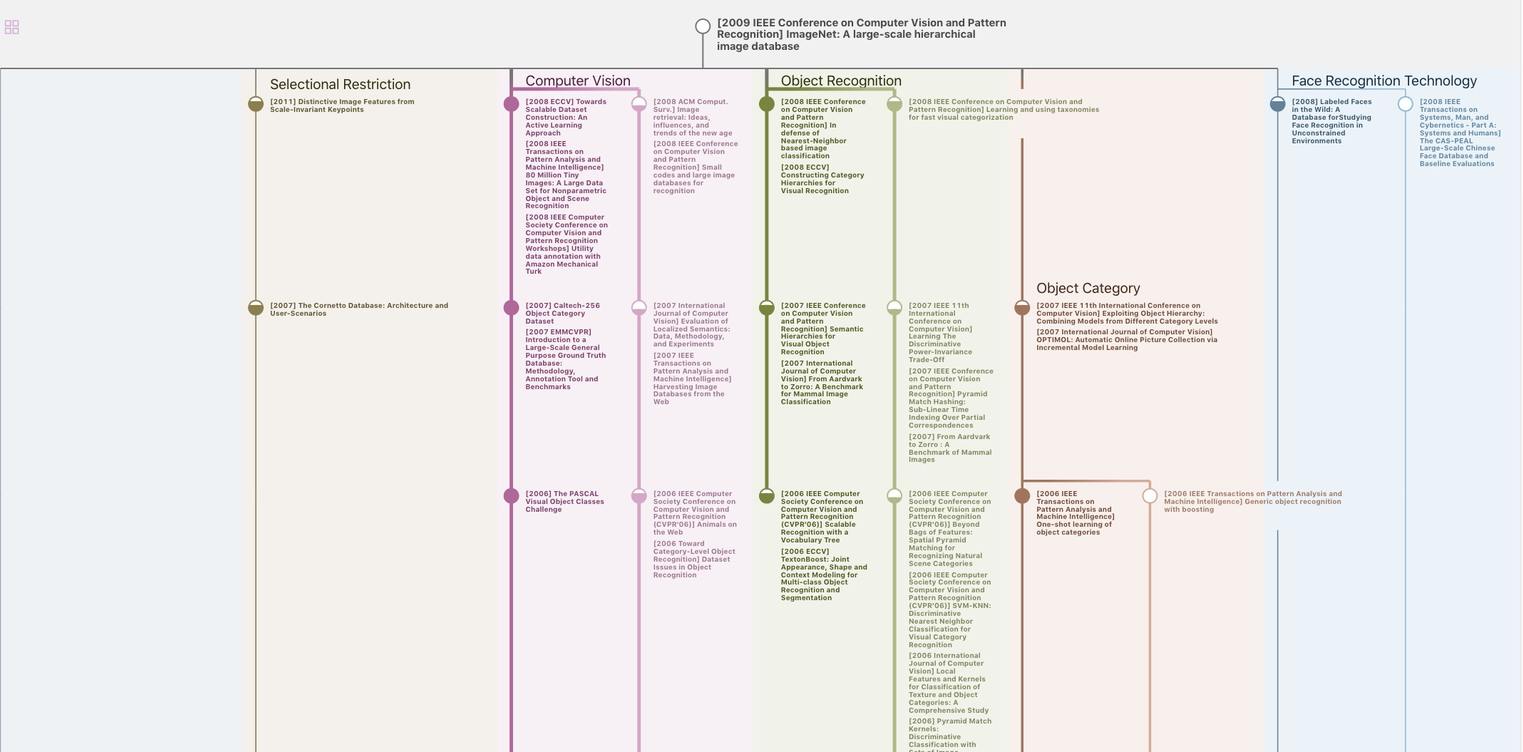
生成溯源树,研究论文发展脉络
Chat Paper
正在生成论文摘要