Desert Seismic Signal Denoising Based on Unsupervised Feature Learning and Time-Frequency Transform Technique
IEEE GEOSCIENCE AND REMOTE SENSING LETTERS(2022)
摘要
Noise reduction is an essential step in seismic exploration. Formally, the random broadband noise in the desert seismic is characterized as nonlinear, nonstationary, and non-Gaussian, and the energy is concentrated mainly in the low-frequency range. Moreover, the reflected signals generally cover the same spectral region as a strong random broadband noise. In this letter, a method combining unsupervised feature learning and the time-frequency transform (TFT) technique is proposed to reduce random broadband noise in desert seismic data. First, as a TFT technique, the variational mode decomposition (VMD) is carried out to decompose the multicomponent desert seismic signal into an ensemble of band-limited modes. Then, we apply an unsupervised feature learning method on each decomposed mode for detecting desert seismic events. Finally, the inverse VMD transform is conducted to obtain the final denoised result. This method is tested on both synthetic and field desert seismic data, demonstrating its preferable performance in reducing random broadband noise and preserving reflected signals.
更多查看译文
关键词
Feature extraction, Transforms, Broadband communication, Noise reduction, Time-frequency analysis, Thin film transistors, Signal to noise ratio, Denoising, desert seismic random broadband noise, feature learning, sparse filtering, variational mode decomposition (VMD)
AI 理解论文
溯源树
样例
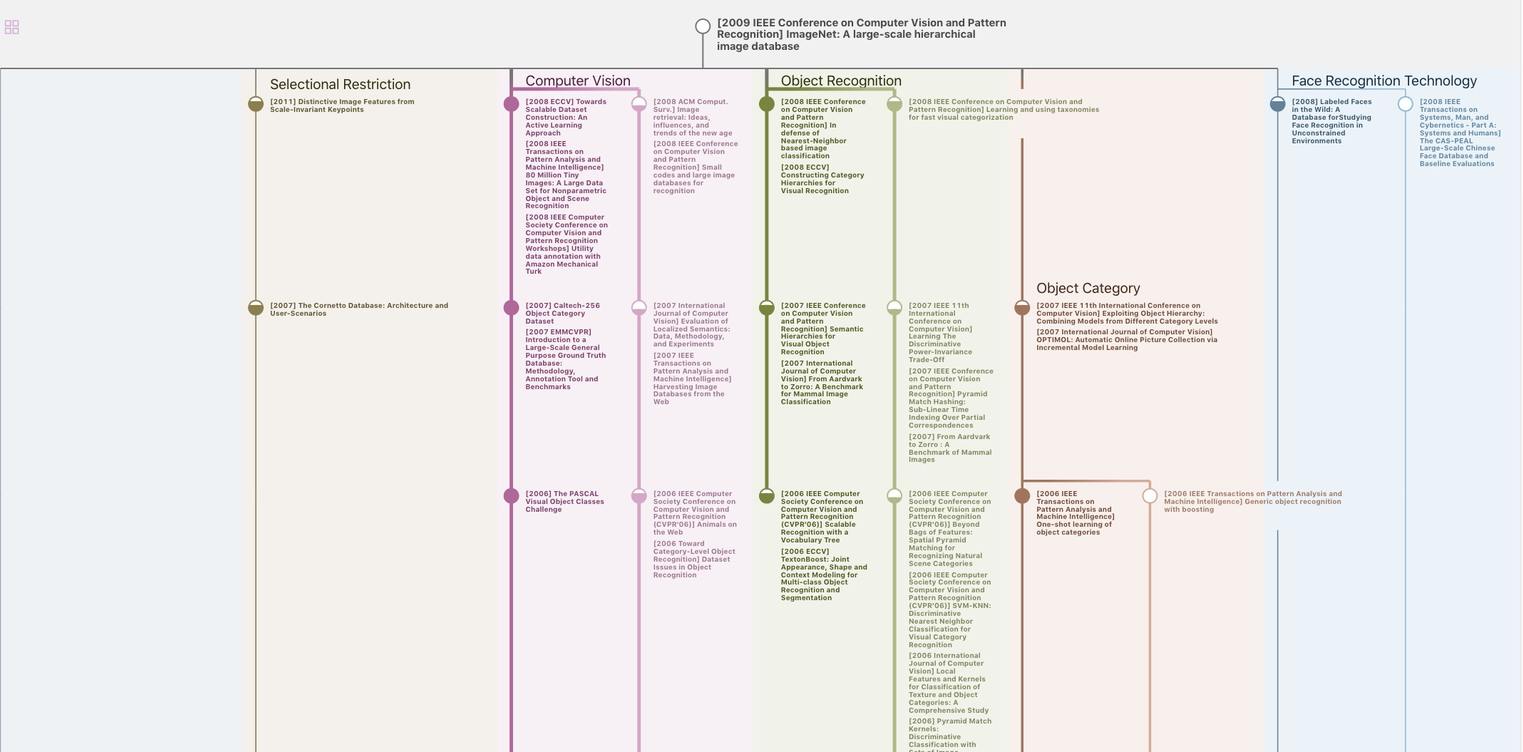
生成溯源树,研究论文发展脉络
Chat Paper
正在生成论文摘要