Language Model Using Differentiable Neural Computer Based On Forget Gate-Based Memory Deallocation
CMC-COMPUTERS MATERIALS & CONTINUA(2021)
摘要
A differentiable neural computer (DNC) is analogous to the Von Neumann machine with a neural network controller that interacts with an external memory through an attention mechanism. Such DNC?s offer a generalized method for task-specific deep learning models and have demonstrated reliability with reasoning problems. In this study, we apply a DNC to a language model (LM) task. The LM task is one of the reasoning problems, because it can predict the next word using the previous word sequence. However, memory deallocation is a problem in DNCs as some information unrelated to the input sequence is not allocated and remains in the external memory, which degrades performance. Therefore, we propose a forget gate based memory deallocation (FMD) method, which searches for the minimum value of elements in a forget gate-based retention vector. The forget gate based retention vector indicates the retention degree of information stored in each external memory address. In experiments, we applied our proposed NTM architecture to LM tasks as a task-specific example and to rescoring for speech recognition as a general-purpose example. For LM tasks, we evaluated DNC using the Penn Treebank and enwik8 LM tasks. Although it does not yield SOTA results in LM tasks, the FMD method exhibits relatively improved performance compared with DNC in terms of bits-per-character. For the speech recognition rescoring tasks, FMD again showed a relative improvement using the LibriSpeech data in terms of word error rate.
更多查看译文
关键词
Forget gate-based memory deallocation, differentiable neural computer, language model, forget gate-based retention vector
AI 理解论文
溯源树
样例
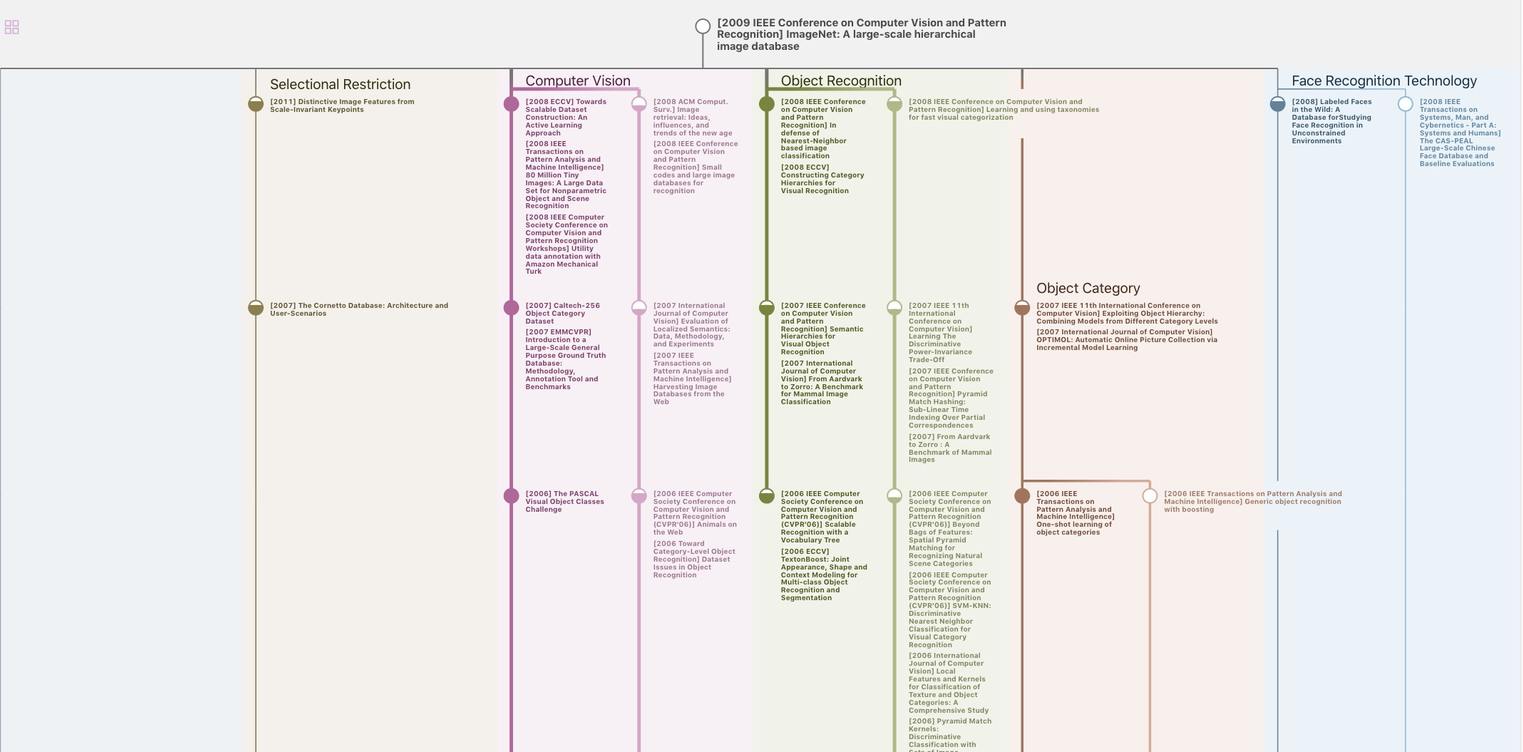
生成溯源树,研究论文发展脉络
Chat Paper
正在生成论文摘要