A bearing fault diagnosis model based on CNN with wide convolution kernels
Journal of Ambient Intelligence and Humanized Computing(2021)
摘要
Intelligent fault diagnosis of bearings is an essential issue in the field of health management and the prediction of rotating machinery systems. The traditional bearing intelligent diagnosis algorithms based on the combination of feature extraction and classification for signal processing require high expert experience, which are time-consuming and lack universality. Compared with traditional methods, the convolutional neural network(CNN) can extract features automatically from the original vibration time-domain signal without any preprocessing. The accuracy of intelligent fault diagnosis can be improved by utilizing the multi-layer nonlinear mapping capability of deep convolutional neural networks. In order to realize the intelligent diagnosis and improve the recognition rate, this paper adopts the strategy of widening convolution kernels to obtain a larger receptive field and proposes a network design process pattern based on this idea, in addition, obtains the convolutional neural network with wide convolution kernels (WKCNN) model through experiments. Based on the time-domain vibration signal, this paper generates more input data through expansion and adopts the wide kernels of the first two convolutional layers to quickly extract features to improve efficiency. The smaller convolution kernels are used for multi-layer nonlinear mapping to deepen the network and improve detection accuracy. The results show that WKCNN performs well in accuracy, anti-noise, and timeliness compared with other diagnostic methods.
更多查看译文
关键词
Bearing intelligent fault diagnosis,Wide convolution kernels,Network design process pattern,WKCNN
AI 理解论文
溯源树
样例
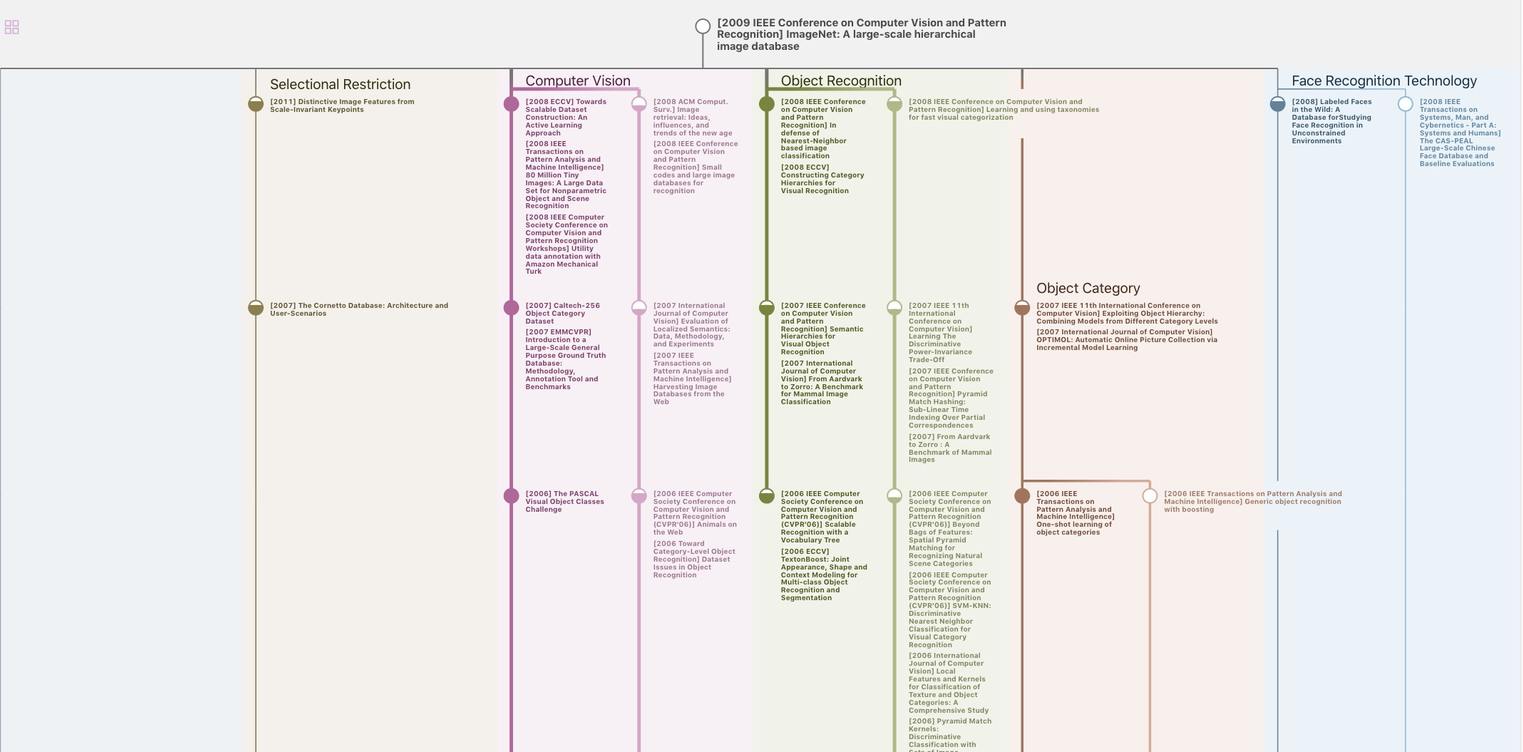
生成溯源树,研究论文发展脉络
Chat Paper
正在生成论文摘要