Weighted Discriminative Sparse Representation for Image Classification
NEURAL PROCESSING LETTERS(2021)
Abstract
Sparse representation methods based on l _2 norm regularization have attracted much attention due to its low computational cost and competitive performance. How to enhance the discriminability of l _2 norm regularization-based representation method is a meaningful work. In this paper, we put forward a novel l _2 norm regularization-based representation method, called Weighted Discriminative Sparse Representation for Classification (WDSRC), in which we consider the global discriminability and the local discriminability using two discriminative regularization terms of representation. The global discriminability is obtained by decorrelating the representation results stemming from all distinct classes. The local discriminability is achieved by the weighted representation in which the representation coefficient of the training images dissimilar to the test image will be reduced and the representation coefficient of the training images similar to the test image will be increased, which restrains the training images dissimilar to the test image and promotes the training images similar to the test image as much as possible in representing the test sample. By considering the global and local discriminability of representations simultaneously, the proposed WDSRC method can gain more discriminative representation for classification. Extensive experiments on benchmark datasets of object, face, action and flower demonstrate the effectiveness of the proposed WDSRC method.
MoreTranslated text
Key words
Discriminative representation, Sparse representation, Global and local discriminability, Image classification
AI Read Science
Must-Reading Tree
Example
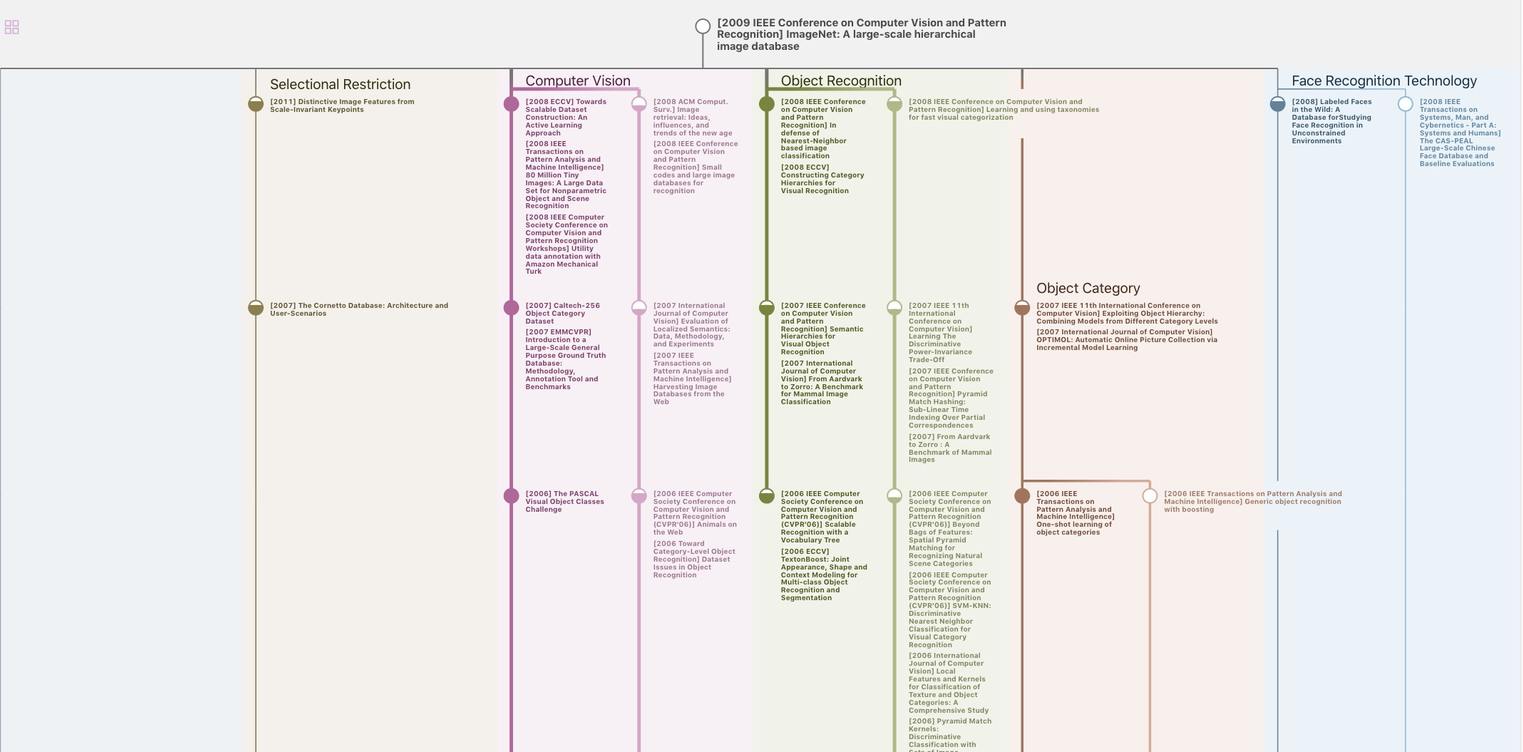
Generate MRT to find the research sequence of this paper
Chat Paper
Summary is being generated by the instructions you defined